The COMPLETE TRUTH About AI Agents (2024)
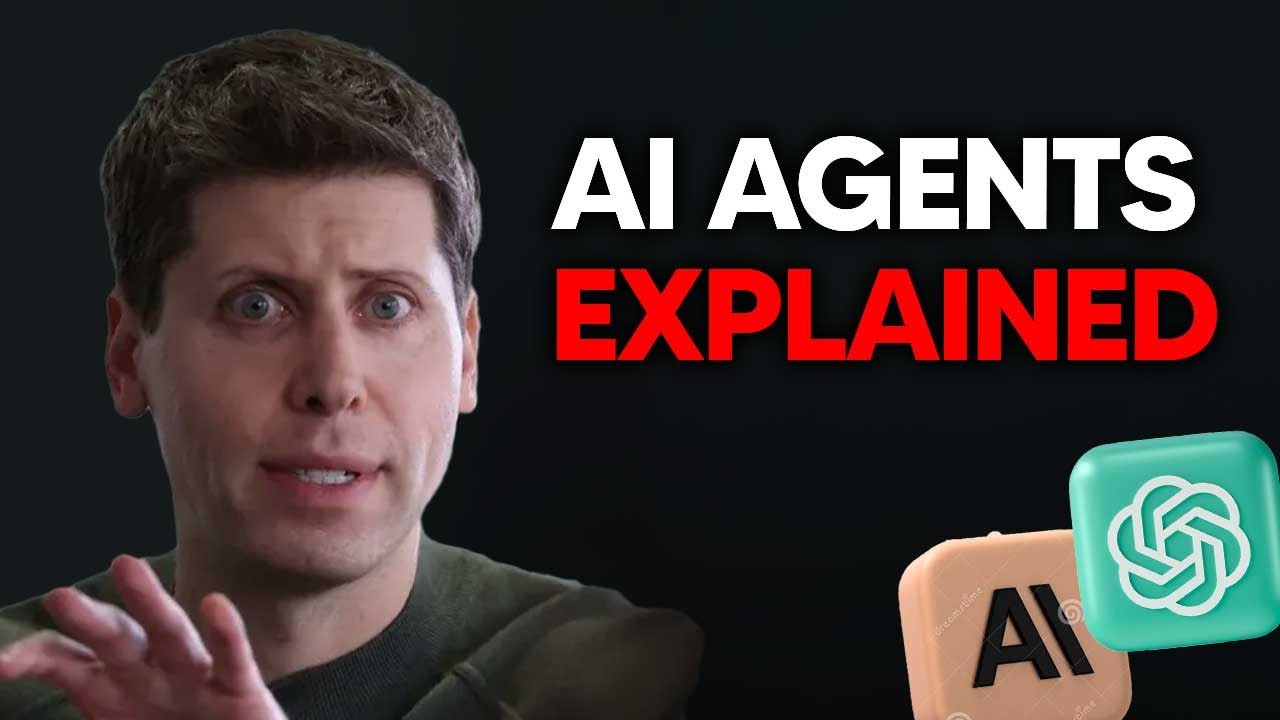
Key Takeaways at a Glance
00:00
AI agents are advanced AI assistants.03:06
Agentic workflows enhance AI performance significantly.05:10
Mixture of Agents paper showcases collaborative AI models.08:13
Open-source LLMS enhance AI capabilities.08:30
Crew AI enables collaborative AI teamwork.09:16
Real-world applications of AI agents are limited.09:46
Cassidy AI offers no-code agentic workflow creation.13:54
Multi-On offers basic AI tasks in browsers.02:12
Creating specialized GPTs for businesses unlocks significant value.16:27
AI agent devices like Rabbit AI face early criticisms and challenges.19:22
Google demonstrates effective customer service AI agents.21:14
Cognition Labs' Devin AI agent streamlines coding tasks.22:20
OpenAI explores agents controlling user devices for task automation.23:32
Meta develops engineering and monetization AI agents for diverse applications.24:59
IMVU focuses on reasoning-based coding agents for enhanced productivity.26:26
AI agents face fundamental hurdles in real-world tasks.27:01
Achieving reliable AI agents requires low error rates in individual actions.28:04
Future AI agents require significant computational scale for effective action.34:14
Bill Gates anticipates AI agents revolutionizing daily tasks in the near future.36:20
Nvidia envisions collaborative AI agents transforming the workplace.39:36
Mustafa Suan warns against fully autonomous AI agents due to potential risks.41:01
Agents rely on language models for interactions.41:36
Enhancing AI agents involves planning and user experience.44:21
Flow engineering is essential for optimizing agent performance.
1. AI agents are advanced AI assistants.
🥇92
00:00
AI agents are advanced AI assistants capable of autonomously executing tasks individually or in teams, enhancing productivity and efficiency.
- AI agents execute tasks autonomously based on instructions.
- They can work individually or collaboratively in teams.
- These agents enhance productivity and efficiency in various tasks.
