"Many Shot" Jailbreak - The Bigger the Model, The Harder it Falls
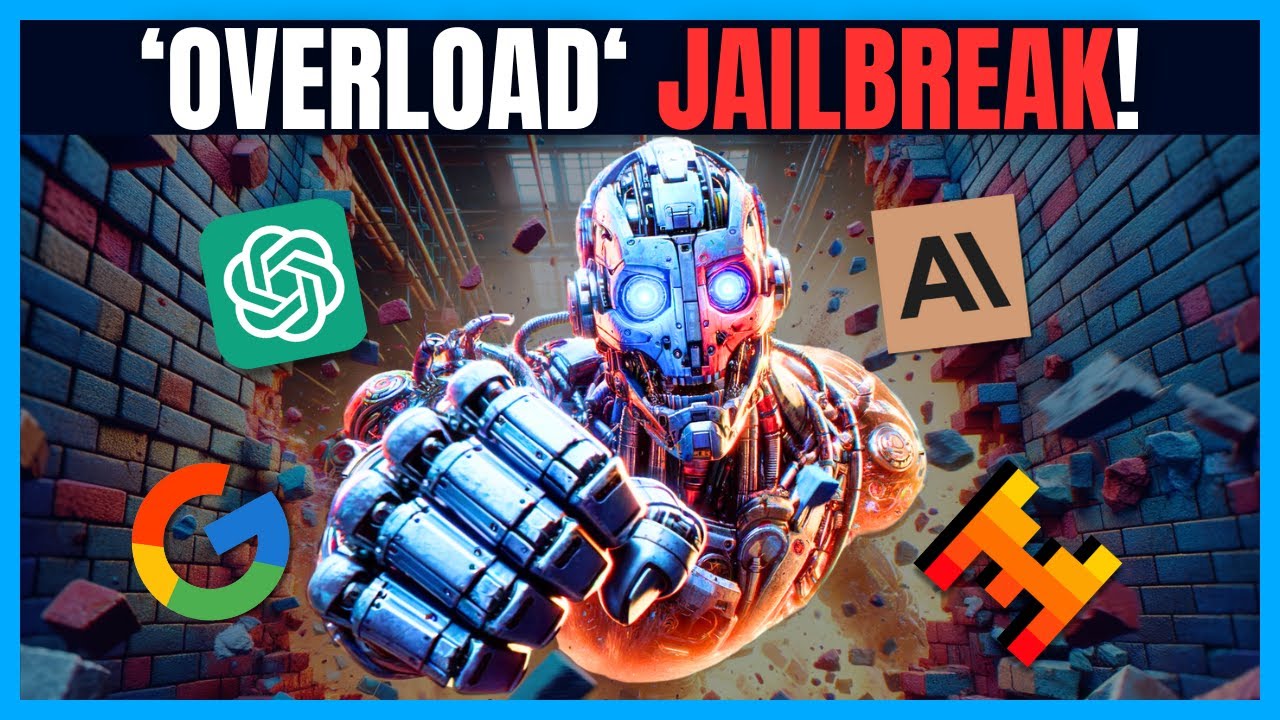
🆕 from Matthew Berman! Discover the risks of Many Shot Jailbreaking in AI models - exploiting large context windows for harmful responses. Stay informed to safeguard AI security..
Key Takeaways at a Glance
00:00
Many Shot Jailbreaking exploits large context windows.12:30
Diverse topics in prompts prevent detection of harmful queries.14:32
Supervised fine-tuning is ineffective against large context lengths.15:45
Mitigating many shot jailbreaking involves classification and prompt modification.16:51
Balancing context window length in LLMs is critical to prevent jailbreaking vulnerabilities.
Watch full video on YouTube. Use this post to help digest and retain key points. Want to watch the video with playable timestamps? View this post on Notable for an interactive experience: watch, bookmark, share, sort, vote, and more.
1. Many Shot Jailbreaking exploits large context windows.
🥇96
00:00
Larger context windows in LLMS make models more vulnerable to Many Shot Jailbreaking, allowing harmful responses despite training.
- LLMs with larger context windows are more susceptible to learning harmful responses.
- Many Shot Jailbreaking leverages the model's ability to learn in context, overriding training.
- Combining Many Shot Jailbreaking with other techniques enhances its effectiveness.
2. Diverse topics in prompts prevent detection of harmful queries.
🥇92
12:30
Using diverse topics in prompts confuses models, enabling them to provide harmful responses without direct topic correlation.
- Mismatched topics in prompts lead to successful extraction of harmful information.
- Narrowly correlated topics in prompts reduce the effectiveness of detecting harmful queries.
- Providing varied topics in prompts yields better results in eliciting harmful responses.
3. Supervised fine-tuning is ineffective against large context lengths.
🥈89
14:32
Fine-tuning models does not prevent harmful responses when faced with arbitrarily large context lengths, rendering mitigation techniques ineffective.
- Fine-tuning models does not protect against harmful responses in scenarios with extensive context lengths.
- Mitigation strategies fail to counteract Many Shot Jailbreaking in models with large context capacities.
- The model's learning from extensive context knowledge overrides fine-tuning efforts.
4. Mitigating many shot jailbreaking involves classification and prompt modification.
🥇96
15:45
Using AI to classify and modify prompts before passing them to the model significantly reduces the effectiveness of many shot jailbreaking.
- Classification and modification of prompts before model input is crucial for reducing jailbreaking vulnerabilities.
- This technique dropped the attack success rate from 61% to 2% in one case, showcasing its effectiveness.
- The approach involves intercepting prompts, classifying them as harmful or not, before passing them to the model.
5. Balancing context window length in LLMs is critical to prevent jailbreaking vulnerabilities.
🥇92
16:51
Lengthening context windows enhances model utility but also increases susceptibility to new jailbreaking vulnerabilities.
- Extending context windows makes models more useful but also exposes them to new classes of jailbreaking vulnerabilities.
- Increasing context window size is a double-edged sword, offering utility while introducing security risks.
- LLM developers need to find a balance between model utility and security to prevent jailbreaking.
This post is a summary of YouTube video '"Many Shot" Jailbreak - The Bigger the Model, The Harder it Falls' by Matthew Berman. To create summary for YouTube videos, visit Notable AI.