Chinese Researchers Reveal How OpenAI o3 Works!
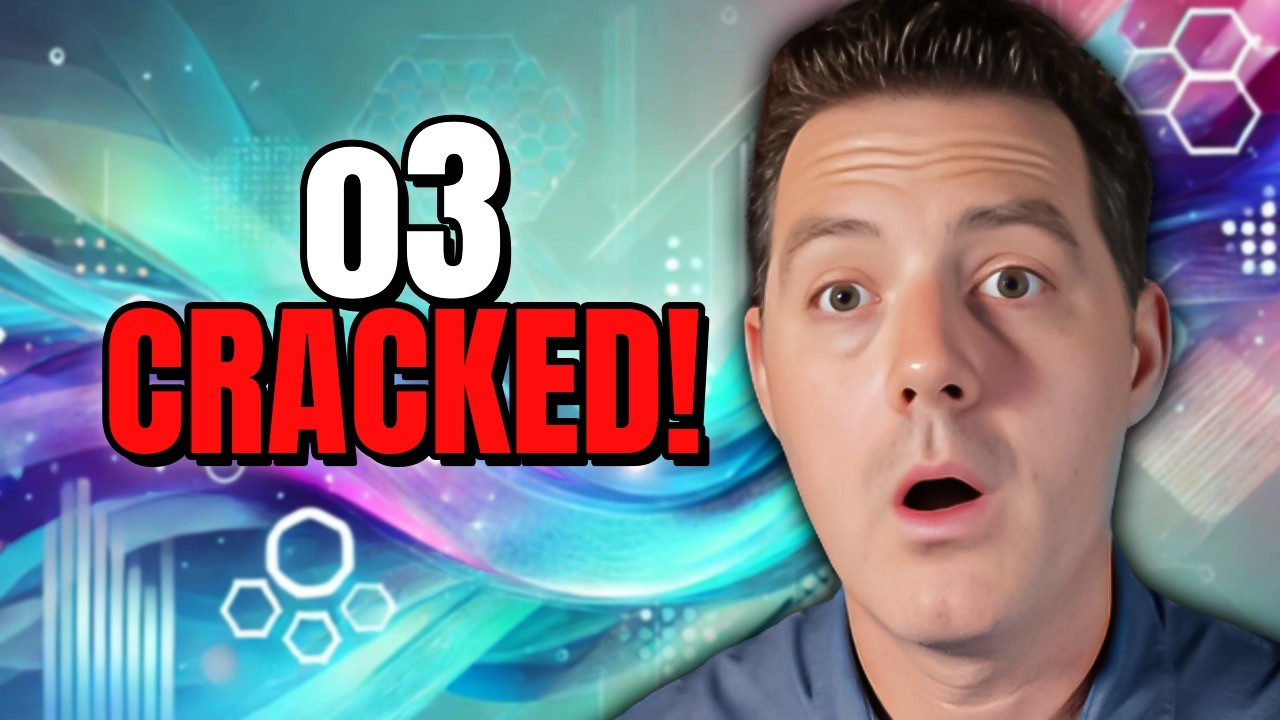
Key Takeaways at a Glance
00:00
Chinese researchers have uncovered the secrets of OpenAI's models.02:04
OpenAI's models are progressing towards AGI.03:56
Test time compute is essential for model performance.07:18
Four key aspects define the thinking models' functionality.11:09
Human-like reasoning behaviors enhance model capabilities.15:27
Exposure to programming code enhances AI reasoning capabilities.16:01
Reward design is crucial for AI learning.17:21
Realistic environments provide valuable feedback for AI.20:55
Search strategies enhance AI problem-solving.27:24
Reinforcement learning can achieve superhuman performance.29:39
Future directions for OpenAI's o3 include adapting to general domains.29:59
Introducing multiple modalities is a key focus for OpenAI.
1. Chinese researchers have uncovered the secrets of OpenAI's models.
🥇95
00:00
The research from Fudan University reveals how OpenAI's 01 and 03 models achieve advanced reasoning capabilities, classified as AGI.
- The study focuses on the concept of 'test time compute', which enhances model performance during inference.
