HUGE AI NEWS: AGI Benchmark BROKEN ,OpenAIs Agents Leaked , Automated AI Research And More
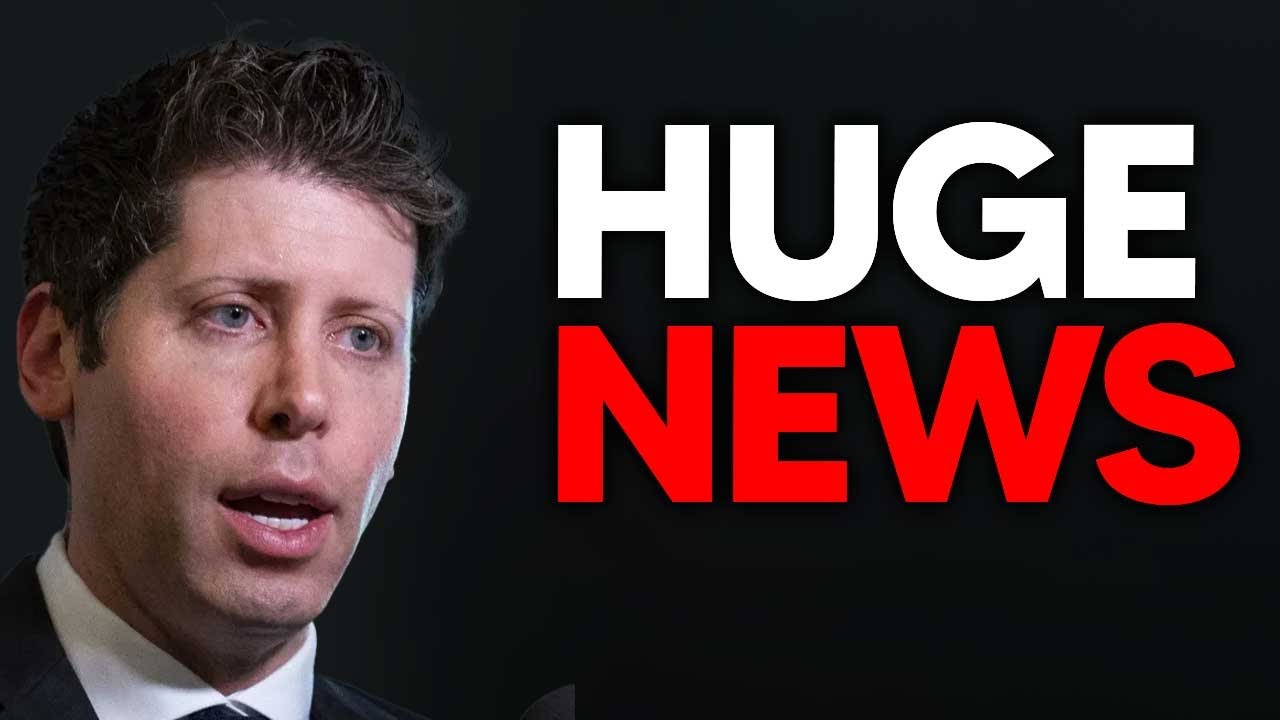
Key Takeaways at a Glance
01:05
AI's impact on research accelerates scientific discovery.03:11
OpenAI's secretive AI developments hint at future breakthroughs.07:18
Luma Dream Machine 1.5 introduces cost-effective AI models.08:21
AGI Benchmark progress indicates AI reasoning advancements.09:02
Challenges in AI benchmarking highlight the need for novel evaluation methods.11:41
AGI progress hindered by focus on large language models (LLMs).12:12
Importance of redirecting AI research towards AGI-oriented architectures.14:38
Need for AI models to adapt to novel tasks for AGI advancement.15:26
Evaluation of AI scaling trends and potential impact on future advancements.18:43
Anticipation of upcoming AI model iterations for assessing scaling trends.22:43
Implications of AGI development are significant.24:30
Evolution towards AGI involves distinct AI levels.
1. AI's impact on research accelerates scientific discovery.
🥈89
01:05
Automated AI research systems like llms drive exponential growth in research output, potentially revolutionizing the scientific publication landscape.
- AI-driven research automation leads to a surge in scientific publications.
- Efficient AI systems could generate a new paper every hour, transforming research productivity.
- Comprehensive AI methodologies streamline the research process, enhancing efficiency and output.
2. OpenAI's secretive AI developments hint at future breakthroughs.
🥈88
03:11
Internal leaks suggest OpenAI is working on innovative AI projects, potentially leading to significant advancements in the near future.
