OpenAIs Surprising New Plan For Superintelligence...
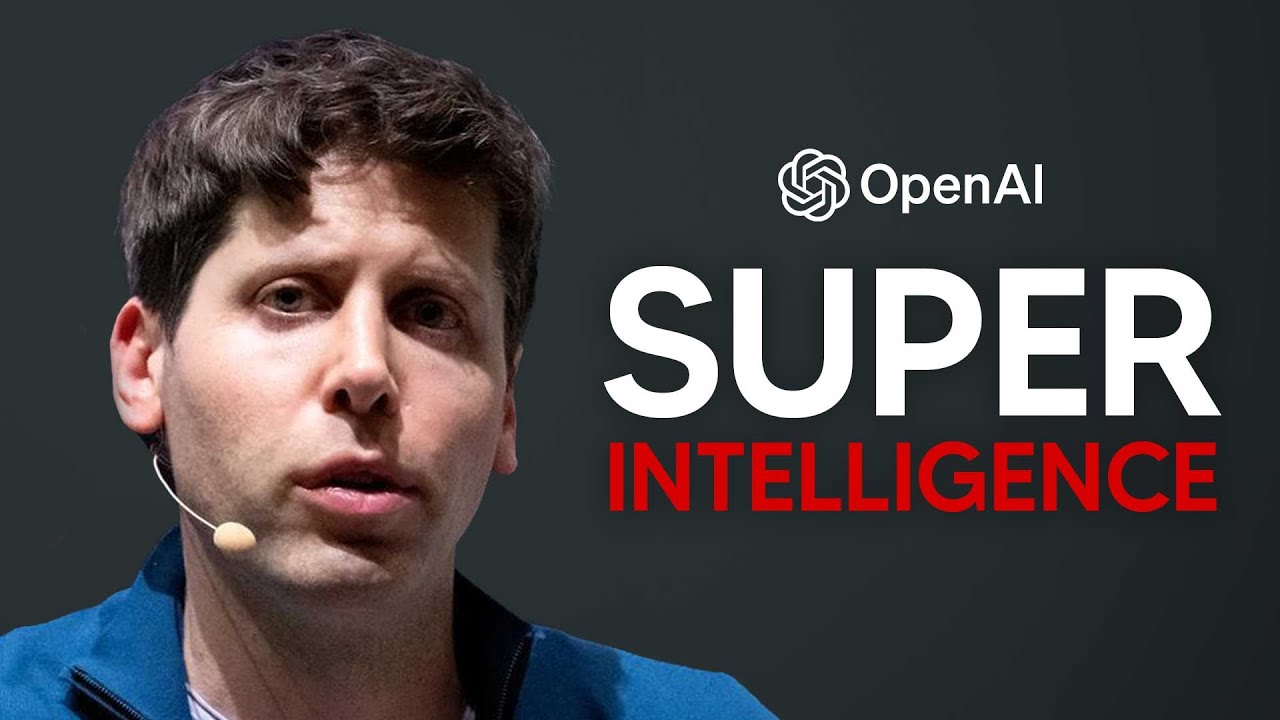
Key Takeaways at a Glance
00:54
AI achieving superhuman intelligence through reinforcement learning in video games.07:10
Emergence of innovative solutions through unsupervised learning in AI.10:23
Generalizing skills learned in video games to real-world applications.11:33
AI agents evolving to be more versatile and helpful across environments.12:34
SEMA outperforms specialized agents in 3D games.14:37
Superintelligence within reach with advanced AI models.17:11
Monte Carlo tree search enhances AI decision-making.21:14
Neuro-symbolic AI combines neural networks with logical reasoning.26:42
Importance of combining online learning with abstraction for AI advancement.27:32
Diversification in AI approaches is crucial for achieving Artificial General Intelligence (AGI).
1. AI achieving superhuman intelligence through reinforcement learning in video games.
🥇96
00:54
OpenAI's approach to developing superhuman intelligence involves reinforcement learning in video games, enabling AI to refine strategies iteratively to superhuman levels.
