Meta's LLAMA 3 SHOCKS the Industry | OpenAI Killer? Better than GPT-4, Claude 3 and Gemini Pro
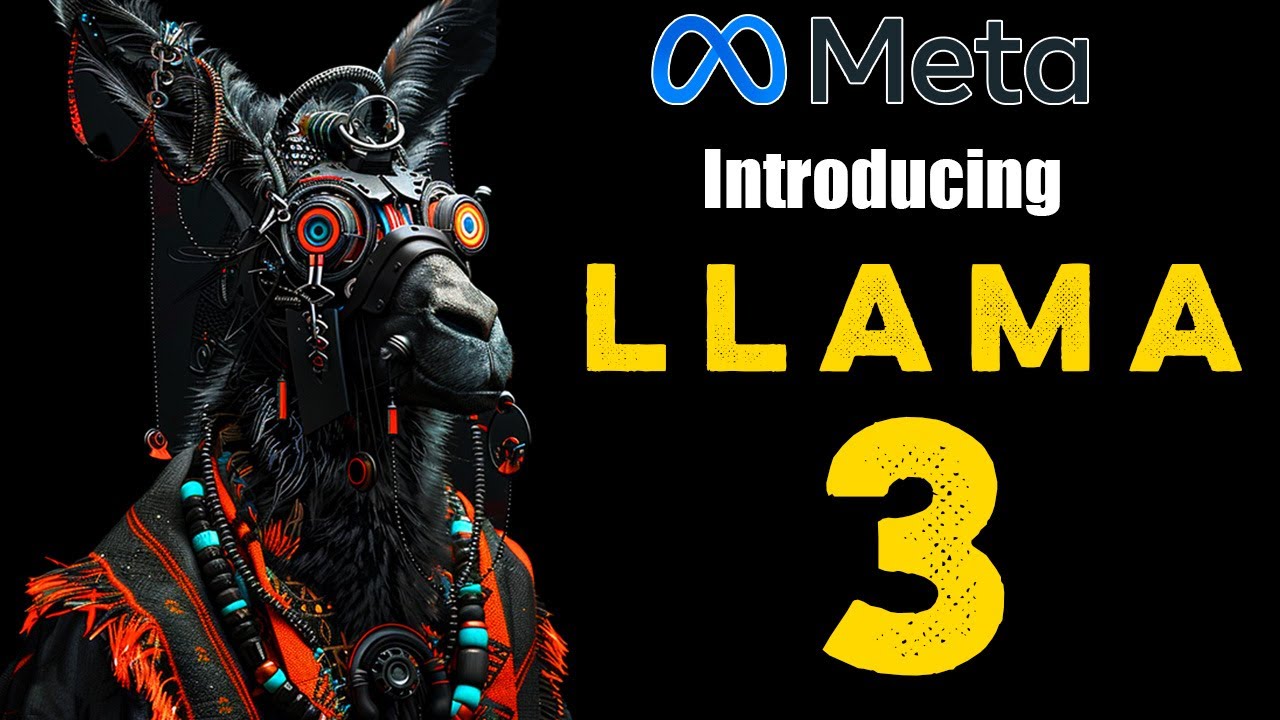
Key Takeaways at a Glance
00:00
Meta's LLAMA 3 models are open-sourced with exceptional performance.02:43
LLAMA 3's upcoming 400 billion parameter model is a game-changer.05:33
Open-sourcing AI models promotes transparency and security.13:09
Synthetic data is a key enabler for training advanced AI models.16:44
Training AI models on code enhances their capabilities.17:20
Synthetic data generation plays a crucial role in AI model training.19:27
Balancing training and inference processes is vital for AI model advancement.22:14
Limitations exist in scaling AI models infinitely.24:32
Physical constraints and infrastructure challenges impact AI model progress.26:19
LLAMA 3 requires inclusion in AI model names.27:25
Scaling challenges in training large language models.30:46
Importance of balancing AI safety and open technology.
1. Meta's LLAMA 3 models are open-sourced with exceptional performance.
🥇92
00:00
LLAMA 3 models with 8 billion and 70 billion parameters show best-in-class performance, with upcoming releases promising multimodality and larger context windows.
- LLAMA 3 models are leading in performance for their scale.
