OpenAI's Q* is back! Is this the real reason Ilya left OpenAI?
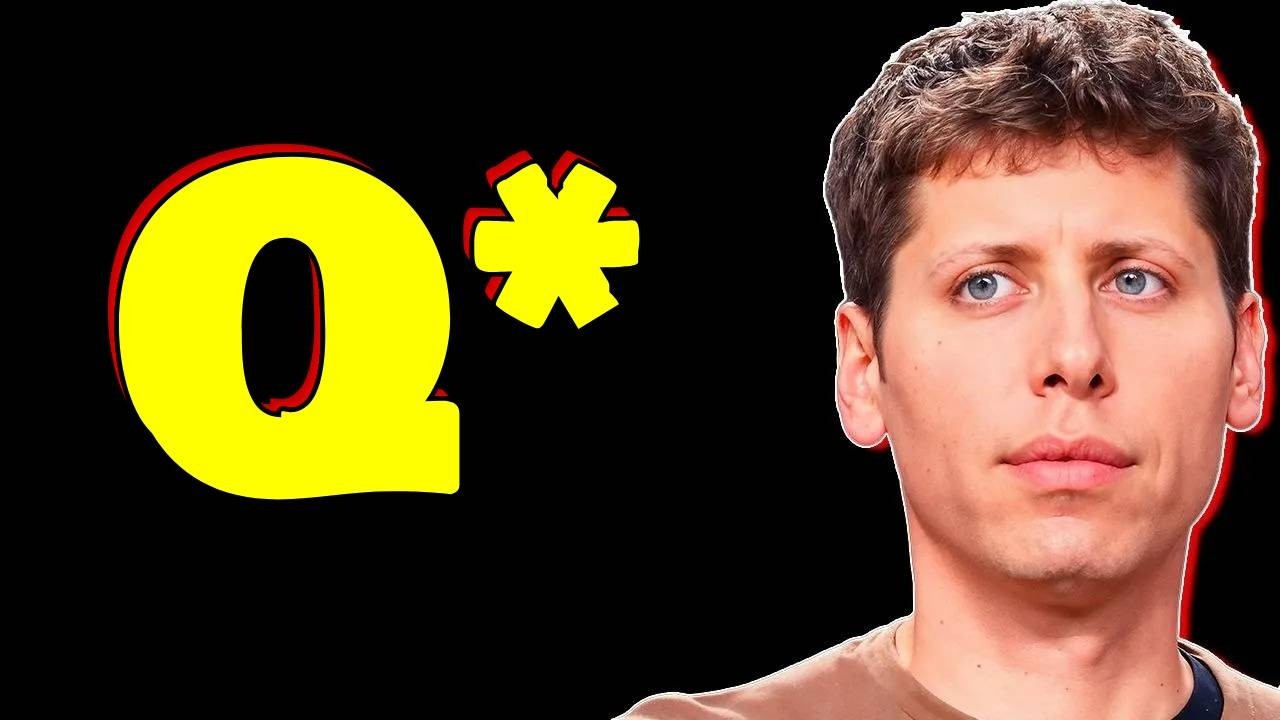
Key Takeaways at a Glance
02:35
OpenAI's Q* represents a significant advancement in AI reasoning.03:03
OpenAI's Q* leak sparks speculation and interest in the AI community.03:41
Google DeepMind's approach to AI involves self-play for skill enhancement.11:52
Merging narrow and general AI branches could lead to new AI capabilities.13:39
Generalist AI mastering 3D virtual environments is crucial.15:42
AI's proficiency in video games signals future advancements.17:28
Synthetic data training propels AI capabilities.19:39
Future AI models may outperform human-generated data.25:45
AI models can predict and describe actions in videos.27:46
AI advancements leverage video data for diverse applications.29:55
AI models evolve through transfer learning and generative models.31:51
AI development focuses on memory augmentation and hierarchical reinforcement learning.35:33
AI advancements aim for superintelligence through continuous learning.38:23
Speculation on Ilya's departure hints at potential AI advancements.38:50
OpenAI's focus on Super Intelligence is strategic.
1. OpenAI's Q* represents a significant advancement in AI reasoning.
🥇92
02:35
Q* integrates neural and symbolic methodologies to enhance AI reasoning for solving complex problems and planning.
- Q* focuses on improving AI reasoning for tasks requiring cumulative learning and advanced planning.
- It aims to outperform current AI technologies in various cognitive tasks.
2. OpenAI's Q* leak sparks speculation and interest in the AI community.
🥈85
03:03
The leaked information about Q* from OpenAI has generated curiosity and discussions among AI researchers and experts.
- Many knowledgeable individuals in the AI field have commented on the potential impact of Q*.
- Speculations suggest Q* could represent a significant advancement in AI technology.
3. Google DeepMind's approach to AI involves self-play for skill enhancement.
🥈89
03:41
DeepMind's AI models like AlphaGo and AlphaStar achieve superhuman skills through self-play and reinforcement learning.