2. Agentic workflows enhance AI performance significantly.
🥇96
03:06
Implementing agentic workflows dramatically improves AI output and reasoning abilities, surpassing non-agentic approaches like zero-shot prompting.
- Agentic workflows involve iterative processes for better results.
- Andrew Ng highlights the substantial improvement in AI performance with agentic workflows.
- Agentic workflows outperform non-agentic methods in coding benchmarks.
3. Mixture of Agents paper showcases collaborative AI models.
🥇94
05:10
The Mixture of Agents paper demonstrates the effectiveness of combining multiple AI models to refine responses iteratively, even with lower-quality auxiliary inputs.
- Utilizing different AI models in layers refines responses through collaboration.
- Open-source LLMS, when combined, outperform individual advanced models.
- Collaboration among AI models leads to substantial improvements in performance.
4. Open-source LLMS enhance AI capabilities.
🥇93
08:13
Leveraging open-source LLMS in agentic workflows significantly boosts AI capabilities, surpassing the performance of individual advanced models.
- Open-source LLMS contribute to improved AI performance through collaboration.
- Combining multiple LLMS enhances reasoning and output quality.
- Agentic workflows with open-source LLMS outperform standalone advanced models.
5. Crew AI enables collaborative AI teamwork.
🥈89
08:30
Crew AI facilitates collaborative working among AI agents, assigning specific roles to each agent for efficient task completion through teamwork and communication.
- Role-based agents in Crew AI enhance task efficiency.
- Agents communicate, share information, and assist each other in task completion.
- Crew AI streamlines complex task accomplishment through teamwork capabilities.
6. Real-world applications of AI agents are limited.
🥉79
09:16
Despite advancements, real-world applications of AI agents are currently limited, with few practical use cases available for widespread adoption.
- Current AI agent applications are limited in scope and practicality.
- Challenges exist in implementing AI agents for diverse real-world scenarios.
- Widespread adoption of AI agents in various industries is still in the early stages.
7. Cassidy AI offers no-code agentic workflow creation.
🥈88
09:46
Cassidy AI provides a user-friendly platform for creating instant no-code agentic workflows, allowing users to build and implement AI workflows easily using natural language prompts.
- No-code platform simplifies the creation of agentic workflows for non-technical users.
- Users can describe tasks in natural language to generate complex AI workflows.
- Cassidy AI enables quick and efficient development of AI workflows for various applications.
8. Multi-On offers basic AI tasks in browsers.
🥈82
13:54
Multi-On provides basic AI functionalities in browsers for simple tasks, showcasing the initial capabilities of autonomous AI agents.
- Multi-On allows users to perform basic tasks using AI directly in web browsers.
- These tasks are fundamental and represent the early stages of autonomous AI agent development.
- Multi-On serves as an entry point to experiencing AI capabilities in everyday browsing activities.
9. Creating specialized GPTs for businesses unlocks significant value.
🥇93
02:12
Tailoring custom GPTs for business applications using the Assistant API offers substantial value, enabling industrial-grade solutions and revenue streams.
- Business-focused GPTs provide enhanced value compared to generic consumer-oriented models available on the market.
- Specializing in AI agency solutions and adapting to industry demands ensures consistent revenue generation and client satisfaction.
- Utilizing the Assistant API empowers businesses to leverage AI capabilities for specific operational needs effectively.
10. AI agent devices like Rabbit AI face early criticisms and challenges.
🥈89
16:27
Devices integrating AI assistants, such as Rabbit AI, encounter initial scrutiny and struggle to meet high expectations, highlighting the complexities of AI hardware implementations.
- Rabbit AI device showcased diverse tasks but faced criticism for not meeting anticipated performance levels.
- Hardware-based AI agents like Rabbit AI demonstrate the intricate balance required for successful AI device deployment.
- Early feedback on AI agent devices emphasizes the need for continuous improvement and user-centric design.
11. Google demonstrates effective customer service AI agents.
🥇92
19:22
Google showcases AI agents providing real-time customer service assistance, illustrating the practical applications of AI in enhancing customer interactions.
- The demo highlights AI's role in streamlining customer inquiries and facilitating seamless transactions.
- Google's customer service agent exemplifies AI's potential in improving user experiences and operational efficiency.
- Real-time support through AI agents enhances customer engagement and satisfaction.
12. Cognition Labs' Devin AI agent streamlines coding tasks.
🥇94
21:14
Devin AI agent from Cognition Labs enhances coding workflows by leveraging GPT-4's cognitive capabilities, showcasing the future potential of AI in software development.
- Devin automates coding tasks, including project planning, debugging, and website deployment, mimicking human software engineering processes.
- The agent's autonomous capabilities demonstrate advancements in AI-driven software development tools.
- Devin's integration of reasoning and long-term planning signifies a significant leap in AI-assisted coding.
13. OpenAI explores agents controlling user devices for task automation.
🥈88
22:20
OpenAI delves into developing agents capable of automating complex tasks by controlling users' devices, showcasing the potential for AI to streamline various manual processes.
