V-JEPA: Revisiting Feature Prediction for Learning Visual Representations from Video (Explained)
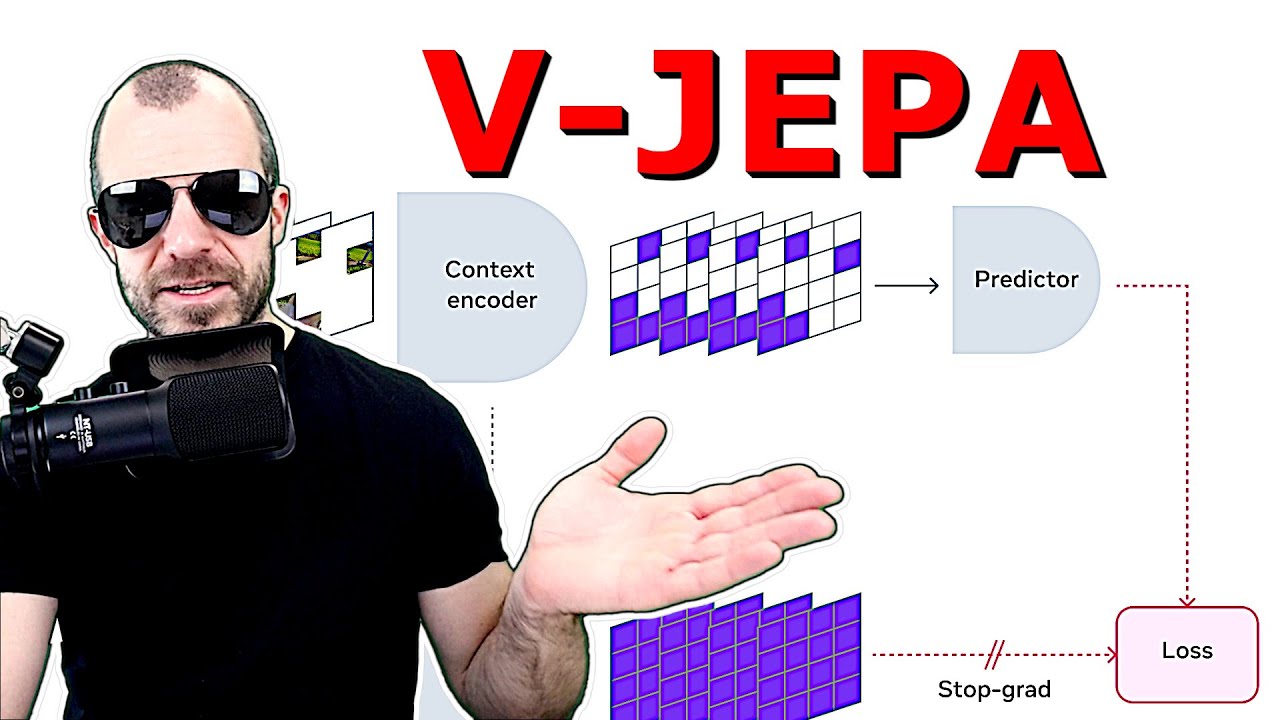
Key Takeaways at a Glance
00:00
Feature prediction is a powerful tool for unsupervised learning.05:24
V-JEPA focuses on unsupervised learning through feature prediction.05:54
Importance of latent space in feature prediction models.10:20
Energy function aids in determining data point compatibility.14:12
Training models with clean and crisp losses enhances performance.18:17
Preventing model collapse is crucial in unsupervised feature learning.27:33
Feature prediction can be a powerful objective for unsupervised learning from video.33:23
V-JEPA architecture involves patching video frames into tokens.44:48
Feature space prediction outperforms pixel space prediction in V-JEPA.46:30
Qualitative evaluation via decoding validates V-JEPA's learned features.49:45
Understanding unsupervised learning principles is crucial.
1. Feature prediction is a powerful tool for unsupervised learning.
π₯96
00:00
Feature prediction allows learning latent features from video data, enhancing downstream tasks like video classification.
