LangChain CEO Talks About the FUTURE of AI AGENTS
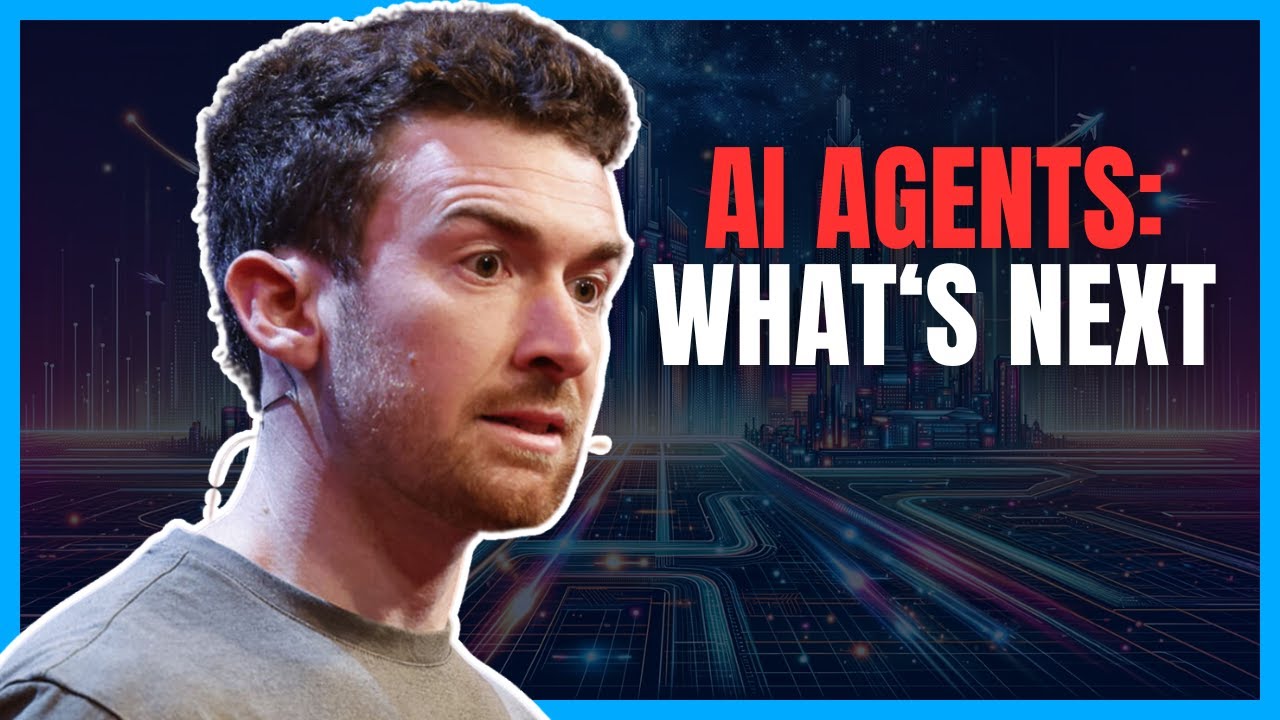
Key Takeaways at a Glance
01:47
Agents are more than just prompts; they possess additional superpowers.08:40
Agent Frameworks play a crucial role in enhancing flow engineering.09:53
Human in the loop remains essential for ensuring reliability in agent applications.11:14
Enhancing user experience (UX) in agent applications is a key focus area.13:26
Memory capabilities of agents enable interactive learning and correction.13:47
Memory in AI agents involves procedural and personalized aspects.14:39
Short-term and long-term memory are crucial for AI agents' development.15:25
Challenges in AI memory management require evolving strategies.
1. Agents are more than just prompts; they possess additional superpowers.
π₯92
01:47
Agents go beyond being mere prompts by having tools, memory, planning abilities, and the capacity to perform actions, enhancing their capabilities significantly.
- Agents can access various tools like calendars, calculators, and code interpreters.
- Memory features include short-term memory within conversations and long-term memory like retrieval augmented generation.
- Agents can plan, reflect, decompose subgoals, and execute actions, elevating their functionality.
2. Agent Frameworks play a crucial role in enhancing flow engineering.
π₯88
08:40
Agent Frameworks aid in optimizing flow engineering, allowing for better planning and coordination among different models, offering a valuable tool for developers.
- Flow engineering involves designing graphs or state machines to improve performance.
- Agent Frameworks assist in prompt engineering and now focus on enhancing flow engineering for improved efficiency.
- Optimizing flow engineering remains a significant area for exploration and development.
3. Human in the loop remains essential for ensuring reliability in agent applications.
π₯87
09:53
Despite advancements, human oversight is crucial to prevent errors and hallucinations, especially in large enterprise settings, striking a balance between automation and human intervention.
- Agent Frameworks help reduce errors through caching, prompt libraries, and human intervention.
- Balancing human involvement is critical to maintaining reliability and quality in agent applications.
- Optimizing the human in the loop strategy is an ongoing experiment to ensure optimal performance.
4. Enhancing user experience (UX) in agent applications is a key focus area.
π₯89
11:14
Improving UX through features like rewind and edit capabilities offers users more control and reliability, contributing to a better interaction with agent applications.
- Rewind and edit functionalities empower users to correct mistakes and make informed decisions.
- Innovative UX elements like rewind and edit enhance reliability and steering ability in agent applications.
- Exploring UX enhancements like rewind and edit can lead to more reliable and user-friendly agent interactions.
5. Memory capabilities of agents enable interactive learning and correction.
π₯86
13:26
Agents can learn interactively by receiving corrections and guidance from users, showcasing the potential for adaptive learning and improvement.
- Interactive teaching allows users to guide agents in tasks like writing tweets in specific styles.
- Memory features enable agents to adapt and improve based on user corrections and feedback.
- Agents like Pythagora demonstrate effective memory capabilities for learning and correction.
6. Memory in AI agents involves procedural and personalized aspects.
π₯92
13:47
AI agents can remember procedures and personalized facts, enhancing user experiences and interactions.
- Procedural memory helps AI remember correct ways to perform tasks.
- Personalized memory enhances user experiences by recalling specific personal details.
7. Short-term and long-term memory are crucial for AI agents' development.
π₯89
14:39
Short-term memory enables real-time learning and improvement, while long-term memory aids in personalization and business knowledge retention.
- Short-term memory facilitates immediate learning and adaptation.
- Long-term memory stores company knowledge for future use and evolution.
8. Challenges in AI memory management require evolving strategies.
π₯85
15:25
Addressing memory storage, retention rules, and adaptation to changing business needs are key challenges in AI memory management.
- Determining what to store and when to forget poses challenges.
- Adapting memory to evolving business requirements is crucial for AI effectiveness.