OpenAI'S Q-STAR Has More SHOCKING LEAKED Details! (Open AI Q*)
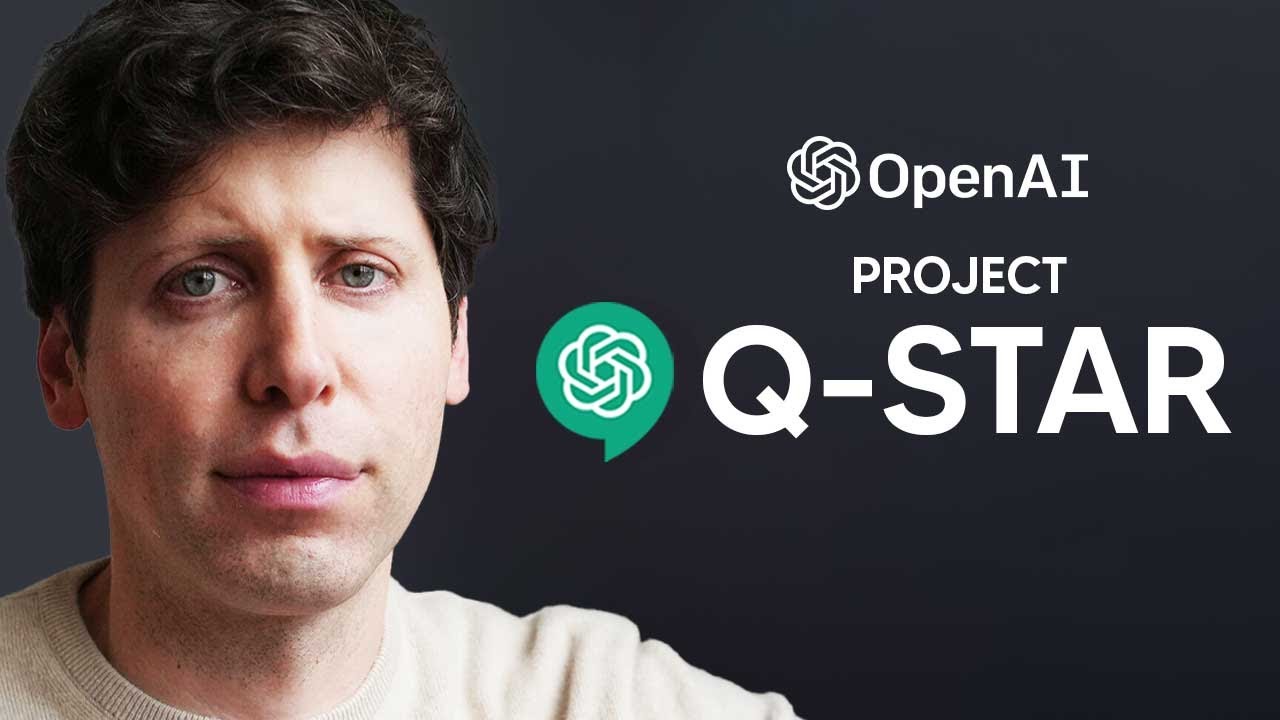
Key Takeaways at a Glance
00:53
QAR introduces a novel approach to dialogue systems.01:53
QAR leverages energy-based models for dialogue generation.02:34
QAR optimizes responses through abstract representation space.03:58
QAR promises advancements in AI reasoning and interaction.05:44
QAR's potential impact extends to various problem-solving domains.11:05
OpenAI's QAR leak sparks discussions on AI advancements.12:36
Meta is exploring unique advantages in representation for improved AI performance.12:57
Sora's video generation method diverges from traditional RL formulations.
1. QAR introduces a novel approach to dialogue systems.
🥇92
00:53
QAR shifts focus to internal deliberation akin to human thought processes, enhancing decision-making in complex problem-solving scenarios.
- QAR aims to mimic human thought processes during tasks like chess playing.
- It focuses on deeper analysis for better decision-making compared to rapid responses.
- The model emphasizes inferring latent variables for improved dialogue system operation.
2. QAR leverages energy-based models for dialogue generation.
🥈89
01:53
QAR's core is an energy-based model that evaluates response compatibility with a given prompt, optimizing for better answers.
- Lower energy values indicate more compatible responses.
- The model evaluates responses holistically beyond token predictions.
- QAR's mechanism enhances understanding of response relevance and appropriateness.
3. QAR optimizes responses through abstract representation space.
🥈88
02:34
QAR's innovation lies in optimizing responses in an abstract representation space, refining thoughts to yield the best answers.
- The model minimizes energy in abstract thought space for optimal responses.
- It employs gradient descent for iterative refinement towards low-energy representations.
- QAR bridges non-linguistic conceptual understanding to linguistic output for human interaction.
4. QAR promises advancements in AI reasoning and interaction.
🥈87
03:58
QAR's approach offers a more efficient, reasoned, and potentially powerful method for generating dialog responses, enhancing AI's ability for human-like reasoning and interaction.
- The system aims for improved text quality and sets a path for future AI advancements.
- QAR introduces a departure from traditional language modeling techniques.
- It utilizes gradient-based inference for enhanced dialog generation.
5. QAR's potential impact extends to various problem-solving domains.
🥈86
05:44
QAR's energy-based models offer a flexible and powerful approach for handling complex situations with multiple solutions, potentially revolutionizing planning processes.
- Energy-based models excel in scenarios with numerous solutions.
- Traditional planning methods struggle with extensive possibilities, making energy-based models a promising alternative.
- QAR's approach is considered highly flexible and efficient for diverse problem-solving contexts.
6. OpenAI's QAR leak sparks discussions on AI advancements.
🥈85
11:05
Despite skepticism around the leak's authenticity, it hints at OpenAI's breakthroughs in AI reasoning and planning, stimulating interest in energy-based models.
- The leak aligns with discussions on latent space planning and search areas in AI.
- OpenAI's potential utilization of energy-based models for unseen math problems sparks speculation and interest.
- The leak prompts considerations of AI's future capabilities in reasoning and problem-solving.
7. Meta is exploring unique advantages in representation for improved AI performance.
🥇92
12:36
Utilizing representations in latent token sequences rather than original space offers significant advantages for AI tasks, enhancing optimization efficiency.
- Reducing compound error and planning horizon are key benefits of this approach.
- Moving away from fixed state SL action representation can boost problem-solving effectiveness.
- Meta's focus on innovative representation methods hints at advancements in AI capabilities.
8. Sora's video generation method diverges from traditional RL formulations.
🥈89
12:57
Sora's approach involves constructing and redefining latent token sequences before decoding them, leading to more effective planning and search processes.
- This method surpasses fixed state SL action representation, enhancing optimization efficiency.
- Meta's exploration of alternative video generation techniques showcases a commitment to cutting-edge AI development.