- Humans excel at mapping low-level signals to semantic understanding.
- V-JEPA leverages the predictive feature principle for unsupervised learning.
- Learning latent features aids in recognizing objects and global motion from video data.
2. V-JEPA focuses on unsupervised learning through feature prediction.
π₯93
05:24
The model emphasizes learning visual representations solely through feature prediction without external aids like pre-trained encoders or human annotations.
- Avoids using pre-trained image encoders, negative examples, or human annotations.
- Efficiently learns visual representations without pixel-level reconstruction.
- Prioritizes feature prediction over traditional pixel-based methods for efficiency.
3. Importance of latent space in feature prediction models.
π₯94
05:54
V-JEPA operates in a latent space, focusing on representations rather than raw signals, enhancing efficiency and effectiveness.
- Operations in latent space enable more extensive budget allocation for feature correctness.
- Avoids pixel reconstruction errors by working solely in the latent space.
- Latent space embeddings facilitate robust feature learning and prediction.
4. Energy function aids in determining data point compatibility.
π₯92
10:20
Systems like V-JEPA use an energy function to assess the compatibility of different parts of data points, aiding in recognizing valid continuations.
- Energy function helps in determining if two data parts are coherent.
- Z variable encapsulates the relationship between data points for accurate predictions.
- Energy function ensures systems recognize valid continuations in data sequences.
5. Training models with clean and crisp losses enhances performance.
π₯89
14:12
Ensuring loss functions are specific to individual choices rather than averaged over all possibilities leads to sharper and more accurate model training.
- Avoiding blurred outcomes by training models with distinct losses for specific choices.
- Preventing loss overlap by focusing on clean and crisp losses for each choice.
- Enhancing model robustness by accounting for specific choices in training losses.
6. Preventing model collapse is crucial in unsupervised feature learning.
π₯96
18:17
Avoiding collapse in unsupervised feature learning is essential to ensure the model does not output constant values, leading to ineffective representations.
- Model collapse occurs when the model outputs constant values due to lack of diverse training examples.
- Strategies like incorporating choice variables and moving averages help prevent collapse and encourage meaningful learning.
- Maintaining a balance between similarity and diversity in representations is key to effective unsupervised learning.
7. Feature prediction can be a powerful objective for unsupervised learning from video.
π₯93
27:33
Utilizing feature prediction as an objective in unsupervised learning from video can lead to versatile visual representations and faster training compared to pixel prediction methods.
- Feature prediction enables the extraction of useful features for downstream tasks or fine-tuning.
- It offers superior efficiency and label utilization compared to pixel-based approaches.
- The approach involves predicting representations of masked video segments rather than pixel-level details.
8. V-JEPA architecture involves patching video frames into tokens.
π₯92
33:23
Video frames are split into 16x16 pixel patches, forming tokens for processing, enabling a language-like treatment for visual data.
- Tokens represent spatial-temporal patches in the video.
- Unrolling patches creates a sequence for language-based processing.
- Continuous masking of regions challenges prediction tasks.
9. Feature space prediction outperforms pixel space prediction in V-JEPA.
π₯89
44:48
Predicting hidden representations yields better performance for downstream tasks compared to pixel-based methods, enhancing label efficiency.
- Evaluation shows improved performance and label efficiency.
- Data mixing and masking strategies impact model performance.
- V-JEPA's feature prediction approach reduces required samples significantly.
10. Qualitative evaluation via decoding validates V-JEPA's learned features.
π₯88
46:30
Decoding masked regions using learned representations showcases the model's ability to reconstruct video content accurately, despite boundary artifacts.
- Decoder reconstructs video content based on predicted latent representations.
- Evaluation focuses on the arrangement and objects in the video.
- Boundary imperfections are expected in this evaluation method.
11. Understanding unsupervised learning principles is crucial.
π₯85
49:45
Grasping the principles behind unsupervised learning is essential for effective implementation.
- Direction unsupervised learning is a cool concept to explore.
- Remaining informed about unsupervised learning is highly valuable.