Hallucination-Free? Assessing the Reliability of Leading AI Legal Research Tools (Paper Explained)
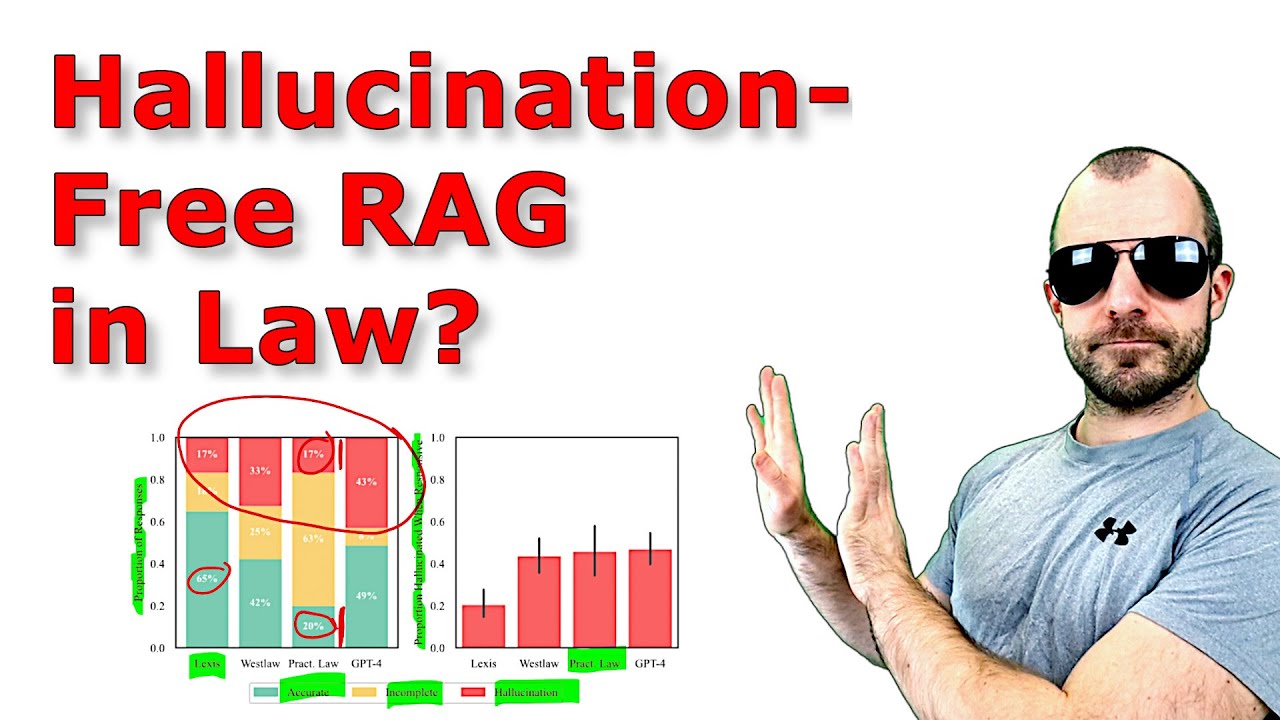
Key Takeaways at a Glance
01:57
Legal research tools integrate AI to enhance search capabilities.04:01
Hallucinations in AI legal research tools pose reliability challenges.15:45
Retrieval augmented generation (RAG) mitigates AI hallucinations.17:21
AI legal tools require nuanced understanding for effective application.18:55
Retrieval augmented generation enhances AI capabilities.28:16
Evaluation of AI tools must consider relevance of reference data.29:53
Hallucination rates impact AI tool reliability.31:59
Choosing the right product for evaluation is critical in AI research.37:00
Beware of misleading marketing claims by legal technology providers.39:01
Critical evaluation of AI tool claims is crucial for informed decision-making.45:27
Specialized education is essential for effective use of AI legal research tools.51:32
Challenges in legal research tools stem from varied expectations.52:01
Differentiating between natural language search and AI-generated outputs is crucial.54:15
Combining human reasoning with AI capabilities enhances system productivity.55:28
Evaluation metrics should distinguish between correctness and groundedness.1:09:20
AI legal research tools require human verification.1:10:11
Appropriate tool application is essential in legal research.
1. Legal research tools integrate AI to enhance search capabilities.
🥇92
01:57
AI integration in legal research tools aims to improve search efficiency by leveraging generative AI to assist in answering legal queries.
- Generative AI assists in collecting facts and references to provide answers.
- AI tools combine publicly available data with generative AI for legal research.
- AI aids in analyzing laws, case law, treaties, and commentaries for legal queries.
2. Hallucinations in AI legal research tools pose reliability challenges.
🥈89
04:01
AI legal tools, despite claims of being hallucination-free, still exhibit errors, impacting accuracy and reliability in legal contexts.
- Large language models may generate incorrect or misleading information, termed as hallucinations.
- The gap between linguistic likelihood and real-world truth leads to AI errors.
- AI systems struggle with unlikely truths and likely falsehoods, causing hallucinations.
3. Retrieval augmented generation (RAG) mitigates AI hallucinations.
🥇94
15:45
RAG enhances language model generation by incorporating retrieved data, reducing hallucination rates and improving answer accuracy.
- RAG combines language model output with relevant retrieved data for more accurate responses.
- The use of search engines to gather contextual information aids in reducing AI errors.
- RAG helps in addressing the limitations of AI models in legal research tasks.
4. AI legal tools require nuanced understanding for effective application.
🥈88
17:21
Utilizing AI in legal research demands a deep understanding of its limitations and the need for context-specific reasoning beyond statistical language predictions.
- Legal question answering necessitates explicit context construction and reasoning.
