Metas New A.I Statement Actually SHOCKED Everyone!
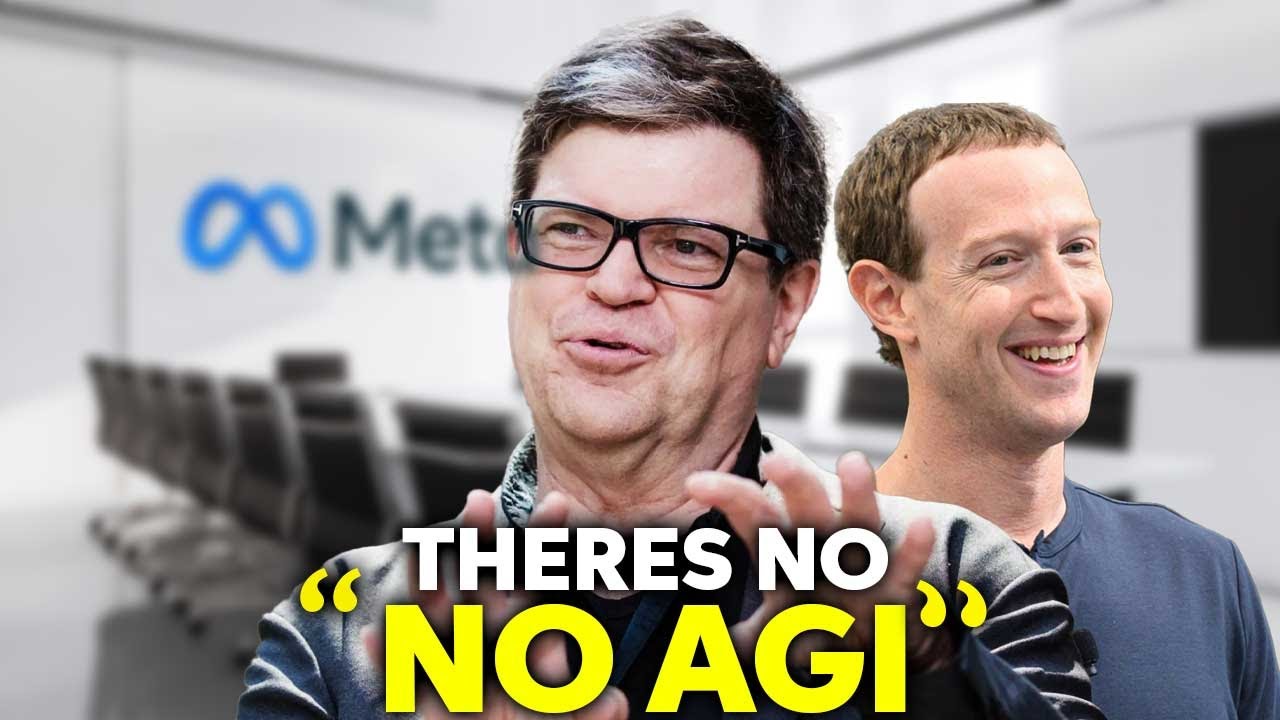
Key Takeaways at a Glance
00:00
Meta's Chief Scientist challenges the concept of AGI.02:57
Limitations of large language models (LLMs) are highlighted.06:46
Meta's AI Chief advocates for non-generative AI models.10:00
Future AI advancements may require a paradigm shift.13:52
Text-based training is insufficient for achieving human-level AI.17:41
AI development towards AGI will be a gradual process.23:29
AI systems need to evolve with new principles and technologies.23:45
Hardware innovation is crucial for advancing AI capabilities.26:39
Future of data centers: Light-based scaling for AGI.31:22
Importance of developing next-gen AI systems beyond NNs.32:34
Evolution of AI systems: Transition towards GPT-5 level advancements.
1. Meta's Chief Scientist challenges the concept of AGI.
🥇92
00:00
Yanin argues that instead of focusing on AGI, we should aim to replicate human and animal intelligence in AI systems, emphasizing efficient learning for diverse applications.
- Current AI lacks general intelligence compared to humans and animals.
- Efficient machine learning akin to human learning is crucial for future AI assistance.
- Shifting focus from AGI to replicating human intelligence poses intriguing industry challenges.