- Self-play allows AI to improve by playing against itself millions of times.
- This approach leads to AI becoming proficient and even surpassing human capabilities in specific tasks.
4. Merging narrow and general AI branches could lead to new AI capabilities.
🥈88
11:52
Combining superhuman narrow AI with more general AI approaches may result in novel AI capabilities.
- The integration of different AI methodologies could pave the way for advancements towards AGI or ASI.
- Video games serve as a platform for evolving AI capabilities through merging different AI branches.
5. Generalist AI mastering 3D virtual environments is crucial.
🥇96
13:39
Mastering 3D virtual environments can lead to generalizing AI agents across various realities, enhancing autonomy and adaptability.
- AI mastering 10,000 simulations may generalize to the real physical world.
- A single agent across axes can be foundational, scaling up massively for training.
- Future vision includes all AI agents as prompts to a Foundation agent.
6. AI's proficiency in video games signals future advancements.
🥇92
15:42
AI's expected superhuman proficiency in video games by mid-2026 hints at transformative advancements in competitive gaming and potential open-source AI versions.
- Training in competitive games enhances strategic thinking and decision-making.
- AI-generated high-quality data from gameplay drives skill levels to superhuman standards.
- AI's gameplay produces vast amounts of training data for self-improvement.
7. Synthetic data training propels AI capabilities.
🥇98
17:28
AI's training on synthetic data, including self-play, generates high-quality data, enabling AI to surpass human-generated data and excel in diverse domains.
- AI's ability to create new games and strategies through self-play enhances its capabilities.
- Synthetic data training leads to efficient problem-solving and skill generalization.
- AI's reinforcement and self-supervised learning refine strategies to superhuman levels.
8. Future AI models may outperform human-generated data.
🥇94
19:39
AI's potential to surpass human data generation with high-quality synthetic data raises questions about economic models and societal implications.
- AI's capacity to create superior art, music, and text prompts reevaluation of human roles.
- AI's ability to generate new knowledge challenges traditional data creation and usage models.
- AI's reliance on synthetic data may reshape economic participation globally.
9. AI models can predict and describe actions in videos.
🥇92
25:45
Models like GPT-4 with vision can annotate and predict actions in video frames, enabling detailed descriptions and future action predictions.
- Annotates video frames to describe actions and predict subsequent actions.
- Capable of providing precise descriptions of player actions in complex 3D environments.
- Generalizes learning abilities across various domains beyond gaming.
10. AI advancements leverage video data for diverse applications.
🥈88
27:46
AI learning from video data extends to medical, surveillance, and gaming applications, enhancing automation and decision-making in various fields.
- Video data analysis can aid in medical monitoring and alert systems.
- Enables automation in surveillance tasks and behavioral analysis.
- Utilizes video footage for training AI agents in diverse scenarios.
11. AI models evolve through transfer learning and generative models.
🥈87
29:55
Transfer learning from gaming to other domains, neuro-symbolic AI, and generative models enhance AI creativity and problem-solving capabilities.
- Transfer gaming strategies to new fields for problem-solving.
- Combines neural networks with symbolic reasoning for abstract concepts.
- Generative models simulate human-like creativity and problem-solving.
12. AI development focuses on memory augmentation and hierarchical reinforcement learning.
🥈89
31:51
Incorporating memory mechanisms and reinforcement learning strategies enhances AI reasoning, planning, and adaptability for complex tasks.
- Memory mechanisms improve information retention and utilization.
- Hierarchical reinforcement learning optimizes strategies at multiple levels.
- Enhances AI's ability to plan, execute strategies, and adapt to new challenges.
13. AI advancements aim for superintelligence through continuous learning.
🥇91
35:33
Combining methodologies like multi-agent collaboration and continuous learning pushes AI capabilities towards achieving superintelligence and generalizing skills across domains.
- Multi-agent collaboration enhances AI's ability to learn diverse skills and strategies.
- Continuous learning methodologies elevate AI capabilities to superhuman levels.
- Aims to generalize AI skills beyond video games to various fields.
14. Speculation on Ilya's departure hints at potential AI advancements.
🥈88
38:23
Ilya's departure and potential involvement in new AI ventures raise questions about hidden AI breakthroughs and their impact on the industry.
- Ilya's deep involvement in AI projects suggests insider knowledge of significant advancements.
- The secrecy surrounding potential AI discoveries fuels speculation about the future of AI development.
- Ilya's departure may signal a shift in the competitive landscape of AI research.
15. OpenAI's focus on Super Intelligence is strategic.
🥇92
38:50
OpenAI's emphasis on Super Intelligence over AGI indicates a deliberate strategic direction towards a specific goal for AI development.
- Prioritizing Super Intelligence aligns with a focused approach for AI advancement.
- The choice to target SSI suggests a clear roadmap for OpenAI's research and development.
- This strategic focus may differentiate OpenAI's objectives from other AI initiatives.