xLSTM: Extended Long Short-Term Memory
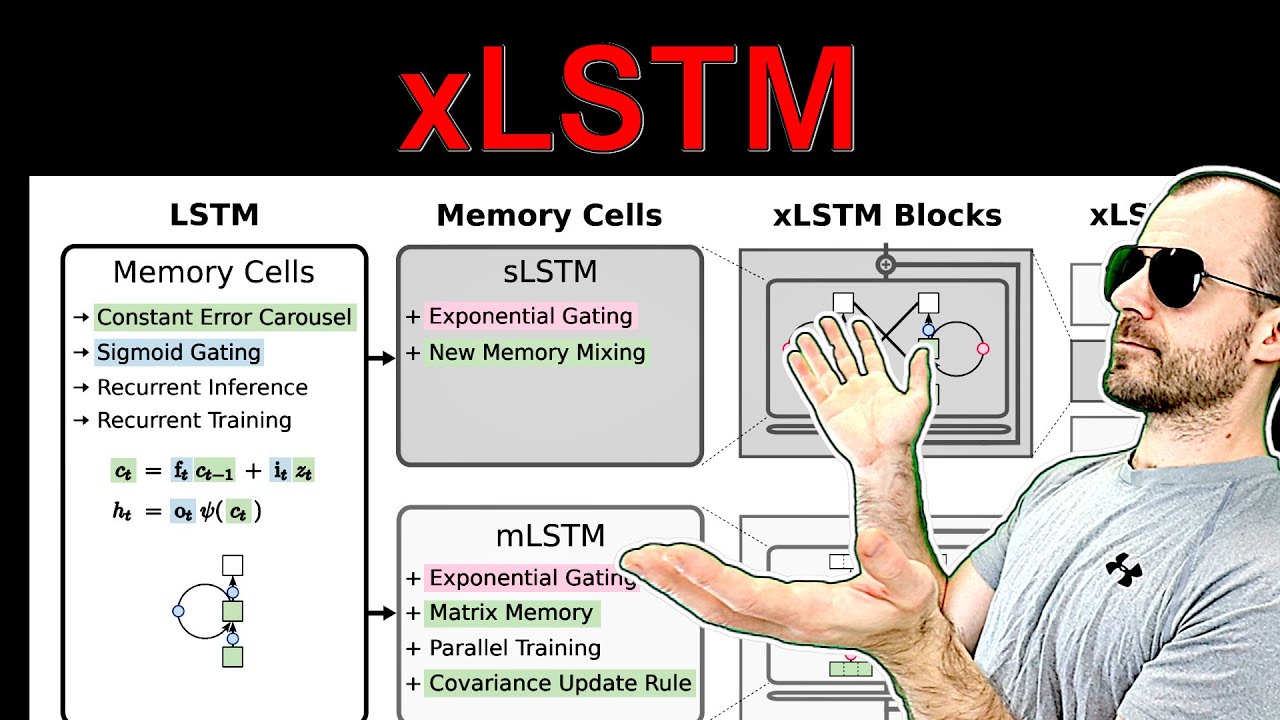
Key Takeaways at a Glance
00:00
xLSTM aims to enhance LSTM models with modern learnings.02:12
LSTMs have been pivotal in various domains despite limitations.07:37
xLSTM introduces innovative modifications to traditional LSTM structures.11:49
Comparing LSTM and Transformer mechanisms reveals distinct advantages.18:04
Understanding the role of C and H in xLSTM is crucial.18:32
Significance of gates in extending LSTMs without gradient issues.27:41
Transition from scalar to vector operations enhances memory mixing.31:41
Replacing sigmoid with exponential functions aids gradient stability.35:35
xLSTM introduces new nonlinearity and memory extension techniques.41:47
xLSTM's memory handling strategy offers computational advantages.42:59
Parallelizable linear operations in xLSTM facilitate efficient training.47:19
xLSTM integrates Transformer-like elements for enhanced functionality.51:36
xLSTM shows competitive performance in language modeling.54:27
xLSTM limitations include computational costs and training speed.
1. xLSTM aims to enhance LSTM models with modern learnings.
🥈88
00:00
The paper explores advancing LSTM models with insights from modern Transformer architectures, aiming to improve performance in language modeling.
- Integrating knowledge from Transformer models into LSTM architectures.
- Seeking to compete with attention-based models in language tasks.
- Highlighting the evolution of neural network architectures over time.
2. LSTMs have been pivotal in various domains despite limitations.
🥈82
02:12
LSTMs have been widely used due to their effectiveness in processing sequences like time series and audio data, despite facing challenges compared to attention mechanisms.
