GPT-4o Mini Arrives In Global IT Outage, But How βMiniβ Is Its Intelligence?
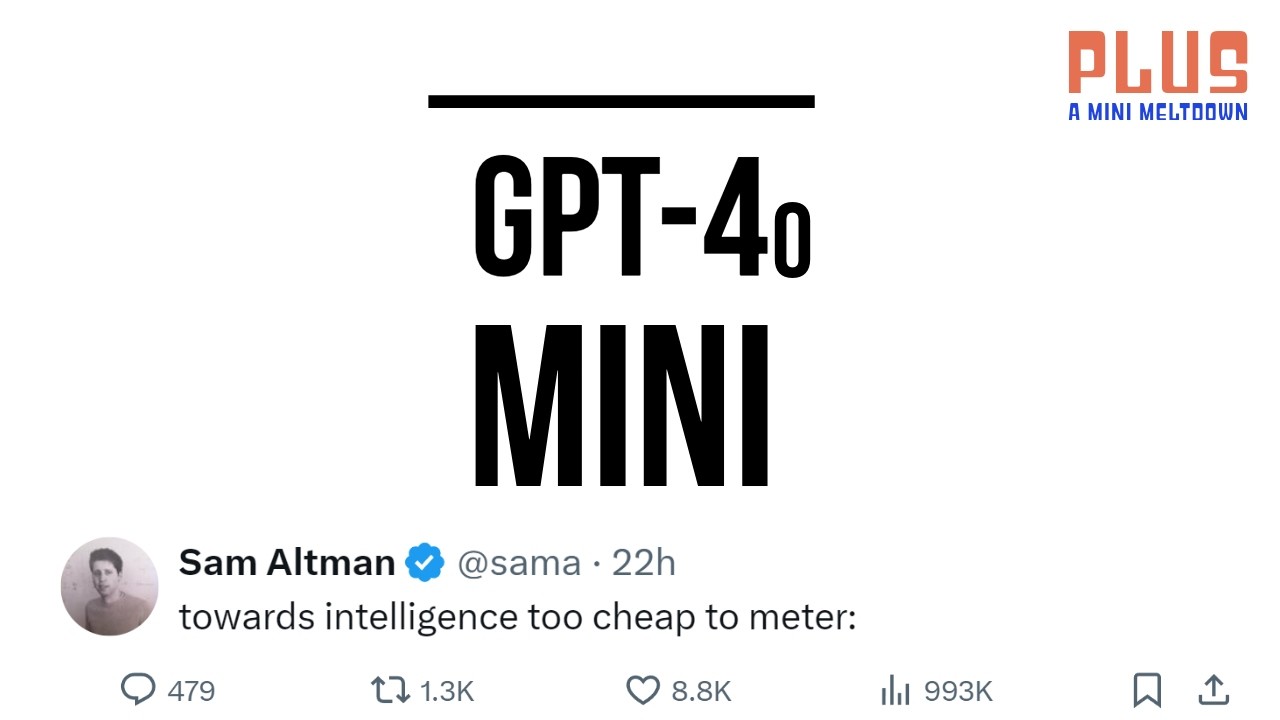
Key Takeaways at a Glance
00:18
GPT-4o Mini offers superior intelligence at a lower cost.02:02
GPT-4o Mini's capabilities are currently limited to text and vision.04:27
Benchmarks may not fully capture a model's capabilities.05:39
Models may excel in specific tasks but lack broader understanding.09:19
Current AI models lack real-world grounding and embodied intelligence.12:05
Models' reliance on textual data hinders their ability to grasp real-world nuances.15:29
Real-world data crucial for enhancing AI models.16:43
AI models' limitations in handling complex scenarios.
1. GPT-4o Mini offers superior intelligence at a lower cost.
π₯92
00:18
OpenAI's GPT-4o Mini provides enhanced intelligence at a reduced cost compared to similar models like Google's Gemini 1.5 Flash.
- GPT-4o Mini outperforms competitors like Gemini 1.5 Flash on the MMLU Benchmark while being more cost-effective.
- The model showcases improved performance metrics, indicating advancements in AI capabilities.
- Lower costs and higher scores make GPT-4o Mini an attractive option for users.
2. GPT-4o Mini's capabilities are currently limited to text and vision.
π₯88
02:02
Despite its name, GPT-4o Mini currently supports only text and vision, with audio capabilities expected in the future.
- The model lacks video and audio support, limiting its functionality compared to broader AI models.
- Future updates are anticipated to include audio inputs and outputs, expanding its utility.
- GPT-4o Mini's current focus on text and vision may restrict its application in certain scenarios.
3. Benchmarks may not fully capture a model's capabilities.
π₯87
04:27
Relying solely on benchmarks like MMLU may overlook crucial aspects of AI models, such as common sense reasoning.
- Models optimizing for specific benchmarks may neglect essential skills like common sense reasoning.
- Prioritizing benchmark performance can lead to trade-offs in other critical areas of AI functionality.
- The limitations of benchmark evaluations highlight the need for a more comprehensive assessment of AI models.
4. Models may excel in specific tasks but lack broader understanding.
π₯89
05:39
AI models trained extensively on specific tasks may struggle with real-world scenarios requiring common sense or contextual understanding.
- Specialized training can lead to proficiency in narrow domains but hinder performance in diverse contexts.
- Models like Gemini 1.5 Flash may excel in certain areas but falter when faced with unconventional challenges.
- The challenge lies in balancing task-specific expertise with general intelligence.
5. Current AI models lack real-world grounding and embodied intelligence.
π₯86
09:19
AI models primarily rely on textual data and lack the ability to understand the physical world and object interactions.
- Models focus on text prediction rather than real-world comprehension, limiting their practical applicability.
- Efforts are underway to imbue AI models with physical intelligence to enhance their understanding of real-world dynamics.
- The disconnect between textual intelligence and real-world knowledge poses challenges for AI advancement.
6. Models' reliance on textual data hinders their ability to grasp real-world nuances.
π₯85
12:05
AI models' dependence on textual information restricts their capacity to comprehend real-world complexities accurately.
- Text-based training limits models' understanding of spatial, social, and physical interactions.
- Models struggle to navigate scenarios that require contextual understanding beyond textual prompts.
- Enhancing models with real-world data is crucial to bridge the gap between textual intelligence and practical knowledge.
7. Real-world data crucial for enhancing AI models.
π₯92
15:29
Grounding AI models in real-world data significantly improves their performance and mitigates errors and hallucinations.
- Models trained on text alone can make mistakes and hallucinate.
- Simulations based on real-world data can provide more accurate and reliable answers.
- AI models need to be trained on diverse data to handle complex real-world scenarios.
8. AI models' limitations in handling complex scenarios.
π₯88
16:43
AI models like GPT-4o Mini can struggle with complex scenarios, making errors even with slight variations in input.
- Models may overlook critical details in scenarios, leading to incorrect responses.
- Even minor changes in input can significantly impact AI model performance.
- Challenges exist in ensuring AI models understand and respond appropriately to nuanced scenarios.