- It identifies four critical elements that contribute to the models' thinking abilities.
- The findings aim to open-source the understanding of these advanced AI models.
2. OpenAI's models are progressing towards AGI.
🥇90
02:04
The 01 model represents a significant milestone, achieving reasoning capabilities comparable to PhD-level proficiency.
- It is part of OpenAI's roadmap towards artificial general intelligence, moving through defined stages.
- The model can perform human-like reasoning, including clarifying questions and exploring solutions.
- Current advancements suggest we may be nearing the third stage of AI development.
3. Test time compute is essential for model performance.
🥇92
03:56
The ability of models to think during inference time significantly boosts their performance on complex tasks like mathematics and scientific reasoning.
- More computation time during inference leads to better results.
- This approach marks a shift from traditional self-supervised learning to reinforcement learning.
- The 01 model exemplifies this new paradigm in AI development.
4. Four key aspects define the thinking models' functionality.
🥈88
07:18
The researchers identified policy initialization, reward design, search, and learning as critical components of the models.
- Policy initialization involves pre-training and fine-tuning to prepare the model for tasks.
- Reward design is crucial for guiding the model's learning process.
- Search capabilities during inference allow the model to explore multiple solutions.
5. Human-like reasoning behaviors enhance model capabilities.
🥈89
11:09
The models incorporate behaviors such as problem analysis, task decomposition, and self-correction to improve reasoning.
- These behaviors allow the model to break down complex problems into manageable tasks.
- Self-evaluation and correction enable the model to refine its responses iteratively.
- The ability to propose alternative solutions is crucial for overcoming reasoning obstacles.
6. Exposure to programming code enhances AI reasoning capabilities.
🥇92
15:27
Research shows that exposure to programming code significantly improves a model's logical reasoning skills, making it more effective in problem-solving.
- Structured logical data helps strengthen reasoning capabilities.
- Self-reflection allows models to assess and improve their outputs.
- Combining code exposure with self-reflection leads to better performance.
7. Reward design is crucial for AI learning.
🥈89
16:01
AI models utilize different reward systems, such as outcome rewards and process rewards, to learn from their outputs effectively.
- Outcome rewards assess the final output, while process rewards evaluate each step taken.
- Process rewards provide feedback on intermediate steps, allowing for targeted improvements.
- This dual approach enhances learning efficiency in complex problem-solving.
8. Realistic environments provide valuable feedback for AI.
🥈87
17:21
Interacting with realistic environments allows AI models to receive accurate feedback on their outputs, improving their learning process.
- Running generated scripts in compilers can validate AI outputs.
- In cases where real-time feedback isn't available, reward models simulate expected outcomes.
- This feedback loop is essential for refining AI performance.
9. Search strategies enhance AI problem-solving.
🥇90
20:55
AI models employ various search strategies to explore potential solutions and improve output quality during training and inference.
- Tree search techniques allow for broader exploration of solutions.
- Sequential revisions refine answers based on previous outputs.
- Effective search strategies can enable smaller models to outperform larger ones.
10. Reinforcement learning can achieve superhuman performance.
🥇95
27:24
Reinforcement learning allows AI to learn from trial and error, potentially surpassing human capabilities in specific tasks.
- AI models can discover new strategies through extensive self-play.
- The example of AlphaGo illustrates how AI can innovate beyond human understanding.
- Removing human feedback can lead to more efficient learning processes.
11. Future directions for OpenAI's o3 include adapting to general domains.
🥈88
29:39
Researchers are exploring how to adapt o3 to general domains like math and science, especially when answers are not clear.
- Adapting to clear domains is easier, but unknown problems pose challenges.
- The goal is to enable models to think through problems without known answers.
- This adaptation is crucial for expanding the model's applicability.
12. Introducing multiple modalities is a key focus for OpenAI.
🥈85
29:59
OpenAI is working on integrating multiple modalities into o3, enhancing its capabilities.
- Multiple modalities will allow the model to process different types of data.
- This integration is expected to improve the model's performance in various tasks.
- OpenAI has already discussed this direction in their research.