100 Billion Humanoid Robots, New AI Agent, GPT-5 Not That Good?
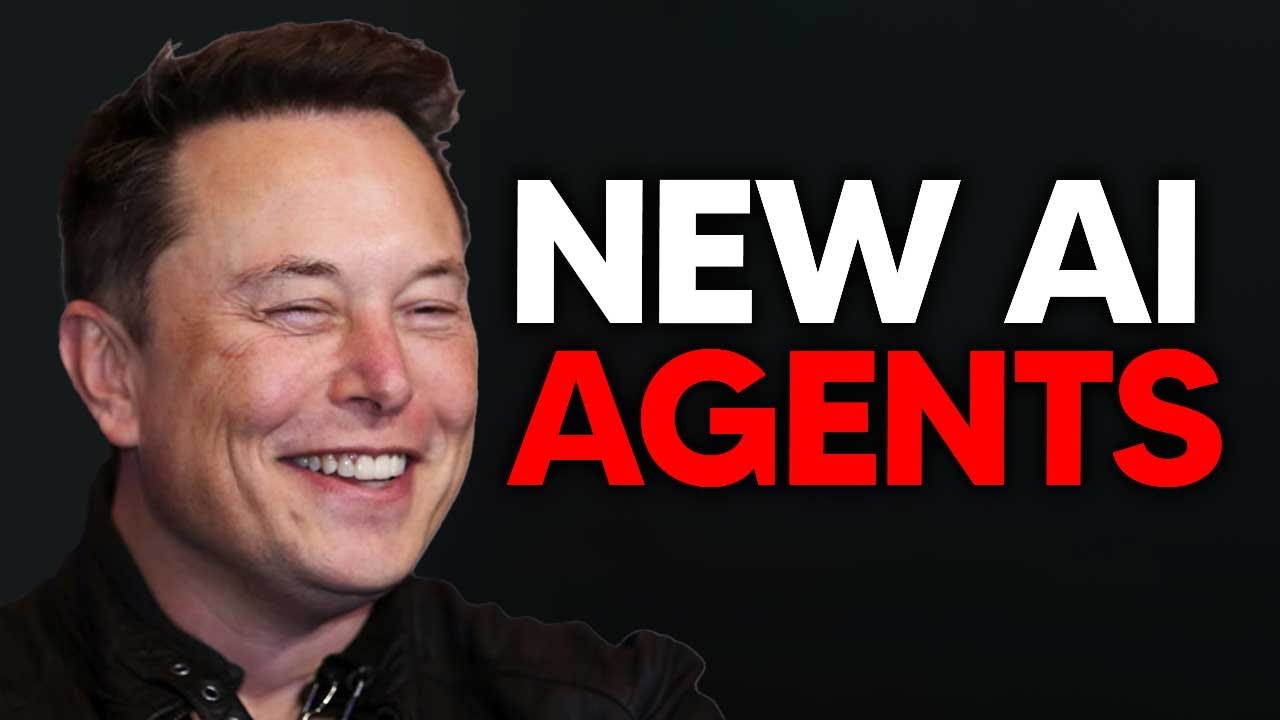
Key Takeaways at a Glance
00:00
Humanoid robots are being trained to imitate human actions autonomously.03:06
Challenges exist due to limited degrees of freedom in current humanoid robots.03:18
Teleoperation data capture innovation is essential for training autonomous robots.05:46
Future advancements aim for autonomous skills on more advanced hardware platforms.06:43
Elon Musk envisions mass production of humanoid robots for various tasks.13:14
Potential of AI agents like Jace in automating business processes.14:08
Implications of GPT-5's potential advancements in AI capabilities.24:01
Integration of multiple AI models to surpass existing benchmarks.26:33
GPTs use a multi-layered approach for generating responses.26:49
Synthesizers aggregate responses from different AI models.27:11
Iterative refinement process enhances GPT performance.
1. Humanoid robots are being trained to imitate human actions autonomously.
🥇92
00:00
Stanford University collaborates with Google Deep Mind to train humanoid robots using an RGB camera to mimic human movements for autonomous task performance.
