AI Won't Be AGI, Until It Can At Least Do This
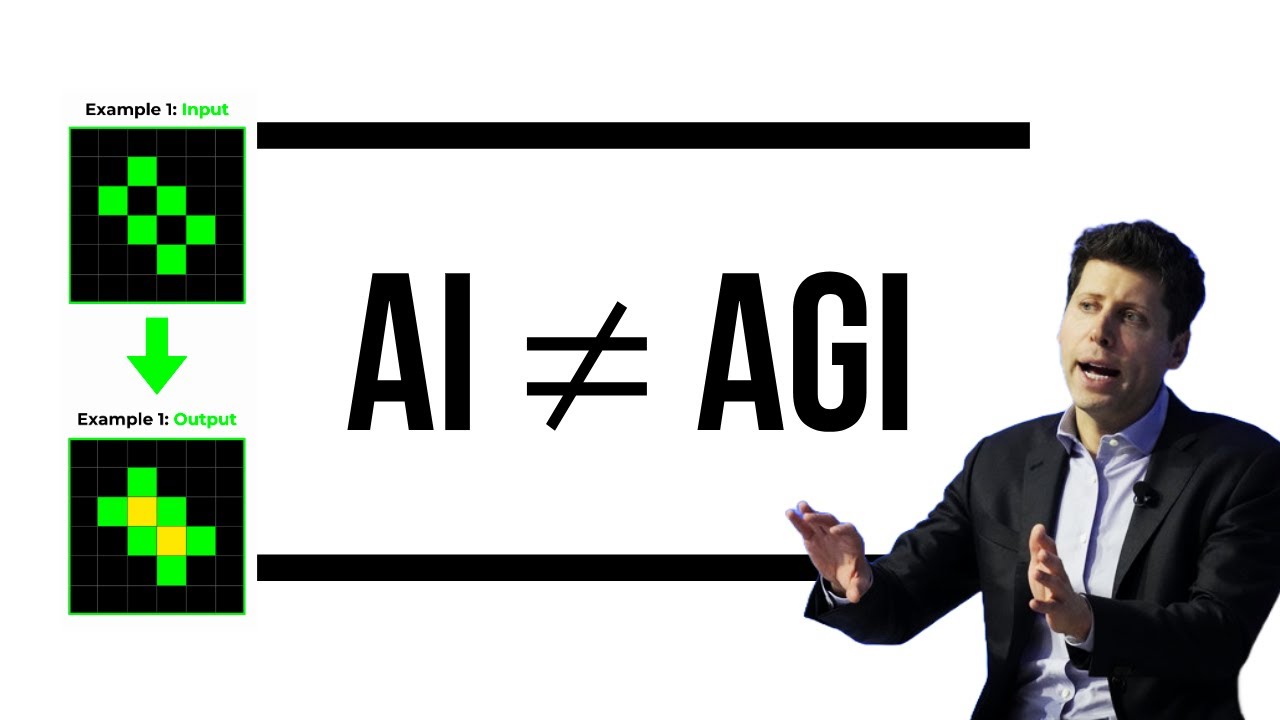
Key Takeaways at a Glance
00:00
Current AI models lack general intelligence.10:14
AI progress hinges on addressing reasoning gaps.11:59
AI faces challenges in meeting AGI expectations.12:12
AI development requires more than data scaling.13:51
AI's limitations stem from lack of adaptability to new tasks.15:29
Scaling up parameters and data won't lead to AGI.16:51
Compositionality in models can enhance reasoning abilities.19:00
Improving methods to locate reasoning programs within models is essential.22:54
Active inference can significantly boost model performance.27:21
Combining neural networks with symbolic systems can enhance planning tasks.29:21
Unlocking tacit knowledge can significantly advance AI.31:50
AI development requires a combination of approaches, not a single breakthrough.
1. Current AI models lack general intelligence.
🥇95
00:00
AI models like GPT-4 struggle with abstract reasoning challenges due to their inability to generalize beyond training data, highlighting the gap in achieving artificial general intelligence.
- Models fail when faced with new, unseen scenarios not present in their training data.
- AI lacks the ability to reason independently and adapt to novel situations.
