Llama 405b: Full 92 page Analysis, and Uncontaminated SIMPLE Benchmark Results
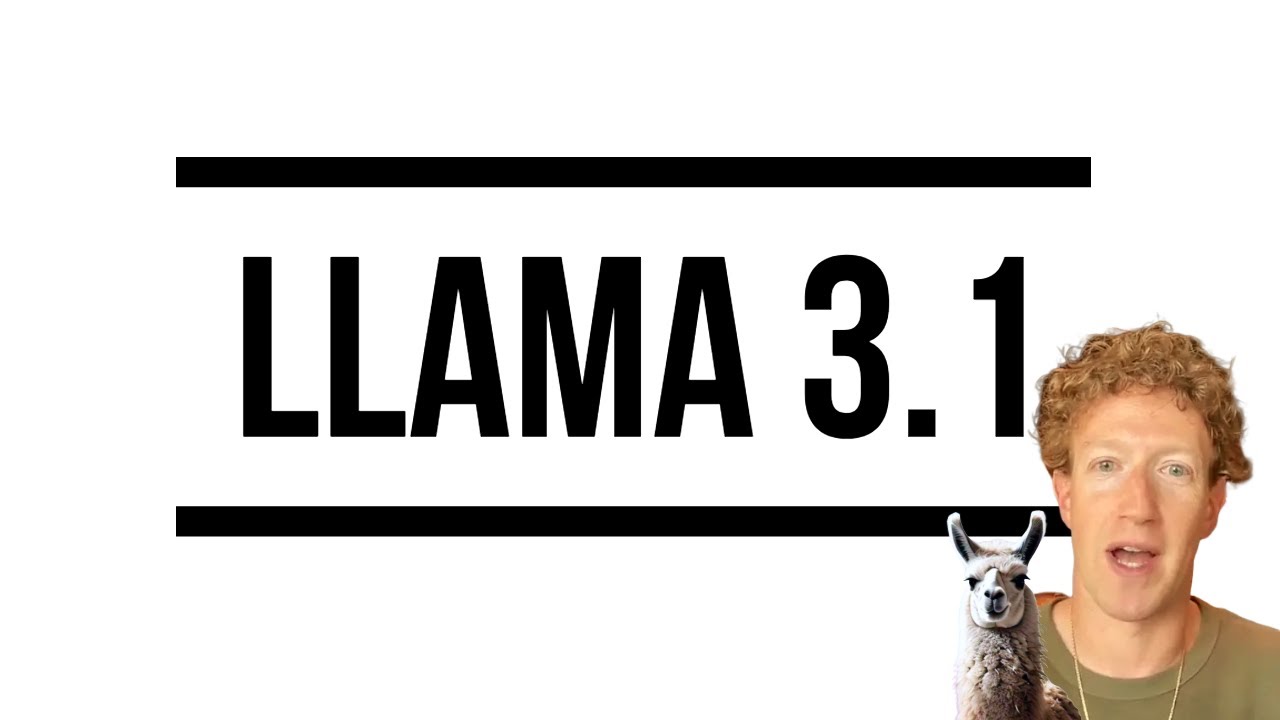
Key Takeaways at a Glance
00:00
Llama 3 1 405 billion parameter model delivers comparable quality to leading language models.02:47
Meta's commitment to open-source AI raises questions about data transparency and sourcing.08:01
Llama 3 1 405b model's training data cleaning process emphasizes quality annotations and filtering.10:06
Llama 3 1 405b model showcases advancements in reasoning and math skills training.11:35
Private Simple Benchmark reveals significant performance gaps between AI models and human reasoning.16:06
Contamination in traditional benchmarks is a significant issue.17:35
Llama 405b excels in long-context question answering.19:40
Llama 405b demonstrates safety improvements.25:40
Meta emphasizes responsible development of AGI.
1. Llama 3 1 405 billion parameter model delivers comparable quality to leading language models.
🥇92
00:00
Meta's Llama 3 1 405b model matches or surpasses GPT 4 in quality, showcasing significant advancements in AI language models.
- Meta's innovations include higher quality data and increased compute power for superior performance.
- The model's scale of operations, exceeding 10^25 floating point operations, demonstrates its computational prowess.
2. Meta's commitment to open-source AI raises questions about data transparency and sourcing.
🥈88
02:47
The definition of open-source AI remains ambiguous due to undisclosed training data sources, raising concerns about data integrity and reproducibility.
- Meta's reliance on various data sources makes replicating models challenging.
- Issues with data accessibility and transparency highlight potential ethical and legal implications.
3. Llama 3 1 405b model's training data cleaning process emphasizes quality annotations and filtering.
🥈87
08:01
Meta's meticulous data cleaning approach focuses on removing tonal issues, emojis, and ensuring high-quality human annotations for model training.
- The model's training involved expert models to enhance data quality and filter out undesirable elements.
- Synthetic data generation using the Frontier Model aids in model training and improvement.
4. Llama 3 1 405b model showcases advancements in reasoning and math skills training.
🥈89
10:06
The model's training methodology includes teaching reasoning steps, filtering incorrect reasoning traces, and enhancing mathematical skills through human prompts.
- Meta's approach involves training models to recognize and learn from reasoning chains.
- Utilization of Monte Carlo research for valid reasoning traces demonstrates a sophisticated training strategy.
5. Private Simple Benchmark reveals significant performance gaps between AI models and human reasoning.
🥇93
11:35
The Simple Benchmark, rigorously vetted and private, exposes substantial performance disparities between AI models and human reasoning abilities.
- Models like Claude 3 5 Sonic outperform others, but still fall short compared to human performance.
- Challenges like spatial intelligence questions highlight limitations in AI's ability to simulate real-world scenarios.
6. Contamination in traditional benchmarks is a significant issue.
🥇92
16:06
Contamination through word matching or engram checks is prevalent in traditional benchmarks, leading to underestimation of the problem.
- Exclusion of benchmarks with too few examples or extreme erratic behavior when data was cleaned.
- Private benchmarks like those from Scale AI are expected to become more common.
- Human comparisons and leaderboards may pose challenges in benchmark evaluations.
7. Llama 405b excels in long-context question answering.
🥈88
17:35
Llama 405b's strength lies in answering questions that require scouring through a long context of 128k tokens, outperforming other models in such scenarios.
- Comparison with GPT-4, GPT-40, and Claude 3.5 Sonic showed significant superiority.
- The model's performance in infinite benching QA tasks and handling multiple needles in a haystack was highlighted.
8. Llama 405b demonstrates safety improvements.
🥈87
19:40
Llama 405b shows a lower violation rate compared to competitors, balancing safety with a low false refusal rate, critical for model usefulness.
- Acknowledgment of prompt injection susceptibility compared to other models.
- Meta's rigorous pre-checks and safety considerations are commendable.
- Awareness of false refusals and the importance of balancing safety with model utility.
9. Meta emphasizes responsible development of AGI.
🥈89
25:40
Meta's release of Llama 405b aims to encourage the industry to embrace open and responsible development of Artificial General Intelligence.
- Incentivized separate team for preventing contamination in pre-training data.
- Continuous improvements in Foundation models are expected, exploring complex architectures and training recipes.
- Comparison anticipation with future models like Gemini 2 and GPT-5.