- Robots observe and mimic human movements to collect data for autonomous task execution.
- Advanced pose estimation algorithms are used to collect human motion data.
- Training involves shadowing human actions using policies trained in a simulation environment.
2. Challenges exist due to limited degrees of freedom in current humanoid robots.
🥈87
03:06
Current humanoid robots lack the range of motion humans possess, making certain tasks challenging due to their rigid nature.
- The unitary robot used has restrictions in performing tasks requiring extensive flexibility.
- Despite limitations, effective policies are developed through reinforcement learning for task execution.
3. Teleoperation data capture innovation is essential for training autonomous robots.
🥈89
03:18
Innovative methods are being developed to capture teleoperation data effectively for training robots, overcoming challenges in traditional teleoperation.
- New approaches are being explored to gather data efficiently for training robots.
- Low-level policies are trained using reinforcement learning on diverse human motion data sets.
4. Future advancements aim for autonomous skills on more advanced hardware platforms.
🥈88
05:46
Future developments target training autonomous skills on advanced hardware platforms like Unit's newest robot to enhance capabilities and flexibility.
- Exploration of training policies on smoother, more flexible robots for improved task performance.
- Anticipating advancements in robotics for enhanced research capabilities and broader applications.
5. Elon Musk envisions mass production of humanoid robots for various tasks.
🥇93
06:43
Elon Musk discusses plans for producing 100 million humanoid robots annually to perform diverse tasks, envisioning a future where robots are ubiquitous in various roles.
- Ambitious goals set for humanoid robots to undertake tasks ranging from household chores to industrial functions.
- Speculation on the potential impact of advanced humanoid robots on society and industries.
6. Potential of AI agents like Jace in automating business processes.
🥇92
13:14
AI agents like Jace showcase the potential to automate tasks like scheduling interviews, managing hiring processes, and even creating companies with simple prompts.
- Jace demonstrates capabilities in handling complex tasks like creating business plans and generating revenue.
- Despite limitations in handling complicated tasks and browsing speed, advancements are being made to enhance performance.
- AI agents like Jace hint at the future potential for automating various business operations.
7. Implications of GPT-5's potential advancements in AI capabilities.
🥈89
14:08
Anticipation for GPT-5's ability to excel in multi-step reasoning and planning tasks, potentially revolutionizing AI capabilities.
- Expectations for GPT-5 to overcome current limitations in AI agents related to planning and reasoning.
- Forecasts suggest that upcoming AI models could significantly enhance capabilities beyond existing frameworks like GPT-4.
- GPT-5's release could mark a significant advancement in AI technology, impacting various industries.
8. Integration of multiple AI models to surpass existing benchmarks.
🥇94
24:01
Utilizing a mixture of open-source AI models to surpass GPT-4's performance on challenging benchmarks.
- Combining the strengths of various AI models to achieve superior performance in tasks.
- Demonstrating the effectiveness of collaborative AI approaches in pushing the boundaries of AI capabilities.
- Highlighting the potential for innovative frameworks to enhance AI model performance significantly.
9. GPTs use a multi-layered approach for generating responses.
🥇92
26:33
GPTs utilize multiple layers of AI models to synthesize various responses into a single high-quality output.
- Responses from different models are combined to enhance the quality of the final output.
- The iterative process of refining answers through multiple layers improves response accuracy.
- This multi-layered approach enables GPTs to outperform previous benchmarks.
10. Synthesizers aggregate responses from different AI models.
🥈88
26:49
Synthesizers combine answers from various AI models to create a comprehensive and enriched response.
- The synthesizer acts as an aggregator, merging inputs from different models into a cohesive output.
- This aggregation process enhances the quality and depth of the final response.
- It allows for the consolidation of diverse perspectives into a unified answer.
11. Iterative refinement process enhances GPT performance.
🥇94
27:11
GPTs undergo iterative refinement where each layer improves upon the previous one, leading to superior performance.
- Successive layers analyze and enhance responses from preceding layers, optimizing the final output.
- This iterative process enables GPTs to surpass older models like GPT-40 in benchmark tests.
- Continuous refinement through layers ensures the generation of high-quality responses.