- Generalization issues persist even with extensive training on similar examples.
2. AI progress hinges on addressing reasoning gaps.
🥈88
10:14
Closing the gap in AI's ability to reason independently and handle novel scenarios is crucial for advancing towards artificial general intelligence.
- Models need to evolve to handle tasks beyond what they have been explicitly trained on.
- Enhancing adaptability and independent reasoning are key milestones for AI advancement.
- Overcoming reasoning limitations is essential for AI to achieve higher levels of intelligence.
3. AI faces challenges in meeting AGI expectations.
🥇92
11:59
Scaling up models alone is insufficient to bridge the gap to artificial general intelligence, requiring novel approaches beyond data size increase.
- Merely increasing data volume does not enable models to generalize to unseen scenarios.
- Adapting on the fly to novel situations is a critical aspect AI needs to develop for AGI.
- Optimism exists that AI can evolve to handle novel tasks, but it demands more than data scaling.
4. AI development requires more than data scaling.
🥈85
12:12
Achieving artificial general intelligence demands innovative solutions beyond simply increasing the volume of training data for AI models.
- Scaling data alone does not address the fundamental challenges in AI's reasoning capabilities.
- Novel approaches are necessary to enhance AI's adaptability and problem-solving skills.
- AI evolution towards AGI necessitates advancements in reasoning and adaptability.
5. AI's limitations stem from lack of adaptability to new tasks.
🥈89
13:51
Models struggle with tasks not explicitly present in their training data, showcasing the challenge of adapting to unforeseen scenarios.
- Inability to generalize from known to unknown tasks hinders AI's adaptability.
- Human-like sample efficiency in learning remains a significant hurdle for AI models.
- Achieving adaptability to novel tasks requires more than just data scaling.
6. Scaling up parameters and data won't lead to AGI.
🥈88
15:29
Merely increasing parameters and data in current models is overly simplistic and won't solve the challenges towards achieving AGI.
- Scaling up parameters and data is not a comprehensive solution for achieving general intelligence.
- Challenges towards AGI require more than just adding parameters and data to existing models.
7. Compositionality in models can enhance reasoning abilities.
🥇92
16:51
Models that can better compose reasoning blocks into complex structures show promise in advancing towards general intelligence.
- Enhancing models' ability to compose reasoning blocks can lead to improved reasoning capabilities.
- Compositionality in models is crucial for advancing towards general intelligence.
8. Improving methods to locate reasoning programs within models is essential.
🥈89
19:00
Enhancing techniques to identify and locate reasoning programs within language models is crucial for advancing their mathematical reasoning abilities.
- Locating reasoning programs within models is key to enhancing their mathematical reasoning capabilities.
- Methods to improve the identification of reasoning programs within models are essential for their development.
9. Active inference can significantly boost model performance.
🥇94
22:54
Implementing active inference techniques during test time fine-tuning can greatly enhance model performance in solving complex tasks.
- Active inference methods during fine-tuning can lead to substantial performance improvements.
- Test time fine-tuning with active inference techniques can significantly boost model capabilities.
10. Combining neural networks with symbolic systems can enhance planning tasks.
🥈87
27:21
Integrating neural networks with traditional symbolic systems can improve planning tasks, leveraging the strengths of both approaches.
- Combining neural networks with symbolic systems can enhance the planning capabilities of models.
- Jointly training neural networks with symbolic systems can lead to improved performance in planning tasks.
11. Unlocking tacit knowledge can significantly advance AI.
🥇92
29:21
Training AI models on tacit data could lead to notable progress, but human experts need to document reasoning for further advancements.
- Much valuable knowledge is implicit and not explicitly written down.
- Documenting methodologies and failures is crucial for AI development.
- AI progress relies on human input to make implicit knowledge explicit.
12. AI development requires a combination of approaches, not a single breakthrough.
🥈88
31:50
Progress in AI involves merging different paradigms like deep planning and discrete search, rather than relying on a single revolutionary advancement.
- Combining various AI paradigms can lead to significant advancements.
- Winning in AI competitions may come from merging different approaches.
- Incremental progress through combined methods is key to achieving AGI.