- Future releases will introduce multimodality and larger context windows.
- Open-sourcing LLAMA 3 models allows for broader access and experimentation.
2. LLAMA 3's upcoming 400 billion parameter model is a game-changer.
🥇94
02:43
The upcoming LLAMA 3 model with 400 billion parameters is anticipated to surpass Gemini Pro 1.5 and compete with Cloud 3 Opus and GPT-4 Turbo.
- The 400 billion parameter model is expected to set a new standard in AI capabilities.
- LLAMA 3's advancements pose a significant challenge to existing top models.
- This model's release could unlock vast research potential and energy in the ecosystem.
3. Open-sourcing AI models promotes transparency and security.
🥈89
05:33
Making AI models open source fosters transparency, collaboration, and ensures a more balanced playing field in AI development.
- Open source AI models allow for broader scrutiny and improvement.
- Enhanced security through collective development and shared standards.
- Balanced growth and sophistication across the AI landscape.
4. Synthetic data is a key enabler for training advanced AI models.
🥈88
13:09
Using synthetic data generated by AI proves effective in training advanced AI models, overcoming limitations posed by the availability of real-world data.
- Synthetic data offers a solution to data scarcity for training AI models.
- Recent research indicates the efficacy of synthetic data in enhancing AI model training.
- Synthetic data usage facilitates continuous improvement and expansion of AI capabilities.
5. Training AI models on code enhances their capabilities.
🥇92
16:44
Training AI models on code, even if not intended for coding tasks, improves their general abilities and skill set.
- Using code data for training enhances model generalization.
- Access to large models for synthetic data extraction can boost model performance.
- Training on code data can lead to significant improvements in AI models.
6. Synthetic data generation plays a crucial role in AI model training.
🥇94
17:20
Synthetic data generation is increasingly utilized to train AI models, leveraging outputs from existing models to enhance performance.
- Synthetic data extraction from large models aids in training new models effectively.
- Utilizing synthetic data from advanced models can potentially surpass original model performance.
- Generating synthetic data is a key aspect of training advanced AI models.
7. Balancing training and inference processes is vital for AI model advancement.
🥈88
19:27
The future of AI model development may involve a shift towards more emphasis on generating synthetic data for inference to feed back into models.
- The balance between training and inference processes is critical for model evolution.
- Synthetic data generation could become a primary focus in advancing AI models.
- Inference-driven synthetic data creation may shape the future of AI model training.
8. Limitations exist in scaling AI models infinitely.
🥈89
22:14
There are fundamental constraints preventing unlimited scaling of AI models beyond certain levels, curbing runaway intelligence growth.
- Physical limitations and network architecture constraints restrict model scalability.
- Compounding corruption over multiple generations may hinder exponential model improvements.
- Concerns about rapid, extreme intelligence growth are tempered by existing constraints.
9. Physical constraints and infrastructure challenges impact AI model progress.
🥈87
24:32
The development of AI models faces challenges related to physical limitations, infrastructure requirements, and chip shortages.
- GPU chip shortages pose significant bottlenecks in AI model training.
- Global infrastructure investments of trillions are needed to support AI advancements.
- Challenges in chip availability and infrastructure development affect AI model growth.
10. LLAMA 3 requires inclusion in AI model names.
🥈85
26:19
LLAMA 3 usage mandates naming AI models with LLAMA 3 at the beginning, ensuring compliance with terms of use.
- Companies using LLAMA 3 to train models must name them with LLAMA 3 at the start.
- Enforcement of naming conventions ensures acknowledgment and adherence to LLAMA 3 usage terms.
11. Scaling challenges in training large language models.
🥈88
27:25
Scaling demands innovative strategies beyond better scaling laws and infrastructure, like managing training across 16k GPUs effectively.
- 400 billion parameter models are still in training, emphasizing the complexity of scaling.
- Innovative strategies are crucial to handle high computational demands in training large models.
12. Importance of balancing AI safety and open technology.
🥇92
30:46
Balancing AI safety research with open technology development is crucial to prevent misuse by authoritarian regimes or bad actors.
- Ensuring open access to AI technology while prioritizing safety measures is essential for preventing misuse.
- Potential dangers include ideological manipulation and control if AI falls into wrong hands.