ORPO: Monolithic Preference Optimization without Reference Model (Paper Explained)
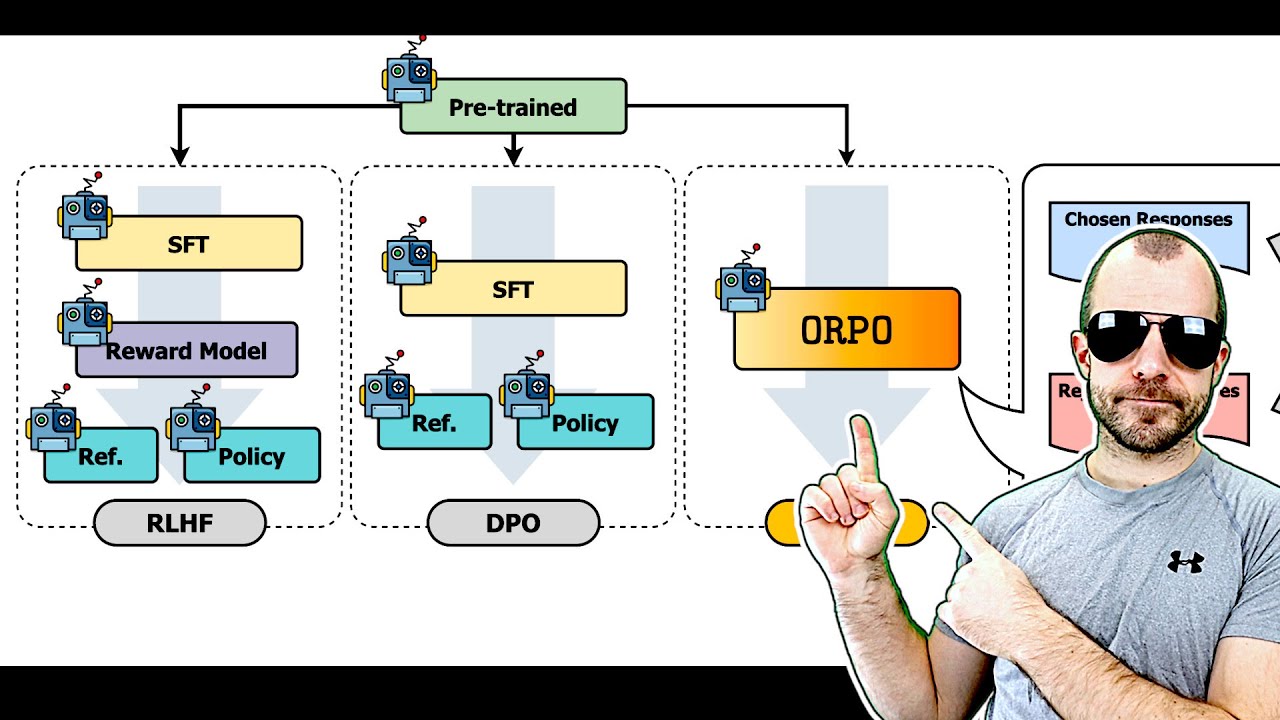
Key Takeaways at a Glance
03:05
Alignment enhances model outputs to match desired criteria.06:12
ORPO simplifies preference optimization by integrating steps.17:43
ORPO addresses limitations of supervised fine-tuning for alignment.18:10
ORPO's odds ratio loss integrates preference data for alignment.18:27
Understanding odds and odds ratio is crucial for modeling.20:01
Logarithmic transformations play a key role in loss functions.26:14
Weighted contrast gradients enhance model adaptation.30:59
Preferential use of odds ratio over probability ratios for balanced distributions.
1. Alignment enhances model outputs to match desired criteria.
π₯89
03:05
Alignment in ORPO focuses on improving model outputs to align with preferred responses, increasing the likelihood of desired answers and decreasing undesired ones.
- Alignment aims to make model outputs more consistent with expected responses.
- The process involves adjusting model outputs based on preference ratings.
- ORPO ensures that model responses are in line with user expectations.
2. ORPO simplifies preference optimization by integrating steps.
π₯92
06:12
ORPO streamlines the process by combining supervised fine-tuning and alignment steps into one procedure, eliminating the need for multiple models and reducing computational requirements.
- ORPO merges supervised fine-tuning and alignment into a unified approach.
- This integration reduces the complexity of the optimization process.
- By combining steps, ORPO enhances efficiency and computational resource utilization.
3. ORPO addresses limitations of supervised fine-tuning for alignment.
π₯87
17:43
ORPO overcomes the shortcomings of supervised fine-tuning by introducing an auxiliary loss to differentiate between desired and undesired model outputs.
- The method corrects the failure of supervised fine-tuning to distinguish between preferred and non-preferred responses.
- By incorporating an auxiliary loss, ORPO ensures better alignment of model outputs.
- The approach enhances the ability to train models to produce desired responses effectively.
4. ORPO's odds ratio loss integrates preference data for alignment.
π₯88
18:10
ORPO utilizes an odds ratio loss that incorporates preference data to guide model training towards generating responses aligned with user preferences.
- The odds ratio loss considers the likelihood of desired responses given specific inputs.
- By leveraging preference data, ORPO optimizes model outputs to match user expectations.
- The odds ratio loss mechanism enhances the alignment process in ORPO.
5. Understanding odds and odds ratio is crucial for modeling.
π₯92
18:27
Odds indicate the likelihood of an event happening compared to not happening, while the odds ratio compares odds between two outcomes.
- Odds show how much more likely an event is to happen than not.
- Odds ratio is the ratio of odds between two outcomes, aiding in comparing probabilities.
6. Logarithmic transformations play a key role in loss functions.
π₯88
20:01
Taking the log of odds ratios and applying non-linearities helps in transforming probabilities for effective modeling.
- Log transformations help in converting values into different spaces for better analysis.
- Non-linearities aid in mapping probabilities to desired ranges for optimization.
7. Weighted contrast gradients enhance model adaptation.
π₯87
26:14
Introducing weighted contrast gradients based on likelihood amplifies model adaptation towards chosen responses, improving alignment.
- Amplifying gradients for low likelihood responses accelerates model adaptation towards desired outputs.
- Balancing gradients based on likelihood enhances model performance and alignment.
8. Preferential use of odds ratio over probability ratios for balanced distributions.
π₯89
30:59
Utilizing odds ratio over probability ratios provides a wider range of values for modeling, ensuring a more balanced and stable distribution.
- Odds ratio offers a more evenly distributed set of values for effective modeling.
- Probability ratios tend to create spiky distributions, limiting modeling flexibility.