2. Limitations of large language models (LLMs) are highlighted.
🥈87
02:57
Yanin critiques LLMs for their limited logic understanding, lack of physical world comprehension, and absence of persistent memory, essential for effective learning.
- LLMs struggle with basic logic tasks and lack understanding of the physical world.
- Persistent memory is crucial for enhancing AI intelligence and learning capabilities.
- Challenges in logic, physical world understanding, and memory hinder LLMs from achieving human-level intelligence.
3. Meta's AI Chief advocates for non-generative AI models.
🥈89
06:46
Yanin promotes the development of non-generative AI models like VJEA, emphasizing efficient learning, understanding the physical world, reasoning, and hierarchical planning.
- VJEA utilizes self-supervised learning without predefined labels for efficient learning.
- Abstract representation in VJEA enhances understanding of complex concepts.
- Non-generative models like VJEA aim to revolutionize AI by mirroring human learning processes.
4. Future AI advancements may require a paradigm shift.
🥈85
10:00
Yanin suggests moving away from scaling LLMs and investing in alternative AI research for smarter systems with less data dependency, potentially reshaping the AI landscape.
- Rethinking AI strategies beyond LLM scaling could lead to significant advancements.
- Embracing new AI approaches may redefine the future of AI intelligence.
- Shifting focus from LLMs to innovative AI systems could revolutionize AI capabilities.
5. Text-based training is insufficient for achieving human-level AI.
🥇92
13:52
Relying solely on text for training AI will not lead to human or animal intelligence levels due to text saturation.
- Training from text has limitations due to saturation even with vast amounts of data.
- Animal and human learning is not primarily text-based but involves non-linguistic elements.
- AI needs to move beyond text to understand and learn from various modalities.
6. AI development towards AGI will be a gradual process.
🥈89
17:41
Achieving Artificial General Intelligence (AGI) will involve incremental progress, not a sudden breakthrough event.
- AGI development requires advancements in learning from videos, associative memories, reasoning, and planning.
- Building systems with human-level intelligence will take at least a decade or more due to various challenges.
- Superintelligence emergence will not be an abrupt event but a gradual ramp-up in intelligence levels.
7. AI systems need to evolve with new principles and technologies.
🥈85
23:29
Developing AI systems that can reason, plan, and learn hierarchically requires new principles and fabrication technologies.
- Advancements in hardware and architectural innovation are crucial for AI systems to reach human brain compute power levels.
- Efforts are ongoing to enhance energy efficiency and processing speed in AI hardware.
- Combining transformative technologies like Transformers and CETS can lead to more efficient AI systems.
8. Hardware innovation is crucial for advancing AI capabilities.
🥈87
23:45
Improving hardware efficiency, reducing power consumption, and exploring new fabrication technologies are essential for AI progress.
- Current hardware is far less efficient than the human brain in terms of power consumption.
- Innovations like photonic chips offer energy-efficient computing with faster data processing.
- Investment in hardware advancements like photonic chips can significantly impact AI scalability and efficiency.
9. Future of data centers: Light-based scaling for AGI.
🥇96
26:39
Light Matter is revolutionizing data centers by using light for data transfer, enabling massive scaling for AGI and next-gen models.
- Scaling with light allows for larger chip sizes and increased interconnectivity.
- Eliminating traditional networking equipment paves the way for all-to-all interconnectivity.
- Reducing energy consumption and enabling scaling to a million nodes are key benefits.
10. Importance of developing next-gen AI systems beyond NNs.
🥇92
31:22
Focusing on advancing AI beyond NNs is crucial for overcoming limitations and progressing towards AGI.
- Encouraging young AI researchers to work on systems that surpass NNs.
- Predictions suggest AGI arrival between 2025 and 2030, signaling a significant shift.
- Emphasizing the need for architectures like Vjeer for future AI advancements.
11. Evolution of AI systems: Transition towards GPT-5 level advancements.
🥈88
32:34
Anticipating advancements like GPT-5 and breakthroughs in AI development to redefine the landscape.
- Expectations for overcoming limitations and showcasing the potential of AI advancements.
- Upcoming years are poised to reveal significant progress and understanding in AI evolution.
- Potential for validating existing approaches or introducing groundbreaking innovations.