NeurIPS 2023 Poster Session 3 (Wednesday Evening)
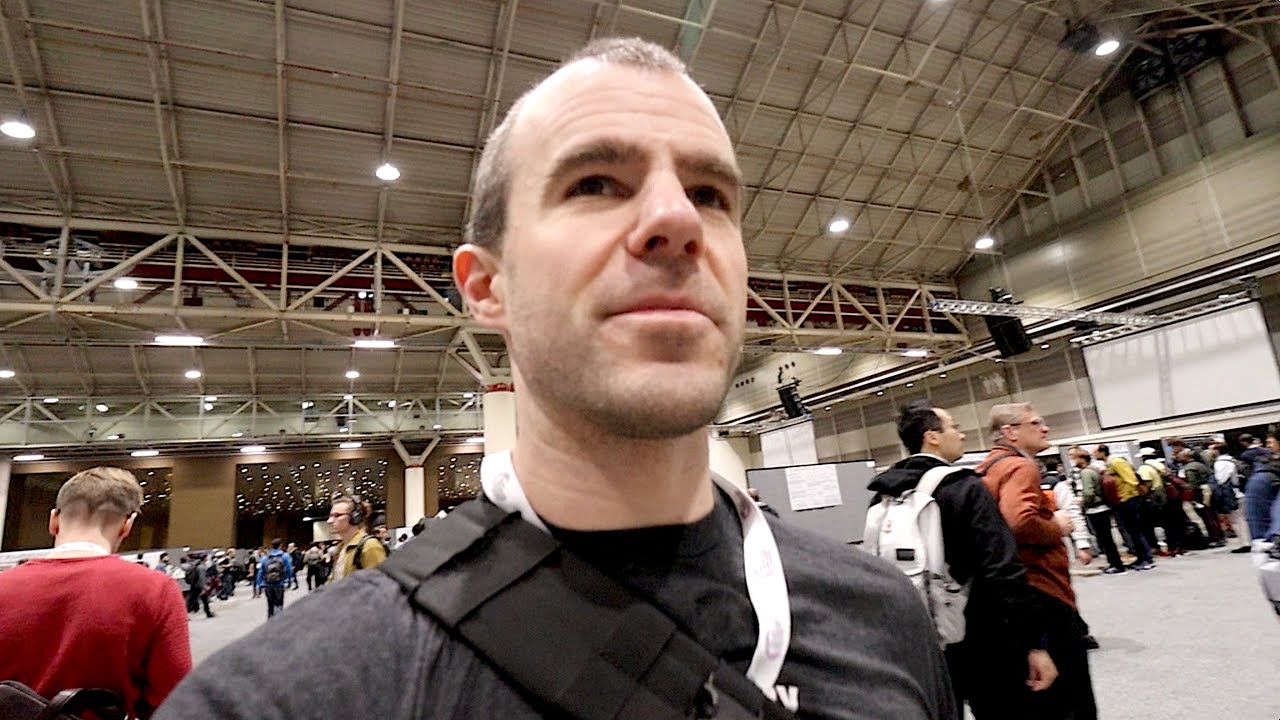
Key Takeaways at a Glance
00:20
Graph of circuits is used to explore the optimal design space.01:00
Graph surrogate model approximates the behavior of a simulator.05:10
Constraint optimization is used to find the most optimal design parameters.- NeurIPS 2023 Poster Session 3 (Wednesday Evening)
15:20
Empowering Collaborative Filtering with Principled Adversarial Contrastive Loss22:11
Cluster-aware Semi-supervised Learning: Relational Knowledge Distillation Provably Learns Clustering28:30
Privacy Assessment on Reconstructed Images: Are Existing Evaluation Metrics Faithful to Human Perception?29:39
Proposed method for evaluating information leakage in images.31:22
Importance of using a universal metric for evaluating different datasets and attack methods.32:53
Future work on scaling the information leakage evaluation.
1. Graph of circuits is used to explore the optimal design space.
🥈85
00:20
The graph of circuits is a representation of a circuit design problem, where each node corresponds to a circuit component and the edges represent the connections between them.
- The graph is used to solve the pre-layout designing problem and optimize the design parameters.
- It helps in minimizing the objective function while satisfying the specifications of the circuit.
2. Graph surrogate model approximates the behavior of a simulator.
🥈88
01:00
The graph surrogate model is trained using a graph neural network (GNN) on labeled circuit instances.
- The GNN predicts labels for unlabeled circuit instances, approximating the behavior of a simulator.
- This approach reduces the computational cost of generating labeled samples for optimization.
3. Constraint optimization is used to find the most optimal design parameters.
🥇92
05:10
Constraint optimization algorithms, such as ESASO and ASO, are applied to the graph surrogate model to find the design parameters that satisfy the specifications and minimize the objective function.
- ESASO is a static optimization framework, while ASO is a dynamic learning framework.
- These algorithms iteratively optimize the design parameters based on the labels predicted by the graph surrogate model.
4. NeurIPS 2023 Poster Session 3 (Wednesday Evening)
🥉78
The content is a transcript of the NeurIPS 2023 Poster Session 3 held on Wednesday evening.
- The session likely covered various topics related to machine learning and artificial intelligence.
- The transcript provides insights into the discussions and research presented during the session.
5. Empowering Collaborative Filtering with Principled Adversarial Contrastive Loss
🥇92
15:20
Principled adversarial contrastive loss can improve generalization ability in recommendation systems by finding a better loss function.
- The loss function should achieve better generalization ability, meaning it performs well even when the testing and training data have different distributions.
- Adversarial training can be used to learn the loss function, which is equivalent to solving a distributional robust problem.
6. Cluster-aware Semi-supervised Learning: Relational Knowledge Distillation Provably Learns Clustering
🥈88
22:11
Relational knowledge distillation (RKD) can be used to learn clustering structure in semi-supervised learning.
- RKD helps the student model learn the clustering structure revealed by the teacher model.
- By leveraging the clustering structure, the student model can achieve good generalization and reduce the number of labeled samples needed.
7. Privacy Assessment on Reconstructed Images: Are Existing Evaluation Metrics Faithful to Human Perception?
🥈82
28:30
Existing evaluation metrics for privacy assessment on reconstructed images may not align with human perception.
- Metrics that suggest similarity between reconstructed and original images may not accurately reflect human perception.
- This can lead to privacy leakage if the wrong candidates are selected based on the metrics.
8. Proposed method for evaluating information leakage in images.
🥈85
29:39
The proposed method involves training a model using a triplate loss and comparing the feature vectors of original and reconstructed images.
- The model is trained using anchor, positive, and negative samples.
- The generated scores align well with human observers' judgments.
9. Importance of using a universal metric for evaluating different datasets and attack methods.
🥇92
31:22
Different metrics perform well for different datasets and attack methods, highlighting the need for a universal metric.
- The proposed method consistently performs well across different datasets and attack methods.
- Changing the training setup or loss function also improves the results.
10. Future work on scaling the information leakage evaluation.
🥉78
32:53
The authors plan to work on scaling the evaluation to a larger scale, from 1 to 10, to capture different levels of information leakage.
- This is an area for further research and improvement.
- The dataset, labels, and codes will be made accessible for others to use.