How to give AI "Memory" - Intro to RAG (Retrieval Augmented Generation)
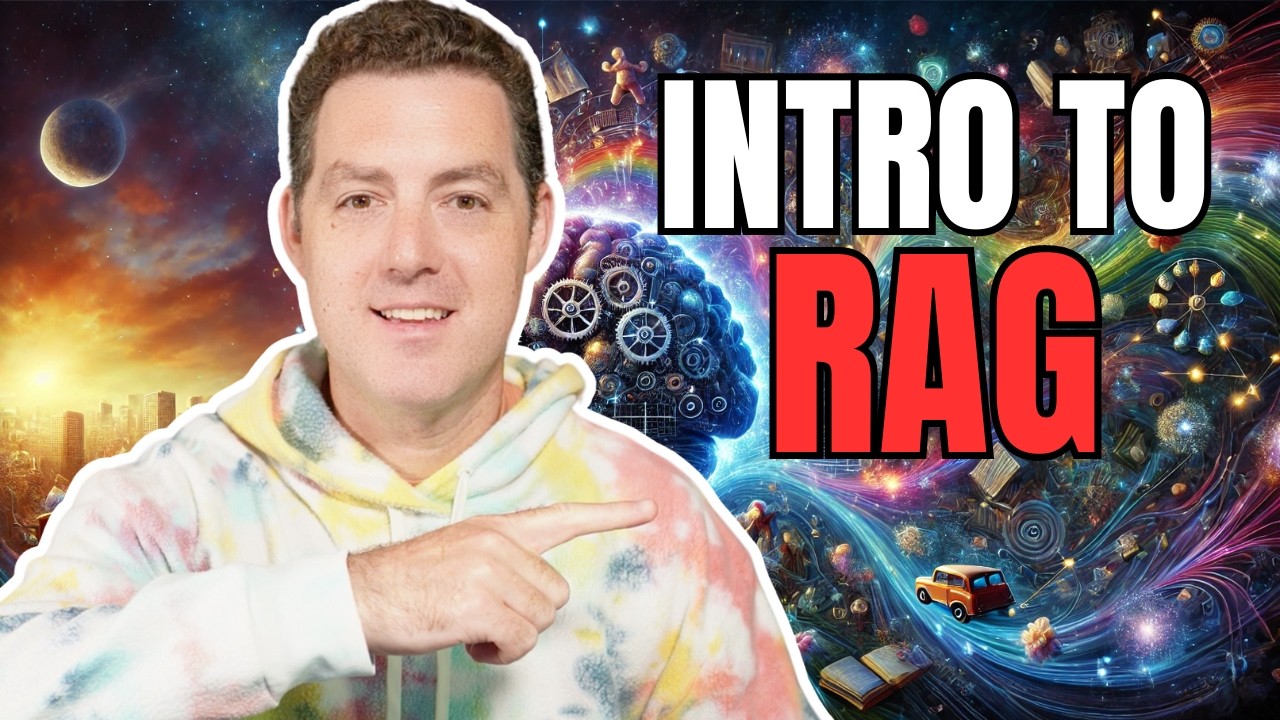
Key Takeaways at a Glance
00:00
RAG enhances large language models with external knowledge.02:12
RAG overcomes limitations of context windows in large language models.03:29
RAG facilitates personalized interactions and continuous learning.07:44
RAG optimizes information retrieval for large language models.10:40
RAG empowers agents to provide comprehensive and accurate responses.
1. RAG enhances large language models with external knowledge.
🥇95
00:00
Retrieval Augmented Generation (RAG) provides large language models with additional information, acting as a memory extension beyond their initial training.
- RAG allows large language models to access external sources for prompt augmentation.
- It enables models to query external databases for relevant information to enhance responses.
- RAG serves as a method to continuously update models with new knowledge efficiently.
2. RAG overcomes limitations of context windows in large language models.
🥇92
02:12
Context windows in models like GPT have limitations in storing and accessing vast amounts of information, making RAG a more efficient solution.
- Context windows restrict the amount of information models can process in prompts and responses.
- RAG allows for the storage and retrieval of extensive knowledge without overwhelming the context window.
- Using RAG prevents prompt limitations and ensures relevant information is accessible.
3. RAG facilitates personalized interactions and continuous learning.
🥈89
03:29
By enabling models to retain conversation history and access specific knowledge sources, RAG supports personalized interactions and ongoing learning.
- Models can store past interactions for improved user understanding and tailored responses.
- Access to external databases empowers models to adapt and learn from new information over time.
- RAG enhances the ability of models to provide accurate and relevant responses.
4. RAG optimizes information retrieval for large language models.
🥈88
07:44
Utilizing RAG allows for efficient retrieval of relevant data from external sources, enhancing the accuracy and depth of model responses.
- RAG streamlines the process of accessing external knowledge for models.
- It ensures that models can incorporate up-to-date information into their responses.
- By leveraging RAG, models can provide more precise and informed answers.
5. RAG empowers agents to provide comprehensive and accurate responses.
🥈87
10:40
Agents leveraging RAG can iteratively gather information, incorporate external knowledge, and deliver enhanced responses to complex queries.
- Agents can utilize RAG to access diverse data sources and refine their responses.
- RAG enables agents to offer detailed and well-researched answers to user queries.
- The use of RAG enhances the capabilities of agents in providing valuable insights.