- Reinforcement learning allows AI to continuously improve performance through feedback from the game environment.
- AI systems can play millions of times, learning from mistakes and successes to refine strategies.
- OpenAI has successfully used reinforcement learning in video games like Dota 2 to surpass human champions.
2. Emergence of innovative solutions through unsupervised learning in AI.
🥇94
07:10
AI systems can develop unique and innovative problem-solving abilities through unsupervised learning, leading to emergent behaviors and solutions.
- AI agents can discover novel solutions without explicit training for specific outcomes.
- Training AI systems in simulated environments can lead to unexpected and creative problem-solving approaches.
- Innovative abilities can emerge from AI systems playing millions of times and exploring various strategies.
3. Generalizing skills learned in video games to real-world applications.
🥈89
10:23
Skills and strategies acquired in video games can be generalized to other domains like mathematics, science, and real-world problem-solving.
- Strategic thinking and planning from games can be applied to fields beyond gaming.
- Training AI agents in diverse video game environments enhances their adaptability to different tasks and environments.
- Advanced AI models aim to improve understanding and action based on higher-level language instructions.
4. AI agents evolving to be more versatile and helpful across environments.
🥈87
11:33
Advancements in AI models aim to create more versatile and helpful AI agents that can follow instructions in various settings, potentially benefiting any environment.
- Enhancing AI agents' ability to follow instructions in diverse game settings can lead to more helpful AI agents in real-world applications.
- Generalizing AI capabilities from video games to real-world actions through language interfaces is a key research focus.
- Using video games as training sandboxes can aid in understanding how AI systems can become more beneficial in different contexts.
5. SEMA outperforms specialized agents in 3D games.
🥇92
12:34
SEMA surpasses agents trained for specific games, showcasing superior adaptability and generalization capabilities.
- SEMA excels in unseen games despite lacking specific training for those games.
- Generalization beyond training data is crucial for robotic success.
6. Superintelligence within reach with advanced AI models.
🥈89
14:37
Advancements in AI models like SEMA and neuro-symbolic AI hint at the proximity of achieving superintelligence.
- Combining neural networks with symbolic reasoning enhances AI's cognitive abilities.
- Neuro-symbolic AI integration crucial for AGI development.
7. Monte Carlo tree search enhances AI decision-making.
🥈87
17:11
Monte Carlo tree search method aids AI in evaluating strategies and selecting optimal moves based on simulations.
- AlphaZero's success in chess demonstrates the effectiveness of Monte Carlo tree search.
- MCTS allows AI to make strategic decisions with limited search positions.
8. Neuro-symbolic AI combines neural networks with logical reasoning.
🥈85
21:14
Neuro-symbolic AI merges neural networks' pattern recognition with symbolic AI's logical thinking, enhancing AI's problem-solving capabilities.
- Combining neural networks with rule-based reasoning leads to more effective AI systems.
- AlphaGo's success showcases the power of integrating neural and symbolic AI components.
9. Importance of combining online learning with abstraction for AI advancement.
🥇92
26:42
Scaling alone is insufficient; deep learning struggles with reasoning and factuality. Neuro-symbolic approaches offer hope by combining learning and abstraction.
- Deep learning faces challenges in reasoning despite vast data and models.
- Pure symbolic AI is not the ultimate solution due to knowledge generation limitations.
- Neuro-symbolic AI, with more resources, could revolutionize the field.
10. Diversification in AI approaches is crucial for achieving Artificial General Intelligence (AGI).
🥈89
27:32
Intelligence is multifaceted; a one-size-fits-all solution is inadequate. Combining neuro-symbolic AI with large-scale knowledge is essential for progress.
- No single approach can solve the complexity of intelligence.
- AGI requires a combination of diverse AI methodologies and extensive knowledge bases.
- LLMs represent only a fraction of human intelligence, necessitating a broader AI pipeline.