AI Doom | Google's STUNNING Videogame Generation Model BREAKS the Videogame Industry...
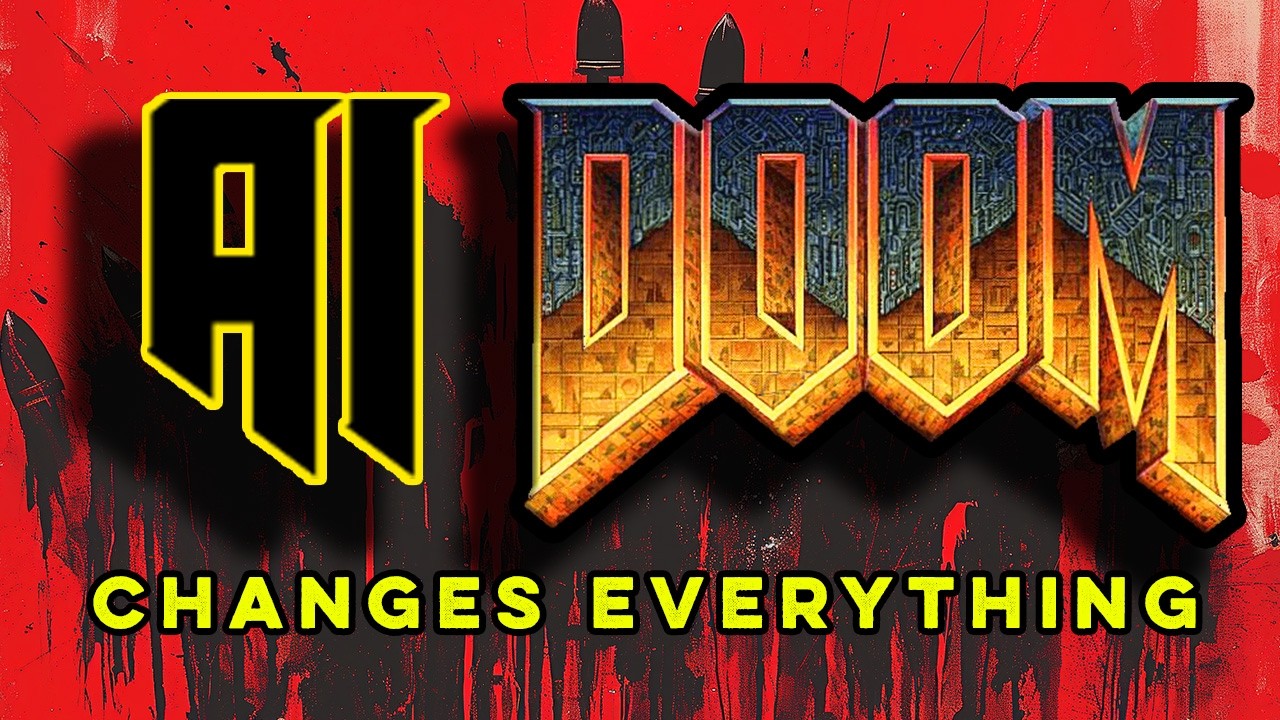
Key Takeaways at a Glance
00:00
AI-generated games in real-time are becoming a reality.00:40
Evolution from human-coded to AI-generated games.04:41
Diffusion models enable AI to create new content autonomously.07:43
AI agents learn game dynamics through reinforcement learning.11:30
Challenges in training AI agents for game generation.13:10
Human perception challenged by AI-generated game content.14:07
Random chance plays a significant role in outcomes.14:20
In-depth details and tactics are crucial for success.
1. AI-generated games in real-time are becoming a reality.
🥇92
00:00
Google's neural model can simulate games like Doom as players engage, revolutionizing game development and player experience.
- Real-time AI generation of games is advancing rapidly.
- Game engine neural models dream up game worlds and actions as players interact.
- This technology has the potential to reshape the gaming industry.
2. Evolution from human-coded to AI-generated games.
🥈88
00:40
Transitioning from human-coded games to AI-generated ones like Doom showcases a paradigm shift in game development.
- Historically, games were coded by humans, but AI now creates game content.
- AI models like diffusion models are used to generate game content autonomously.
- This shift marks a significant milestone in the gaming industry.
3. Diffusion models enable AI to create new content autonomously.
🥈89
04:41
Diffusion models like the one used by Google's game engine can generate new content without human intervention.
- Diffusion models learn from noisy images to create new, original content.
- These models operate by learning patterns from noisy data to produce coherent outputs.
- The use of diffusion models represents a novel approach to content creation.
4. AI agents learn game dynamics through reinforcement learning.
🥈87
07:43
AI agents in games like Doom learn game dynamics through reinforcement learning, mimicking human-like decision-making processes.
- Reinforcement learning involves positive and negative reinforcements to guide AI behavior.
- Creating effective reward functions is crucial to shaping AI behavior in games.
- AI agents can exhibit unexpected behaviors based on reinforcement learning outcomes.
5. Challenges in training AI agents for game generation.
🥈85
11:30
Training AI agents for game generation poses challenges due to the need for labeled data and complex reward functions.
- Collecting labeled data for AI training in games like Doom is resource-intensive.
- Designing accurate reward functions is essential but can lead to unintended consequences.
- Balancing positive and negative reinforcements is critical for effective AI training.
6. Human perception challenged by AI-generated game content.
🥇91
13:10
Human perception struggles to differentiate between AI-generated and real game content, showcasing the realism of AI creations.
- AI-generated game content reaches a level of realism that challenges human perception.
- Studies indicate that humans find it challenging to distinguish between AI-generated and real game footage.
- AI advancements in game generation blur the lines between virtual and real worlds.
7. Random chance plays a significant role in outcomes.
🥈85
14:07
Random chance remains a crucial factor, with outcomes often close to a 50-50 split, even with improved strategies.
- Improved strategies only marginally outperform blind selection.
- The study highlights the importance of acknowledging the role of chance in outcomes.
8. In-depth details and tactics are crucial for success.
🥈88
14:20
The success achieved is attributed to the meticulous attention to detail and strategic tactics outlined in the comprehensive paper.
- The paper contains valuable insights and strategies employed by a team of skilled individuals.
- Accessing the full paper provides a deeper understanding of the process and methodologies used.