- Agents can perform tasks like data transfer, form filling, and report generation by emulating human interactions with different applications.
- The focus on device control highlights AI's role in enhancing productivity and efficiency in diverse operational scenarios.
- OpenAI's efforts aim to create agents that can effectively manage users' tasks and workflows autonomously.
14. Meta develops engineering and monetization AI agents for diverse applications.
🥈87
23:32
Meta works on AI agents for engineering tasks and monetization purposes, showcasing the versatility of AI in assisting businesses with coding and advertising.
- Engineering agents aid in software development tasks similar to GitHub's co-pilot, enhancing coding efficiency and accuracy.
- Monetization agents support businesses in advertising on Meta's platforms, offering internal and customer-facing solutions.
- Meta's AI agents cater to a wide range of applications, from technical tasks to revenue generation strategies.
15. IMVU focuses on reasoning-based coding agents for enhanced productivity.
🥇91
24:59
IMVU raises funds to develop AI agents focusing on reasoning and iterative processes to optimize coding tasks, signaling advancements in AI-driven software development.
- The emphasis on iterative processes mirrors human problem-solving approaches, enhancing the quality and efficiency of coding tasks.
- IMVU's agents aim to improve research, reflection, and decision-making in coding, leading to more effective and reliable software development.
- The funding indicates a commitment to enhancing AI agents' capabilities for complex coding challenges.
16. AI agents face fundamental hurdles in real-world tasks.
🥇96
26:26
Despite remarkable achievements, AI agents encounter significant challenges in executing diverse real-world tasks efficiently and reliably.
- Agents struggle with complex actions requiring multiple steps and adaptations to real-world outcomes.
- Interactions with the environment pose challenges for AI agents in decision-making and task completion.
- The gap between human-like reasoning and practical execution remains a significant obstacle for AI agents.
17. Achieving reliable AI agents requires low error rates in individual actions.
🥇92
27:01
For long sequences of tasks to work, each step must have minimal errors, necessitating models to become more reliable through scale and precision.
- Models need to improve reliability by reducing errors in each action.
- Scale and precision enhancements are crucial for achieving low error rates.
- Long-term success depends on minimizing errors in individual actions.
18. Future AI agents require significant computational scale for effective action.
🥈89
28:04
Advancements to GPT models necessitate two more generations of scale to enable agents to consistently perform tasks with precision.
- Future systems demand substantial computational scale for effective action.
- Enhancements in scale are vital for agents to operate effectively.
- Achieving precision in actions requires significant computational advancements.
19. Bill Gates anticipates AI agents revolutionizing daily tasks in the near future.
🥇96
34:14
In around 2.5 years, advanced AI agents will transform various aspects of life, offering services like mental therapy, friendship, and expertise.
- AI agents will revolutionize daily tasks and interactions within a few years.
- Future agents will provide diverse services like mental therapy and expert advice.
- Bill Gates envisions AI agents reshaping human-computer interactions.
20. Nvidia envisions collaborative AI agents transforming the workplace.
🥇94
36:20
Nvidia's CEO discusses a future where AI agents collaborate, assemble teams, and engage in multi-step reasoning, resembling human employees.
- AI agents will work collaboratively, resembling human employees.
- Future agents will engage in multi-step reasoning and complex scenarios.
- Nvidia foresees AI agents transforming workplace dynamics through collaboration.
21. Mustafa Suan warns against fully autonomous AI agents due to potential risks.
🥇93
39:36
Fully autonomous agents formulating plans and goals independently pose significant risks, emphasizing the need for limited autonomy to mitigate dangers.
- Complete autonomy in AI agents can lead to potential risks and dangers.
- Limited autonomy is crucial to prevent undesirable outcomes in AI agents.
- Mustafa Suan advocates for caution in developing fully autonomous AI agents.
22. Agents rely on language models for interactions.
🥇96
41:01
Agents use language models to engage with the external world through tools, memory, planning, and actions, enhancing their functionality and capabilities.
- Language models interact with the external world through various forms.
- Tools, memory, planning, and actions are fundamental components of agent interactions.
- Agents run language models in loops to execute tasks and make decisions.
23. Enhancing AI agents involves planning and user experience.
🥇93
41:36
Improving AI agents requires focusing on planning, user experience, and memory capabilities to create more efficient and effective workflows.
- Developers are investing time in enhancing agent planning and user interface design.
- Memory incorporation in agents is crucial for future advancements in AI workflows.
- New workflows like Alpha Codium aim to optimize coding tasks through improved agent capabilities.
24. Flow engineering is essential for optimizing agent performance.
🥇94
44:21
Optimizing agent performance involves designing efficient flow structures to enhance coding capabilities beyond model improvements.
- Alpha Codium emphasizes flow engineering over model enhancements for coding efficiency.
- Human engineers play a critical role in planning the workflow for AI agents.
- Efficient flow design leads to more effective and advanced AI capabilities.