Test Time Scaling is Bigger Than Anyone Thinks (Proof)
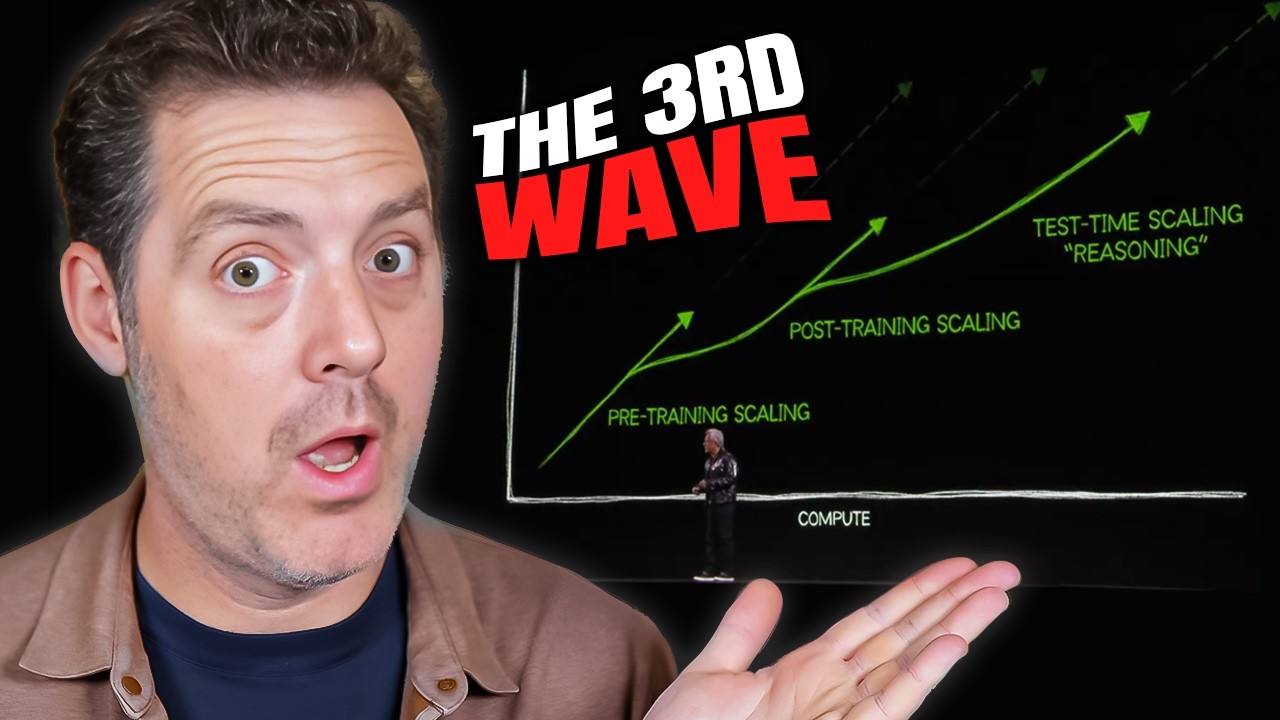
Key Takeaways at a Glance
00:00
Test time scaling significantly enhances AI model performance.03:00
Longer thinking times lead to better AI outcomes.04:06
Process reward models improve AI reasoning capabilities.07:48
Inference time scaling is a growing market opportunity.11:19
Cost of inference time scaling remains a challenge.13:54
AI models are evolving to handle more complex tasks.14:30
Inference time scaling can significantly enhance model performance.15:33
Diffusion models require additional compute for better results.16:09
Focus on inference time for future AI innovations.
1. Test time scaling significantly enhances AI model performance.
π₯95
00:00
Test time scaling allows AI models to think long-term, improving their outputs by utilizing more tokens during inference, which is crucial for advanced reasoning tasks.
- This approach enables models to generate multiple candidate responses and select the best one.
- Research indicates that scaling inference time computation can yield better results than merely increasing model parameters.
- The ability to think longer on complex problems mirrors human cognitive processes.
2. Longer thinking times lead to better AI outcomes.
π₯88
03:00
Just as humans take time to solve complex problems, AI models benefit from extended inference periods to enhance their decision-making processes.
- Models that utilize more thinking time can achieve significantly higher performance on challenging prompts.
- The ability to evaluate multiple ideas before arriving at a conclusion is crucial for complex tasks.
- This approach unlocks new possibilities for reasoning and agentic tasks in AI.
3. Process reward models improve AI reasoning capabilities.
π₯90
04:06
Unlike outcome reward models, process reward models reward AI for each step taken towards a solution, enhancing its ability to refine answers.
- This method allows models to retain correct steps even if the final answer is incorrect.
- It encourages models to think iteratively, improving overall decision-making.
- Understanding this distinction is critical for developing more effective AI systems.
4. Inference time scaling is a growing market opportunity.
π₯92
07:48
Industry leaders predict that inference time scaling will surpass pre-training in market size, indicating a shift in focus towards optimizing AI performance during use.
- Companies like AMD and Nvidia emphasize the importance of inference in their future strategies.
- The demand for computation in AI is expected to increase as models become more capable.
- Investments in AI technology are likely to drive down costs, further expanding market opportunities.
5. Cost of inference time scaling remains a challenge.
π₯85
11:19
While inference time scaling offers substantial benefits, the associated costs can be prohibitively high, especially for extensive computations.
- Running advanced models can require hundreds of thousands of dollars due to high token usage.
- As technology advances, the cost of inference is expected to decrease, making it more accessible.
- Understanding the financial implications is essential for businesses looking to leverage AI.
6. AI models are evolving to handle more complex tasks.
π₯87
13:54
Recent advancements in AI, particularly in inference time scaling, are enabling models to tackle increasingly sophisticated challenges.
- New techniques are being developed to apply inference time scaling to various AI applications, including image generation.
- The evolution of AI capabilities is expected to lead to a broader range of use cases.
- This shift highlights the importance of continuous innovation in AI technology.
7. Inference time scaling can significantly enhance model performance.
π₯92
14:30
Recent research shows that increasing computation during inference can improve performance in large language models and diffusion models.
- Diffusion models can adjust inference time computation through denoising steps.
- Performance gains typically plateau after a few dozen denoising steps.
- The ability to use more compute during the diffusion process leads to higher quality results.
8. Diffusion models require additional compute for better results.
π₯89
15:33
The proposed framework allows diffusion models to utilize more computation, leading to improved sample quality during the generation process.
- The framework includes verifier models to evaluate generated sample quality.
- Algorithms are used to search for better noise candidates based on feedback.
- Higher quality results are achieved when models are given more time and compute.
9. Focus on inference time for future AI innovations.
π₯85
16:09
Investing time in understanding inference time scaling could lead to insights into the next innovations in AI technology.
- Inference time is a critical area for research and development.
- Learning about AI advancements in this area can provide a competitive edge.
- The potential for improved performance makes it a valuable focus for AI enthusiasts.