Open AI Q* (Q-STAR) Exposed - NEW Hidden Details Of Q*
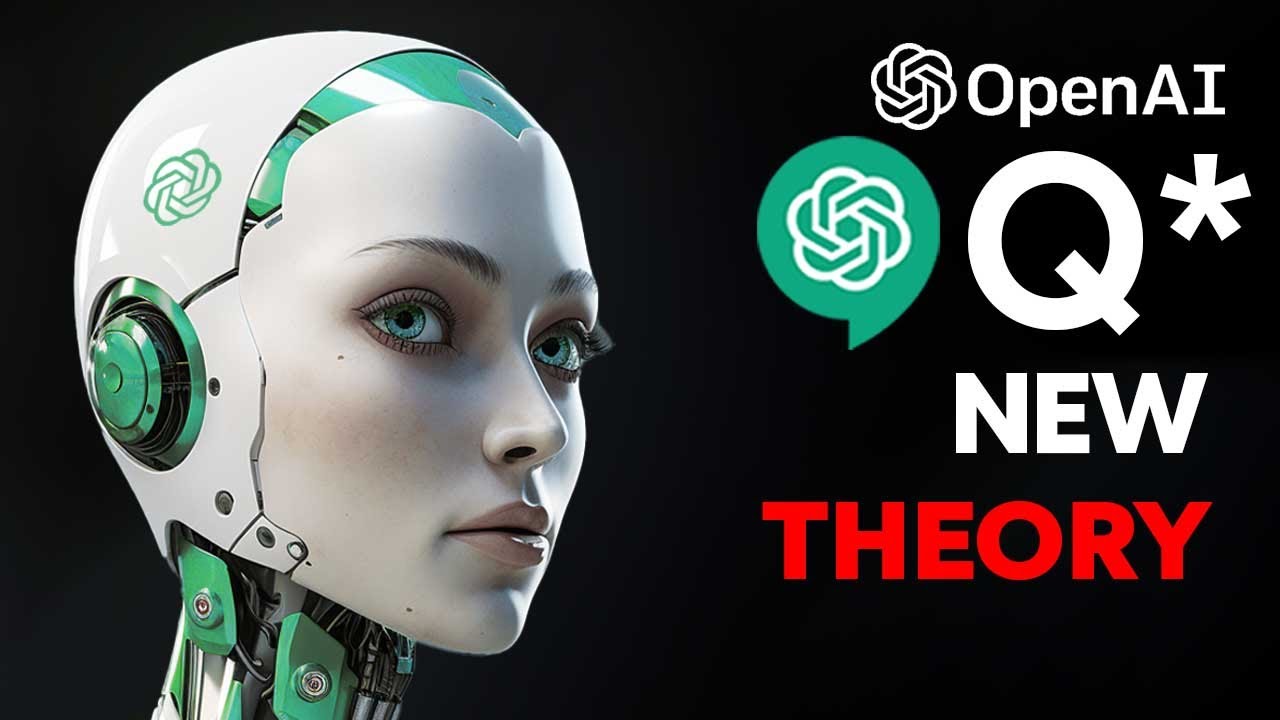
Watch video on YouTube. Use this note to help digest the key points better.
Key Takeaways at a Glance
00:27
Confirmation of Q* leak by Sam Alman.01:04
Q* leak suggests a major breakthrough at Open AI.02:05
Possible connection between Q* leak and Meta's Llama leak.04:04
Implications of Q* leak on encryption and security.13:00
AI's potential to surpass human mathematicians.16:34
OpenAI collaborated with DARPA on cyber security.17:15
Q* combines math and Q learning for reinforcement learning.18:21
OpenAI's research on cryptography is plausible.20:10
The leaked letter strengthens the claims about qstar.25:29
The leak about qstar is credible and hard to disprove.28:04
OpenAI's breakthrough in qstar is significant.30:13
Improving gameplay through self-play and look ahead planning.30:37
Modular reasoning and tree of thoughts in language models.31:22
Reinforcement learning in a multi-step fashion.
1. Confirmation of Q* leak by Sam Alman.
🥈85
00:27
Sam Alman confirmed that the Q* leak was true, indicating that the leaked information about Q* is indeed accurate.
- This confirmation adds credibility to the leaked information.
- The leaked information may have significant implications for AI technology.