- AI systems must discern outdated, overruled, and relevant legal information for accurate responses.
- Effective legal AI implementation goes beyond statistical likelihood to include reasoning and context analysis.
5. Retrieval augmented generation enhances AI capabilities.
🥇96
18:55
Augmenting AI with retrieved documents improves performance by providing explicit references for generating answers, enhancing reasoning abilities.
- Explicitly providing references during runtime boosts AI performance.
- Retrieval augmented generation aids in complex reasoning tasks.
- Access to external information elevates AI capabilities beyond training data.
6. Evaluation of AI tools must consider relevance of reference data.
🥇92
28:16
The quality of AI systems heavily relies on the relevance of reference data provided, impacting the accuracy and reliability of generated outputs.
- Relevance of reference data significantly influences AI performance.
- Retrieval aspect is crucial for AI systems, determining the effectiveness of generated responses.
- Human expertise in curating reference data plays a vital role in AI tool evaluation.
7. Hallucination rates impact AI tool reliability.
🥈85
29:53
AI tools exhibiting hallucination rates affect the credibility and trustworthiness of generated information, highlighting potential inaccuracies.
- Hallucination rates indicate the frequency of incorrect or unsupported responses.
- High hallucination rates raise concerns about the reliability of AI-generated content.
- Inaccuracies due to hallucinations can undermine the utility of AI legal research tools.
8. Choosing the right product for evaluation is critical in AI research.
🥈89
31:59
Selecting the appropriate tool for assessment is essential to ensure accurate evaluations and avoid misleading conclusions.
- Picking the correct product for evaluation is crucial for valid research outcomes.
- Misjudging the tool for evaluation can lead to flawed assessments and misinterpretations.
- Access to the right AI tool is fundamental for unbiased and reliable research findings.
9. Beware of misleading marketing claims by legal technology providers.
🥇92
37:00
Legal tech companies may exaggerate their AI capabilities, leading to potential misinterpretation of their products' reliability.
- Claims of 100% hallucination-free citations may not reflect the overall accuracy of AI outputs.
- Understanding the nuances in marketing statements can prevent misjudgments of AI tools' capabilities.
- Academics scrutinize these claims to ensure transparency and accuracy in AI tool functionalities.
10. Critical evaluation of AI tool claims is crucial for informed decision-making.
🥈87
39:01
Scrutinizing marketing messages and understanding the limitations of AI tools can prevent misinterpretation and reliance on potentially misleading information.
- Distinguishing between marketing claims and actual AI capabilities is vital for users to make informed choices.
- Awareness of the complexities and challenges in AI-generated outputs enhances users' ability to assess tool reliability.
- Balancing the promises of AI tools with realistic expectations ensures effective utilization in legal research tasks.
11. Specialized education is essential for effective use of AI legal research tools.
🥈89
45:27
Utilizing retrieval augmented generation in legal tasks requires a deep understanding of legal contexts and specialized knowledge.
- Legal queries often lack clear-cut answers due to the complexity of case law and contextual dependencies.
- Deciding document relevance in legal settings demands expertise to avoid misleading or irrelevant information.
- Human involvement in selecting and interpreting references enhances the accuracy of AI-generated legal responses.
12. Challenges in legal research tools stem from varied expectations.
🥈88
51:32
Expectations for AI legal research tools often exceed current capabilities, leading to challenges in retrieval and generation accuracy.
- Current tools struggle with retrieval and generation accuracy due to outsized expectations.
- Legal professionals face difficulties due to the mismatch between tool capabilities and user expectations.
- Issues arise from lumping different functionalities together, causing confusion in tool performance.
13. Differentiating between natural language search and AI-generated outputs is crucial.
🥈85
52:01
Understanding the distinction between natural language search and AI-generated outputs is essential for evaluating legal research tool performance.
- Natural language search involves querying in plain language without strict keyword requirements.
- AI-generated outputs go beyond search results, potentially including responses produced by AI models like LLMs.
- Clarity on the differences helps in setting realistic expectations for AI tools.
14. Combining human reasoning with AI capabilities enhances system productivity.
🥇92
54:15
Integrating human reasoning with AI technology can lead to more productive systems by leveraging AI's speed in processing vast information and human expertise in contextual relevance.
- A hybrid approach combining AI's rapid data processing with human judgment enhances system effectiveness.
- Utilizing AI for quick data analysis and human input for contextual understanding creates a balanced and efficient system.
- Collaboration between technology and human expertise optimizes productivity and accuracy in legal research.
15. Evaluation metrics should distinguish between correctness and groundedness.
🥇94
55:28
Assessing AI legal research tools should involve distinct metrics for correctness (factual accuracy) and groundedness (valid references to legal documents) to identify and address hallucinations effectively.
- Correctness focuses on factual accuracy and relevance to the query, while groundedness emphasizes valid references to legal sources.
- Identifying hallucinations requires differentiating between incorrect, ungrounded, and incomplete responses.
- Clear metrics on correctness and groundedness are essential for improving AI tool performance.
16. AI legal research tools require human verification.
🥇96
1:09:20
Users must verify key propositions supported by citations as AI tools have not eliminated errors.
- Errors in AI tools often stem from poor retrieval and lack of legal reasoning.
- Collaboration between humans and machines yields better results than relying solely on AI.
- Understanding the limitations of AI tools is crucial for accurate legal research.
17. Appropriate tool application is essential in legal research.
🥇92
1:10:11
Selecting the correct tool for the specific problem and understanding its capabilities are vital for effective legal research.
- Knowing the strengths and weaknesses of AI tools is crucial for optimal usage.
- Users need to interpret and apply AI-generated outputs correctly for reliable results.
- Misuse of AI tools due to lack of understanding can lead to erroneous conclusions.