AI Conquers Gravity: Robo-dog, Groomed by GPT-4, Stays Balanced on Rolling, Deflating Yoga Ball
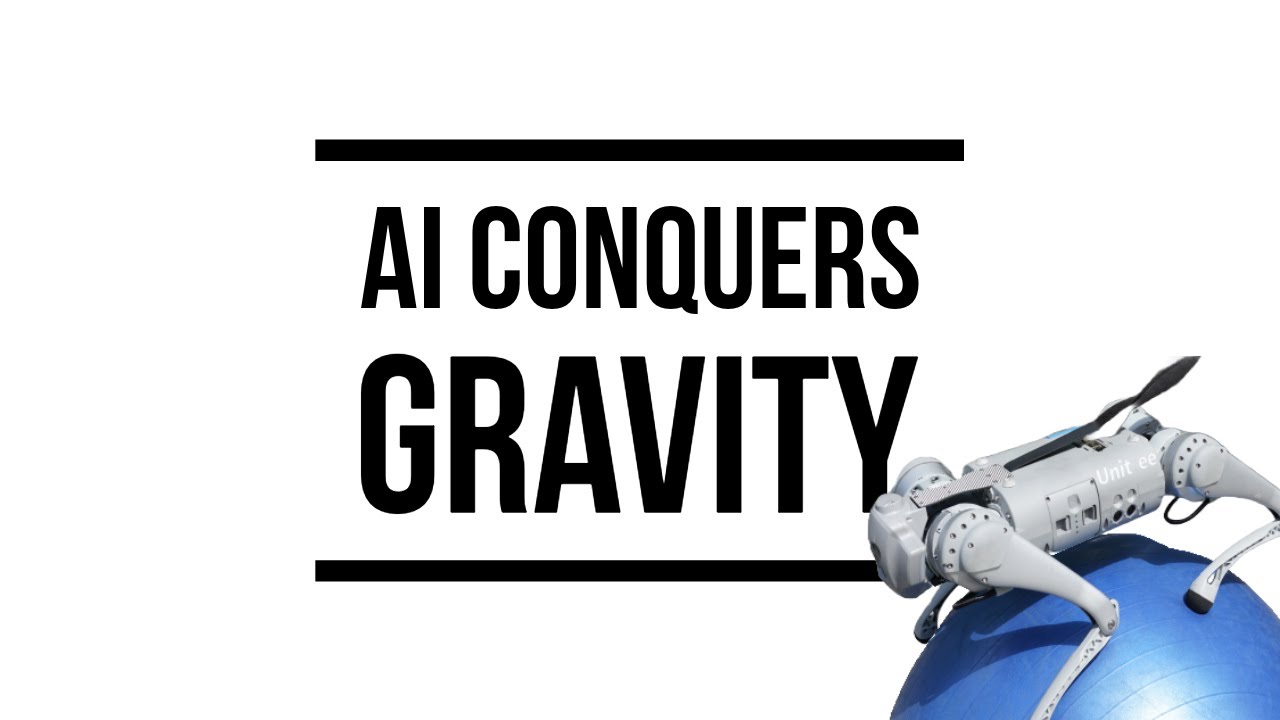
Key Takeaways at a Glance
00:46
GPT-4 guides Robo-dog training from simulation to reality.01:28
Language models like GPT-4 enhance robot training efficiency.05:06
Domain randomization optimizes robot training.09:53
Safety instructions are crucial for GPT-4's training accuracy.12:00
GPT-4's iterative learning surpasses human training methods.12:45
Real-world feedback integration can further enhance simulation to reality transfer.15:21
AI advancements may impact various job sectors.15:46
AI-driven dexterity could redefine human capabilities.
1. GPT-4 guides Robo-dog training from simulation to reality.
π₯92
00:46
Using GPT-4 to train a Robo-dog in simulation and transfer skills to the real world showcases the Sim to real concept.
- Language models like GPT-4 guide the training process from simulation to real-world applications.
- Simulation training with GPT-4 enables efficient and effective skill transfer to physical robots.
- GPT-4's role in training Robo-dogs highlights the potential of language models in robotics.
2. Language models like GPT-4 enhance robot training efficiency.
π₯89
01:28
GPT-4's language model-derived reward functions outperform human ones, aiding in better robot training.
- GPT-4's reward functions perform better than human-designed ones for training robots.
- Language models like GPT-4 excel in generating and refining reward functions for robot learning.
- GPT-4's patient and iterative approach leads to improved robot training outcomes.
3. Domain randomization optimizes robot training.
π₯87
05:06
Domain randomization based on common sense improves the effectiveness of robot training by setting realistic variable ranges.
- Domain randomization provides realistic variable ranges for effective robot training.
- GPT-4's domain randomization enhances learning by setting practical and relevant parameter ranges.
- Realistic domain randomization parameters lead to more reliable robot training outcomes.
4. Safety instructions are crucial for GPT-4's training accuracy.
π₯88
09:53
Incorporating safety instructions ensures GPT-4's policies are stable and realistic for successful robot training.
- Safety-oriented prompts guide GPT-4 in generating stable and safe robot training policies.
- Safety instructions prevent degenerate behaviors and ensure policies are suitable for real-world deployment.
- GPT-4's adherence to safety instructions enhances the reliability of robot training outcomes.
5. GPT-4's iterative learning surpasses human training methods.
π₯86
12:00
GPT-4's ability to continuously improve reward functions and policies outperforms human-designed training curricula.
- GPT-4's iterative learning approach eliminates the need for predefined training curricula.
- GPT-4's self-teaching capability enhances robot training outcomes beyond human-designed methods.
- Continuous improvement through iterative learning sets GPT-4 apart in training robots.
6. Real-world feedback integration can further enhance simulation to reality transfer.
π₯84
12:45
Dynamic adjustment of domain randomization parameters based on real-world feedback could improve the transferability of simulation-trained skills.
- Incorporating real-world feedback for adjusting domain randomization parameters can enhance skill transfer.
- Dynamic parameter adjustments based on performance feedback could optimize simulation to reality transfer in robot training.
- Further improvements in simulation to reality transferability can be achieved through real-world feedback integration.
7. AI advancements may impact various job sectors.
π₯88
15:21
Mass production of robots could lead to significant job disruptions, especially in high-stakes fields like self-driving vehicles.
- Plumbers and other professions might face challenges due to AI advancements.
- Sanctuary AI's developments could revolutionize tasks currently performed by humans.
- AI training through simulations could rapidly transform industries.
8. AI-driven dexterity could redefine human capabilities.
π₯85
15:46
AI's ability to mimic human finger dexterity might become a key differentiator, with Sanctuary AI leading the way.
- Sanctuary AI's advancements in training and simulations could enhance human-like capabilities.
- AI's potential to excel in tasks requiring intricate finger movements is noteworthy.