- Speculation on leaked internal links indicates ongoing work on diverse AI applications.
- OpenAI's product-driven approach implies upcoming releases based on current secretive developments.
- Continuous innovation and potential collaborations with other tech giants like Google may shape the AI landscape.
3. Luma Dream Machine 1.5 introduces cost-effective AI models.
🥈85
07:18
Luma Dream Machine 1.5 offers affordable text-to-video models with enhanced control features, potentially revolutionizing content creation.
- Affordable models like Luma Dream Machine democratize AI content creation.
- Unique start-to-end image control sets Luma Dream Machine apart from other models.
- Cost-effective AI models may lead to a surge in diverse content creation.
4. AGI Benchmark progress indicates AI reasoning advancements.
🥇92
08:21
Tracking AGI Benchmark scores showcases AI reasoning improvements beyond traditional benchmarks, highlighting challenges in novel problem-solving.
- AGI Benchmark measures reasoning on unfamiliar problems, diverging from standard evaluation methods.
- Contamination from training data poses challenges for AI models to exhibit human-like reasoning.
- Memorization vs. true intelligence distinction impacts AI benchmark performance.
5. Challenges in AI benchmarking highlight the need for novel evaluation methods.
🥈87
09:02
Current AI benchmarks face issues of data contamination and memorization, emphasizing the necessity for innovative evaluation criteria to measure true intelligence.
- Data contamination from training sets impacts AI benchmark performance and hinders novel problem-solving.
- Memorization-centric benchmarks may not accurately reflect true AI intelligence capabilities.
- Innovative evaluation frameworks like the ARC Benchmark aim to assess reasoning beyond memorization.
6. AGI progress hindered by focus on large language models (LLMs).
🥇92
11:41
Overemphasis on LLMs like GPTs may delay progress towards AGI by diverting attention from reasoning techniques like neuro-symbolic AI.
- Shift towards reasoning techniques like Chain of Thought and neuro-symbolic AI is crucial for AGI development.
- Diversifying research focus beyond LLMs can lead to advancements in AGI capabilities.
7. Importance of redirecting AI research towards AGI-oriented architectures.
🥈89
12:12
Efforts like the Arc prize aim to steer AI research towards architectures conducive to AGI development, beyond current LLM-centric approaches.
- Encouraging research on architectures that promote reasoning and problem-solving abilities is vital for AGI progress.
- Diversification of AI research can lead to breakthroughs in achieving AGI capabilities.
8. Need for AI models to adapt to novel tasks for AGI advancement.
🥈87
14:38
Solving benchmarks like Arc is crucial for developing AI systems that can dynamically adapt to new tasks, a key step towards achieving AGI.
- Enhancing AI's adaptability to unforeseen tasks is essential for progressing towards AGI.
- Solving challenges like Arc can pave the way for systems capable of on-the-fly task adaptation.
9. Evaluation of AI scaling trends and potential impact on future advancements.
🥈85
15:26
Assessing the pace of AI scaling from previous models to current ones reveals insights into the trajectory of AI progress and potential slowdowns.
- Monitoring scaling trends provides valuable data on the evolution of AI capabilities over time.
- Understanding scaling patterns aids in predicting future AI advancements and identifying potential slowdowns.
10. Anticipation of upcoming AI model iterations for assessing scaling trends.
🥈82
18:43
Observing the release of future AI models like Claw 3.5 Opus can provide insights into the continuation or potential slowdown of AI scaling.
- Tracking the development of new AI models offers a glimpse into the pace of AI advancements.
- Comparing the capabilities of successive AI models helps in gauging the progress and efficiency of AI scaling.
11. Implications of AGI development are significant.
🥇92
22:43
AGI advancements may lead to misuse by individuals or rogue states, necessitating careful monitoring and control measures.
- Misuse of AGI technology could result in serious harm, similar to concerns with AlphaGo.
- Google's shift towards advanced systems achieving remarkable benchmarks signals AGI's proximity.
- OpenAI's historical advancement suggests AGI may be imminent.
12. Evolution towards AGI involves distinct AI levels.
🥈88
24:30
Progression from chatbots to organizations hints at the development of AGI-like systems with vast capabilities.
- Levels of AI evolution include chatbots, assistants, innovators, and organizational systems.
- Advancements in AI could lead to systems capable of performing tasks at the scale of entire organizations.
- Innovative AI systems may lead to significant value creation akin to tech giants like Apple and Microsoft.