2. Q* leak suggests a major breakthrough at Open AI.
🥈82
01:04
The Q* leak indicates that there was a significant breakthrough at Open AI, pushing the boundaries of what is possible with AI technology.
- Open AI is known for constantly pushing the frontiers of AI technology.
- The leaked information suggests that Open AI is making rapid progress in this area.
3. Possible connection between Q* leak and Meta's Llama leak.
🥉78
02:05
The Q* leak and Meta's Llama leak both involved powerful AI language models and were leaked in a similar manner.
- The similarity in the leaks raises questions about the authenticity of the Q* leak.
- The Q* leak may have some validity if the Llama leak was indeed true.
4. Implications of Q* leak on encryption and security.
🥈86
04:04
If the Q* leak is true, it could have significant implications for encryption and security.
- The leak suggests that AI may have advanced to a level where it can crack encryption algorithms.
- This poses a grave threat to the security of sensitive computer data.
5. AI's potential to surpass human mathematicians.
🥈81
13:00
If AI is able to crack major encryption algorithms, it would demonstrate a level of mathematical understanding beyond human capabilities.
- AI's ability to analyze mathematics and come up with new formulas could have far-reaching implications.
- This could lead to breakthroughs and advancements that surpass human capabilities.
6. OpenAI collaborated with DARPA on cyber security.
🥈85
16:34
OpenAI collaborated with DARPA on a cyber security challenge called The Dara AI cyber security Challenge.
- OpenAI trained a special version of Q* on Dara's computers.
- This collaboration suggests that OpenAI has expertise in reinforcement learning and cryptography.
7. Q* combines math and Q learning for reinforcement learning.
🥈82
17:15
Q* is an LLM trained on math combined with Q learning, a reinforcement learning technique.
- OpenAI has expertise in reinforcement learning and has used it to achieve superhuman capabilities in various fields.
- Q* uses optimal policies and aligns with what people speculate about qstar.
8. OpenAI's research on cryptography is plausible.
🥉78
18:21
OpenAI's research on cryptography is not completely impossible, given their expertise in AI algorithms and pattern prediction.
- OpenAI's AI algorithms are good at pattern selection and prediction.
- Their collaboration with DARPA and expertise in reinforcement learning make it plausible that they have worked on cryptography.
9. The leaked letter strengthens the claims about qstar.
🥈86
20:10
The leaked letter, despite being intended to disprove the claims, actually strengthens the claims about qstar.
- The letter shows an expert level of understanding of AI research and cryptography.
- The references to Project Tundra and the to analysis technique support the claims about qstar.
10. The leak about qstar is credible and hard to disprove.
🥈87
25:29
The leak about qstar is credible and has not been successfully disproven, despite attempts to do so.
- The leak contains niche and specialized information that is difficult to fake.
- The timing of the leak and the lack of earlier mentions of qstar on the internet add to its credibility.
11. OpenAI's breakthrough in qstar is significant.
🥈81
28:04
OpenAI's breakthrough in qstar is significant, even if previous breakthroughs have not lived up to initial expectations.
- Breakthroughs in AI often work in specific contexts and may not generalize.
- OpenAI's qstar breakthrough is different from previous ones and has potential implications for encryption and information systems.
12. Improving gameplay through self-play and look ahead planning.
🥈85
30:13
An agent can enhance its gameplay by playing against slightly different versions of itself. Look ahead planning involves using a model of the world to reason into the future and produce better actions or outputs.
- These techniques have been used in AlphaGo and other AI systems.
- Model predictive control is often used for continuous state, while Monte Carlo tree search works on discrete actions and states.
13. Modular reasoning and tree of thoughts in language models.
🥉78
30:37
Language models like GPT-4 use modular reasoning with tree of thoughts and other methods of prompting to improve their base systems.
- These techniques are important for enhancing large language models.
- The article suggests that QAR uses PRMs to score the tree of thoughts reasoning data, which is then optimized with offline reinforcement learning.
14. Reinforcement learning in a multi-step fashion.
🥈82
31:22
Reinforcement learning can be done in a multi-step fashion, using a sequence of reasoning steps instead of contextual bandits.
- This approach is an interesting hypothesis for the future of reinforcement learning.
- It may have implications for AGI and the development of GPT-5.