The Industry Reacts to Llama 4 - "Nearly INFINITE"
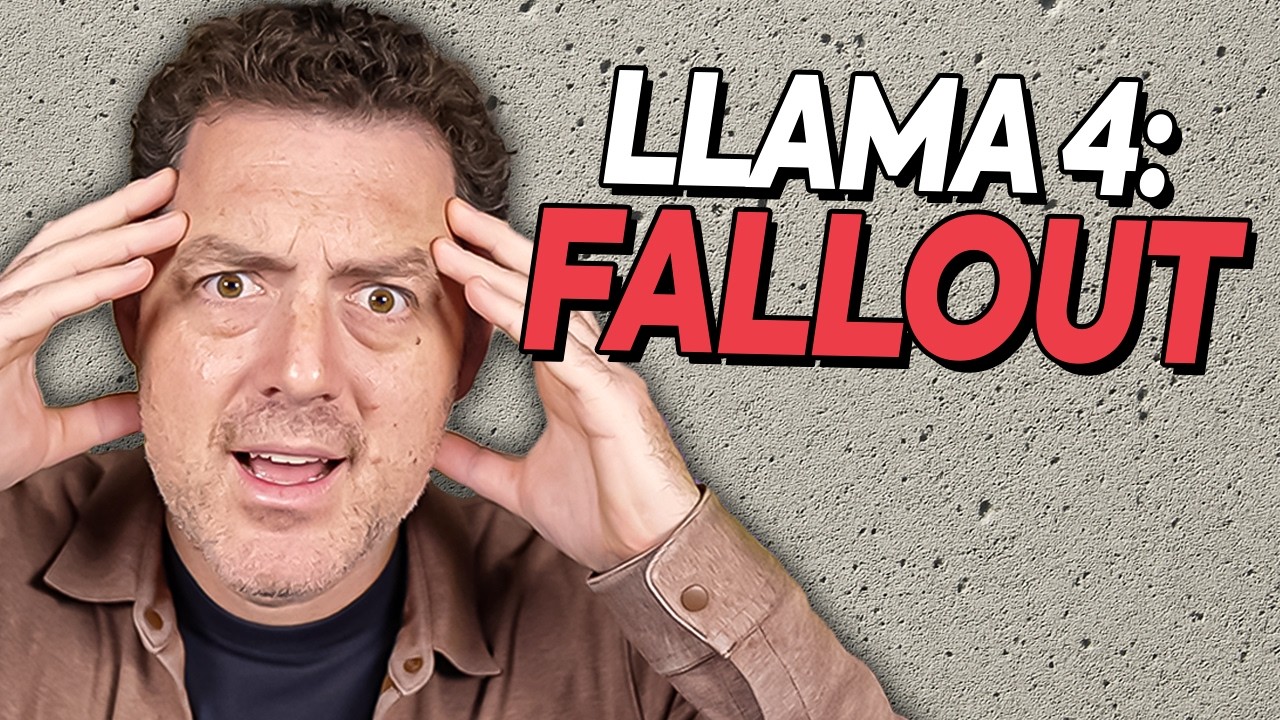
Key Takeaways at a Glance
00:04
Llama 4's release was strategically timed.01:00
Llama 4 models show impressive performance metrics.05:26
Llama 4 is highly efficient and cost-effective.07:45
Industry leaders are optimistic about Llama 4's impact.09:30
Llama 4's context capabilities are groundbreaking.14:44
Llama 4's performance is influenced by active parameters.16:26
The claimed 10 million token context window is questionable.17:11
Llama 4's coding capabilities are under scrutiny.
1. Llama 4's release was strategically timed.
🥈88
00:04
Meta advanced the release of Llama 4 to potentially outpace competitors launching similar models, indicating a competitive landscape in AI.
- The original release date was moved from April 7th to April 5th.
- This suggests awareness of other upcoming model releases.
- The AI industry is closely interconnected, with companies monitoring each other's moves.
2. Llama 4 models show impressive performance metrics.
🥇92
01:00
Independent evaluations reveal that Llama 4's Maverick model outperforms notable competitors like Claude 3.7 Sonnet, showcasing its capabilities.
- Maverick scored 49 in the artificial analysis intelligence index, surpassing Claude 3.7 Sonnet.
- The model's efficiency is highlighted by its lower active parameters compared to competitors.
- Scout, the smaller version, is competitive with GPT-4 Mini.
3. Llama 4 is highly efficient and cost-effective.
🥇95
05:26
Llama 4 models are designed to deliver high performance at a lower operational cost compared to other models in the market.
- Maverick operates at 15 cents per million input tokens, significantly cheaper than competitors.
- The model achieves comparable performance with fewer active parameters.
- This efficiency positions Llama 4 favorably for widespread adoption.
4. Industry leaders are optimistic about Llama 4's impact.
🥇90
07:45
Prominent figures in the tech industry have praised Llama 4, indicating its potential to reshape the AI landscape.
- Satia Nadella emphasized Azure's role in hosting Llama 4 models.
- Michael Dell announced availability on the Dell Enterprise Hub.
- David Saxs highlighted the importance of open-source models in the AI race.
5. Llama 4's context capabilities are groundbreaking.
🥈87
09:30
The model's ability to handle a near-infinite context is seen as a game-changer for various applications.
- Meta claims Llama 4 can manage over 10 million tokens, enhancing its usability.
- This feature allows for processing extensive data inputs like books and movies.
- However, some experts caution that traditional retrieval-augmented generation (RAG) methods may still be more efficient.
6. Llama 4's performance is influenced by active parameters.
🥈88
14:44
Llama 4 models utilize a sparse mixture of experts, activating only a small number of parameters during token generation, which affects performance.
- Despite having a massive number of parameters, only a few are active at any time.
- This design allows for efficient operation even with lower performance metrics.
- The M3 Ultra Max Studios model showcases this with significant memory capabilities.
7. The claimed 10 million token context window is questionable.
🥈85
16:26
Experts suggest that Llama 4's 10 million token context window is virtual, as no training was done on prompts longer than 256 tokens.
- Sending more than 256K tokens often results in low-quality outputs.
- The largest model, Behemoth, has two trillion parameters but lacks reasoning capabilities.
- Future updates may improve reasoning performance.
8. Llama 4's coding capabilities are under scrutiny.
🥈80
17:11
Initial skepticism about Llama 4's coding skills has been noted, especially in comparison to other models like GPT-4.
- Tests showed Llama 4 struggling with complex tasks, such as simulating a bouncing ball in a hexagon.
- Comparisons with Gemini 2.5 Pro and GPT-4 indicate Llama 4's performance is lacking.
- The open-source nature of Llama 4 suggests potential for improvement.