SakanaAI Unveils "Transformer²" AI That EVOLVES at Inference Time
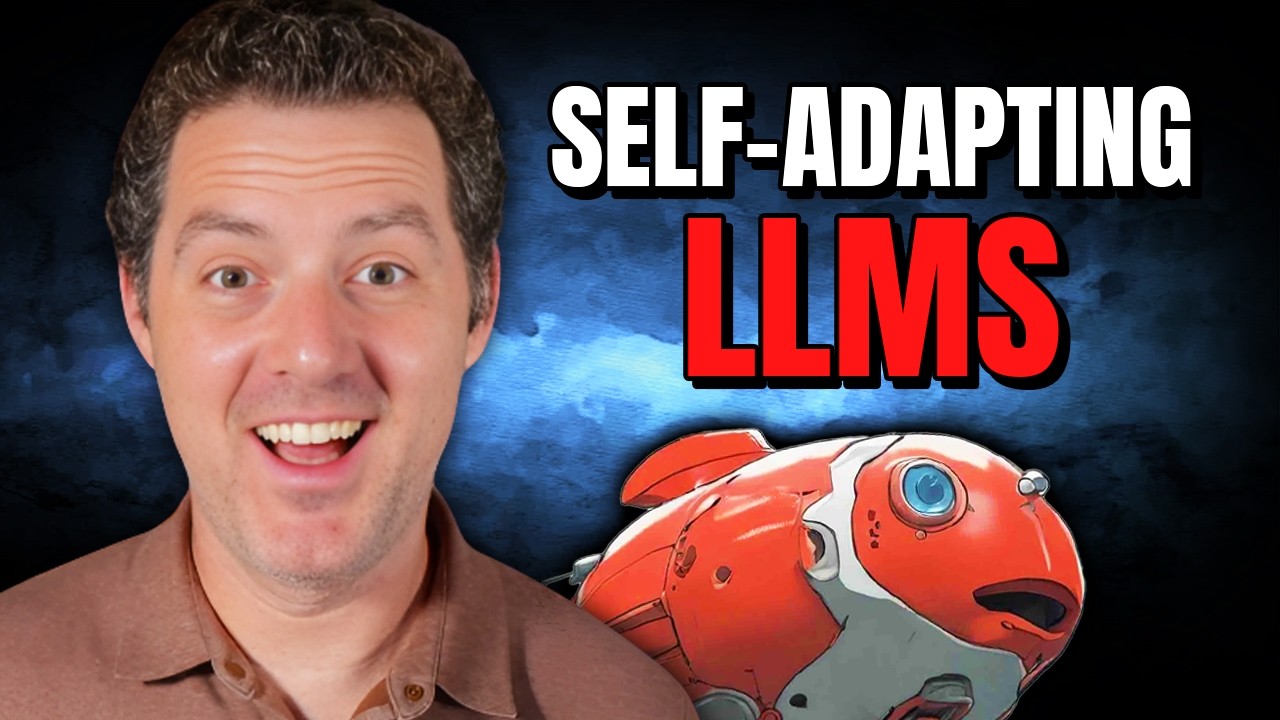
Key Takeaways at a Glance
00:13
Transformer² allows real-time model updates during inference.02:07
Self-adaptive models improve efficiency and flexibility.03:04
The two-pass system enhances task understanding.08:14
Singular value fine-tuning optimizes model adjustments.09:51
Transformer² outperforms traditional fine-tuning methods.14:50
Efficiency is a key advantage of Transformer².17:00
Prompt engineering enhances task identification accuracy.17:49
Transformer² AI allows models to evolve during inference.
1. Transformer² allows real-time model updates during inference.
🥇95
00:13
SakanaAI's Transformer² can adapt its model weights at inference time based on user prompts, enhancing its response accuracy.
- This two-pass approach first identifies the task type before updating weights.
- It aims to overcome the static nature of traditional models that do not learn post-training.
- The method is open-source and applicable to any open-source model.
2. Self-adaptive models improve efficiency and flexibility.
🥇92
02:07
Transformer² proposes a self-adaptive framework that selectively adjusts model weights for unseen tasks, enhancing efficiency over traditional fine-tuning methods.
- This approach allows for continual learning without catastrophic forgetting.
- It dynamically modifies behavior based on task demands without constant retuning.
- Expert modules can be developed offline and integrated on demand.
3. The two-pass system enhances task understanding.
🥇90
03:04
The first pass of Transformer² identifies the task properties, while the second pass applies expert vectors for targeted behavior.
- This method ensures that the model understands the prompt before making adjustments.
- It utilizes reinforcement learning to train task-specific expert vectors.
- The approach mirrors how the human brain adapts to different tasks.
4. Singular value fine-tuning optimizes model adjustments.
🥈88
08:14
Transformer² employs singular value fine-tuning to efficiently update model weights, focusing on essential parameters.
- This method minimizes the number of parameters needing training, enhancing performance.
- It allows for flexible composition of expert modules tailored to specific tasks.
- The approach is designed to prevent overfitting and improve adaptability.
5. Transformer² outperforms traditional fine-tuning methods.
🥇91
09:51
The new model demonstrates superior performance and efficiency compared to existing fine-tuning strategies.
- It achieves better results with fewer parameters than traditional methods.
- The efficiency gains are significant, making it a promising advancement in AI.
- Performance metrics indicate consistent improvements across various tasks.
6. Efficiency is a key advantage of Transformer².
🥇92
14:50
Transformer² demonstrates improved performance with significantly fewer resources compared to traditional models, making it a more efficient option for various tasks.
- It requires less than 10% of the training parameters of previous implementations.
- The second pass inference time is minimal, adding only a small fraction to overall runtime.
- This efficiency is particularly beneficial for complex tasks like the Arc Challenge.
7. Prompt engineering enhances task identification accuracy.
🥈88
17:00
Utilizing prompt engineering and classification experts significantly improves the model's ability to identify tasks accurately during inference.
- The model achieved high accuracy rates, particularly with classification expert prompts.
- However, it struggled with specific tasks like the Arc Challenge using prompt engineering.
- This highlights the importance of tailored prompts for optimal performance.
8. Transformer² AI allows models to evolve during inference.
🥇95
17:49
The Transformer² architecture enables models to adapt and learn over time, enhancing their efficiency and performance during inference without needing constant retraining.
- This method allows model weights to change post-training, which is a significant advancement.
- It reduces the need for frequent new model releases, streamlining the development process.
- The approach has shown improvements across various tasks and models.