Mixtral of Experts (Paper Explained)
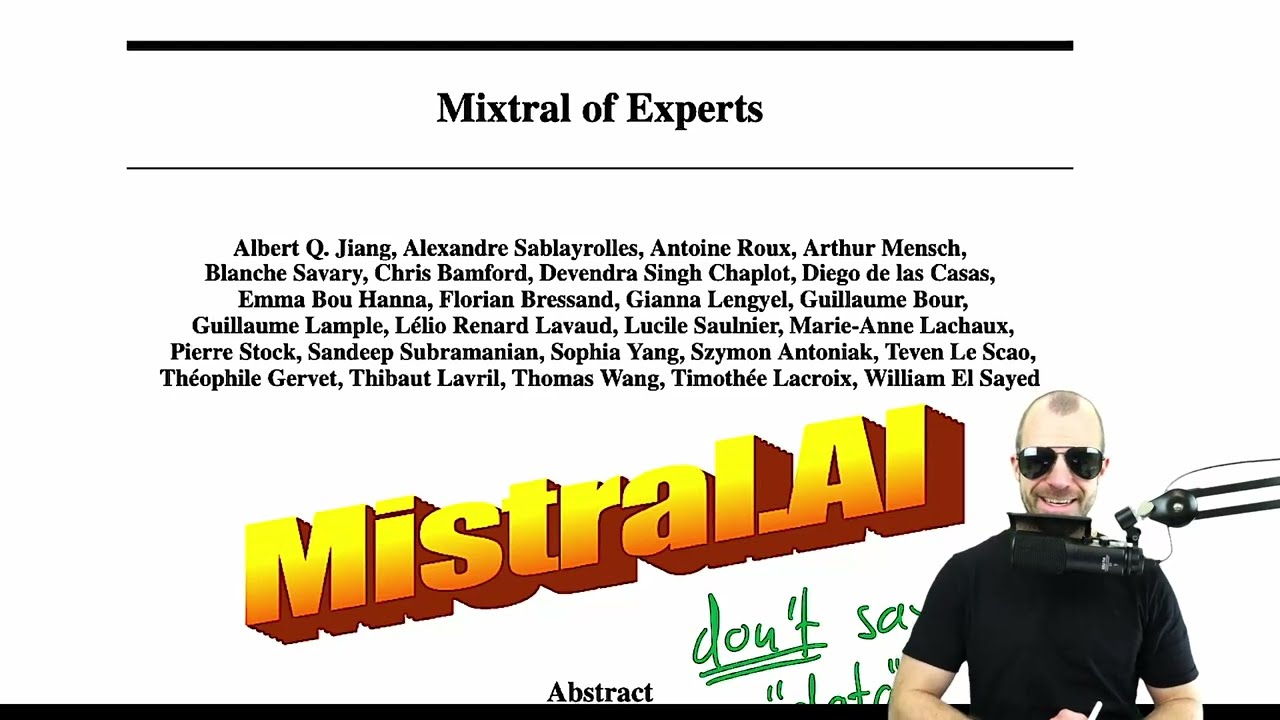
Key Takeaways at a Glance
00:00
Importance of open source approach in AI startups00:00
Significance of training data transparency00:00
Understanding the core components of Transformer models03:00
Mixture of Experts model architecture explained17:00
Challenges and advantages of sparse mixture of experts22:25
Expert parallelism enables high throughput processing.22:28
Expert parallelism for high throughput processing.25:00
Experimental results demonstrate competitive performance.31:30
Routing analysis reveals lack of obvious patterns in expert assignments.33:20
Conclusion emphasizes the release of models under Apache license.34:15
Mixtral of Experts is an exciting concept.
1. Importance of open source approach in AI startups
🥈85
00:00
Mistl AI's open source approach sets it apart from other AI startups, allowing for greater accessibility and flexibility in model usage.
- Models are released under the Apache License, providing freedom to utilize the models as needed.
- Contrasts with other startups that have usage restrictions despite disclosing data sources.
2. Significance of training data transparency
🥉78
00:00
The paper's decision not to disclose training data sources addresses concerns about data privacy and copyright issues.
- Reflects a strategic choice to avoid potential legal and ethical complications related to training data.
- Raises questions about the trade-offs between transparency and reproducibility in research papers.
3. Understanding the core components of Transformer models
🥈82
00:00
Transformer models consist of input tokens, embedding layers, Transformer blocks, attention mechanisms, and feed forward networks.
- Attention mechanisms enable context-dependent computation and information exchange between tokens.
- Feed forward networks apply to individual tokens, contributing to the model's parameter count.
4. Mixture of Experts model architecture explained
🥇92
03:00
The Mixr of Experts model is built on the Mistl 7B architecture and features a sparse mixture of experts model with open weights under Apache 2.
- It outperforms other models on various benchmarks.
- Utilizes a subset of parameters for each token, allowing for optimizations in speed and throughput.
5. Challenges and advantages of sparse mixture of experts
🥈88
17:00
Sparse mixture of experts allows for significant computation savings by utilizing a subset of experts for each token, resulting in a smaller active parameter count.
- The routing neural network decides which expert a token should be routed to, based on the token's intermediate representation.
- This approach leads to faster inference speed at low batch sizes and higher throughput at large batch sizes.
6. Expert parallelism enables high throughput processing.
🥇92
22:25
Implementing expert parallelism by assigning different experts to different GPUs can significantly increase processing throughput.
- Assigning experts to different GPUs makes it appear as a dense operation to each GPU.
- This approach is particularly effective for high throughput scenarios with large batch sizes.
7. Expert parallelism for high throughput processing.
🥈89
22:28
Assigning experts to different GPUs enables dense operations, significantly boosting processing speed for high throughput scenarios.
- This approach is particularly effective for large batch sizes and can enhance overall processing efficiency.
- It allows for efficient utilization of resources and accelerates model performance.
8. Experimental results demonstrate competitive performance.
🥈88
25:00
The experimental results show that the model either matches or outperforms other models such as Llama 270 billion parameter model and GPT-3.5.
- The model's performance is compared to other models like Llama 2, demonstrating its competitive edge.
- The results indicate the model's effectiveness in various tasks.
9. Routing analysis reveals lack of obvious patterns in expert assignments.
🥈85
31:30
The analysis of token routing to different experts indicates a lack of clear semantic patterns in expert assignments.
- Consecutive tokens often go to the same expert, but no clear semantic patterns are observed.
- This suggests that the routing may be based on different aspects of the tokens rather than semantic patterns.
10. Conclusion emphasizes the release of models under Apache license.
🥇91
33:20
The conclusion highlights the release of models under Apache license, enabling widespread use and application development.
- The release under Apache license facilitates the development of new applications and technologies by a wide range of developers.
- This move is seen as a significant contribution to the community.
11. Mixtral of Experts is an exciting concept.
🥈85
34:15
Mixtral of Experts is a promising and exciting concept with potential applications in various fields.
- It is seen as a cool and innovative concept.
- There is anticipation for its impact on the world as it becomes more open source.