Anon Leaks NEW Details About Q* | "This is AGI"
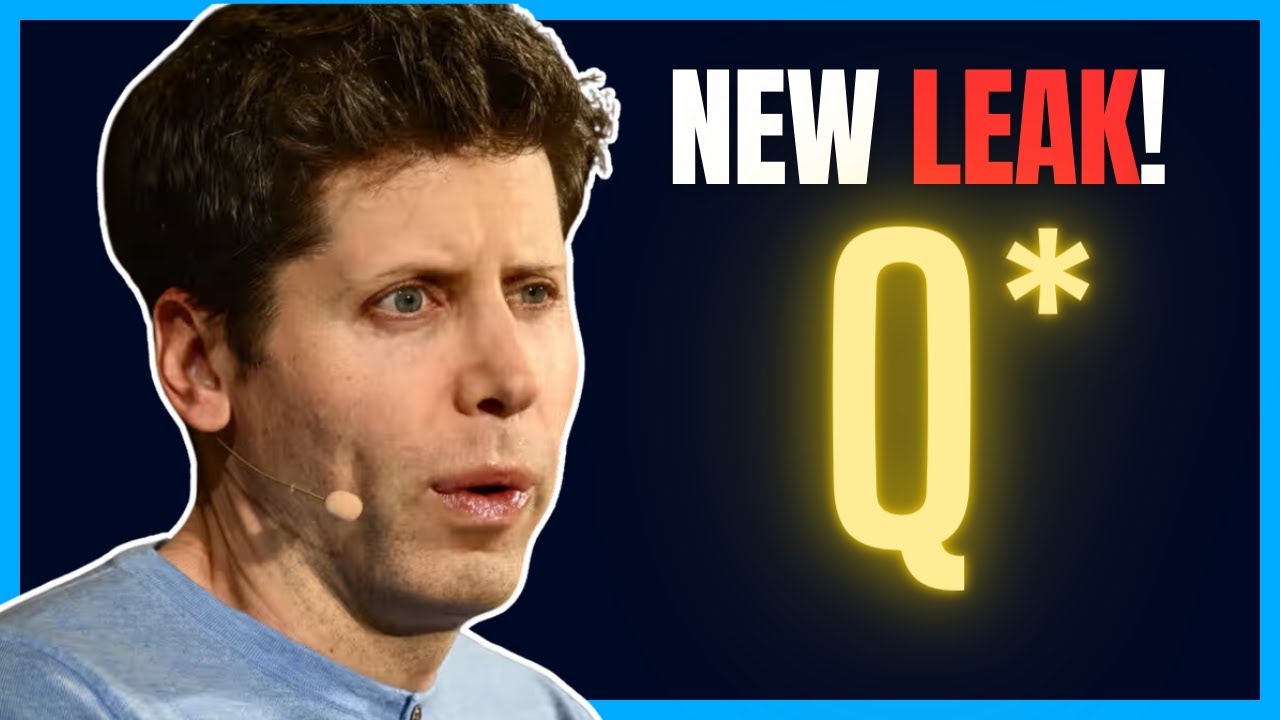
Key Takeaways at a Glance
09:40
QAR aims to enhance dialogue generation through energy-based models.12:25
QAR shifts focus from token prediction to understanding relevance and appropriateness of responses.14:12
QAR introduces abstract representation for optimization beyond text strings.14:22
QAR introduces a new method for generating dialogue responses.19:07
QAR and Quiet Star focus on teaching large language models to reason.21:17
Quiet Star enhances Chain of Thought accuracy.
1. QAR aims to enhance dialogue generation through energy-based models.
🥇92
09:40
QAR utilizes energy-based models to assess answer compatibility with prompts, shifting from token prediction to holistic evaluation for better responses.
- Energy-based models associate energy values with variable configurations.
- QAR mimics internal deliberation for complex problem-solving, akin to human thought processes.
- The model evaluates responses based on energy levels, favoring lower values for better compatibility.
2. QAR shifts focus from token prediction to understanding relevance and appropriateness of responses.
🥈88
12:25
QAR evaluates responses holistically, moving beyond sequential token prediction to assess the underlying relevance and appropriateness of answers to prompts.
- QAR's approach involves understanding the context and appropriateness of responses.
- The model aims to provide better answers by considering the overall relevance to prompts.
- This marks a significant departure from current large language model operations.
3. QAR introduces abstract representation for optimization beyond text strings.
🥈89
14:12
QAR's innovation lies in optimizing abstract representations instead of text strings, using computational minimization to define paths of least resistance.
- Optimization occurs in an abstract space, not limited to textual content.
- Abstract representations are refined iteratively to minimize energy in relation to prompts.
- Gradient descent is employed for computational minimization in abstract spaces.
4. QAR introduces a new method for generating dialogue responses.
🥇92
14:22
QAR leverages EBMs for dialogue generation, optimizing over an abstract representation space, introducing a more efficient method for generating dialogue responses.
- QAR's approach represents a departure from traditional language modeling techniques.
- Utilizing gradient-based inference, QAR offers a potentially more powerful method for generating dialogue responses.
- QAR sets a new benchmark for dialogue systems with its capacity for deep reasoning.
5. QAR and Quiet Star focus on teaching large language models to reason.
🥈89
19:07
QAR and Quiet Star techniques teach large language models how to think and reason through problems, emphasizing reasoning over mere response generation.
- Quiet Star introduces self-teaching via internal monologue for language models.
- These techniques aim to improve reasoning abilities by teaching models to reason generally.
- Reasoning is taught through diverse online text, helping models learn from useful thoughts.
6. Quiet Star enhances Chain of Thought accuracy.
🥈87
21:17
Combining Quiet Star with Chain of Thought improves zero-shot Chain of Thought accuracy on GSM 8K by over 7%, showcasing significant performance enhancement.
- The improvement in accuracy demonstrates the effectiveness of integrating Quiet Star with Chain of Thought.
- The open-sourcing of Quiet Star's code and weights allows for further exploration and experimentation.