Strawberry 2.0 - AI Breakthrough Unlocks New Scaling Law
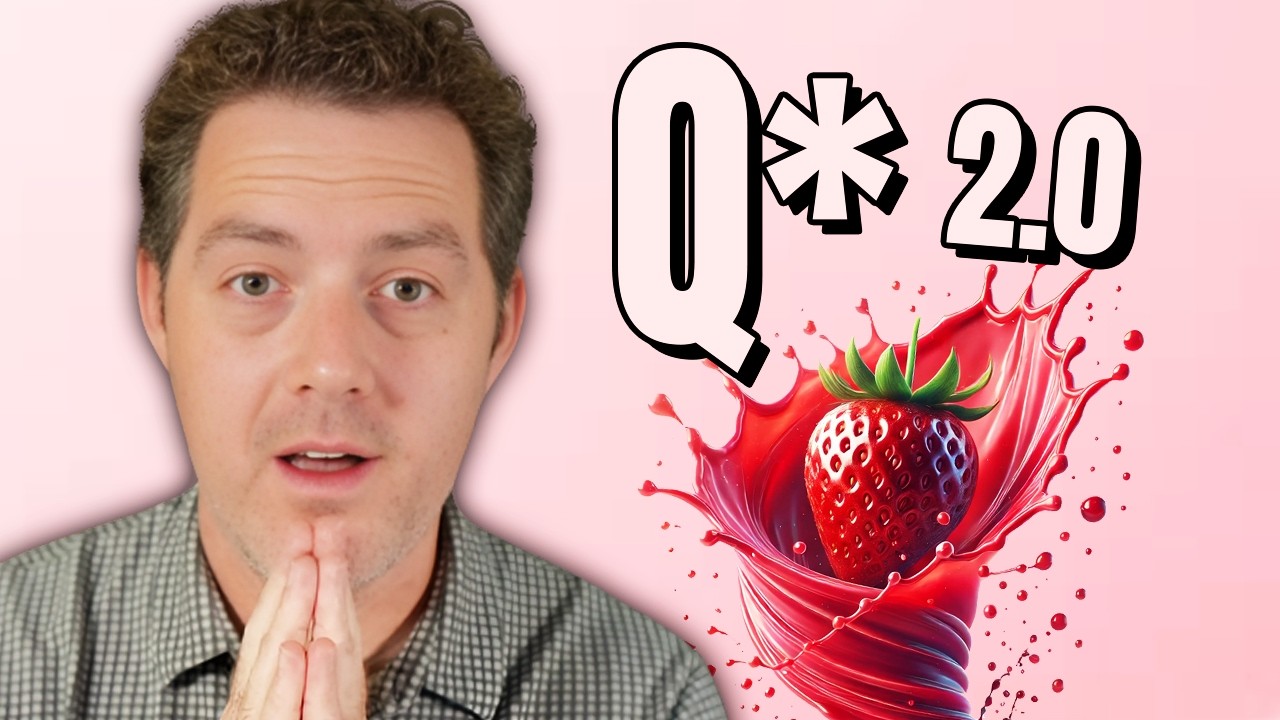
Key Takeaways at a Glance
01:55
The ARC prize benchmarks AGI capabilities effectively.04:50
Test time training significantly enhances model performance.06:10
Small models can achieve impressive results with new techniques.09:06
Dynamic parameter updates enhance inference capabilities.11:05
Augmented inference methods improve model predictions.
1. The ARC prize benchmarks AGI capabilities effectively.
🥈88
01:55
The ARC prize serves as a public competition aimed at developing solutions for AGI benchmarks, focusing on generalization and reasoning.
- Participants must open-source their solutions, promoting transparency and collaboration.
- The average human score on these tests is around 60, providing a baseline for AI performance.
- The competition highlights the challenges AI faces in generalizing from training data to novel problems.
2. Test time training significantly enhances model performance.
🥇95
04:50
The new technique of test time training allows models to update parameters during inference, leading to a substantial improvement in accuracy on complex reasoning tasks.
- This method achieved a score of 61.9 on the ARC prize, surpassing the previous best of 42.
- It enables models to adapt dynamically to new problems by generating training data from test inputs.
- The technique shows that proper computational resource allocation is crucial for solving novel reasoning problems.
3. Small models can achieve impressive results with new techniques.
🥇90
06:10
Recent advancements show that smaller models can perform exceptionally well when combined with innovative training methods like test time training.
- An 8 billion parameter model achieved a 53% accuracy on the ARC validation set, improving the state of the art by nearly 25%.
- Efficient fine-tuning methods allow these models to adapt without extensive retraining.
- The focus on smaller models emphasizes efficiency and accessibility in AI development.
4. Dynamic parameter updates enhance inference capabilities.
🥇92
09:06
Test time training allows models to temporarily update their parameters based on the specific test input, improving prediction accuracy.
- This process involves generating variations of the test problem to create a rich training dataset.
- The model reverts to its original parameters after each inference, maintaining efficiency.
- This dynamic approach challenges traditional assumptions about the necessity of symbolic reasoning in AI.
5. Augmented inference methods improve model predictions.
🥈87
11:05
Techniques like augmented inference and ensembling predictions enhance the performance of language models during testing.
- These methods involve generating multiple candidate predictions and selecting the best through a voting process.
- Geometric transformations are used to create diverse training examples from a single problem.
- This approach helps models better handle tasks with multiple potential solutions.