- Significant impact of LSTMs in machine translation and language modeling.
- Efficient processing of sequences that Transformers may struggle with.
- Resource-efficient application of LSTMs in diverse fields.
3. xLSTM introduces innovative modifications to traditional LSTM structures.
🥇93
07:37
The paper presents novel modifications like exponential gating and matrix memory to enhance LSTM capabilities, focusing on memory capacity and parallel training.
- Incorporating exponential gating and matrix memory for improved performance.
- Enhancing memory capacity through associative memory usage.
- Enabling parallel training similar to attention mechanisms.
4. Comparing LSTM and Transformer mechanisms reveals distinct advantages.
🥈87
11:49
Contrasting LSTM's sequential processing with Transformer's attention mechanism showcases trade-offs in memory usage and optimization complexity.
- Highlighting the benefits of LSTM's constant memory usage.
- Exploring the challenges of information extraction and representation in LSTM.
- Discussing the evolution from RNNs to attention mechanisms in neural networks.
5. Understanding the role of C and H in xLSTM is crucial.
🥇92
18:04
C and H represent different parts of the hidden state in xLSTM, with C being significant for memory mixing and H for output computation.
- C and H are both components of the hidden state in xLSTM.
- C is involved in memory mixing through recurrent connections.
- H is computed for output generation in each time step.
6. Significance of gates in extending LSTMs without gradient issues.
🥈89
18:32
Gating functions in xLSTM prevent problems like exploding gradients, enabling efficient training by controlling memory retention and input incorporation.
- Gates like forget and input gates regulate memory retention and input relevance.
- Additive updating through gating functions mitigates gradient problems in xLSTM.
- Nonlinearities in gates ensure controlled information flow.
7. Transition from scalar to vector operations enhances memory mixing.
🥇94
27:41
Moving from scalar to vector operations in xLSTM allows for memory mixing through matrix operations, improving information exchange between dimensions.
- Vector gates enable elementwise multiplication for enhanced information processing.
- Block diagonal matrices facilitate multi-head memory mixing for diverse information interactions.
- Utilizing matrices enhances information flow across dimensions for improved memory handling.
8. Replacing sigmoid with exponential functions aids gradient stability.
🥈88
31:41
Substituting sigmoid with exponential functions in xLSTM helps prevent vanishing gradients, with normalization aiding in maintaining signal integrity.
- Exponential functions offer non-saturating properties for gradient stability.
- Normalization through division by accumulated values ensures signal normalization.
- Linearizing computations and preventing saturation contribute to gradient stability.
9. xLSTM introduces new nonlinearity and memory extension techniques.
🥇92
35:35
xLSTM incorporates novel nonlinearity and memory extension methods, enhancing memory capacity and computational efficiency.
- New nonlinearity and memory extension improve memory handling.
- Techniques like block diagonal matrices enhance memory storage and retrieval.
- Memory capacity is increased without introducing additional parameters.
10. xLSTM's memory handling strategy offers computational advantages.
🥈87
41:47
The memory management approach in xLSTM provides computational benefits through linear operations and memory organization.
- Linear operations in memory management enhance computational efficiency.
- Optimized memory organization contributes to computational advantages.
- Efficient memory handling leads to improved computational performance.
11. Parallelizable linear operations in xLSTM facilitate efficient training.
🥈89
42:59
xLSTM's linear operations enable parallel processing, enhancing training speed and scalability.
- Linear operations allow for efficient parallelization during training.
- Absence of nonlinear dependencies between time steps aids in parallel processing.
- Linear computations contribute to faster and scalable training processes.
12. xLSTM integrates Transformer-like elements for enhanced functionality.
🥈85
47:19
By incorporating Transformer-like components, xLSTM enhances functionality and performance in memory processing.
- Integration of Transformer elements improves memory processing capabilities.
- Transformer-inspired features elevate the functionality of xLSTM.
- Transformer components contribute to enhanced memory operations.
13. xLSTM shows competitive performance in language modeling.
🥈88
51:36
xLSTM competes well with similar models in general language modeling tasks, especially where recurrent neural networks are beneficial.
- Competitive performance in tasks where recurrency is advantageous.
- Comparable performance to similar models in general language modeling.
- Evaluation results indicate competitive standing in the field.
14. xLSTM limitations include computational costs and training speed.
🥈82
54:27
Challenges include expensive computational load for large language experiments and limitations in fast parallel training due to recurrency.
- Computational costs increase with larger memory sizes.
- Inability to optimize fully for larger xLSTM architectures.
- Development of fast CUDA kernel to address training challenges.