Meta's Shocking New Research | Self-Rewarding Language Models
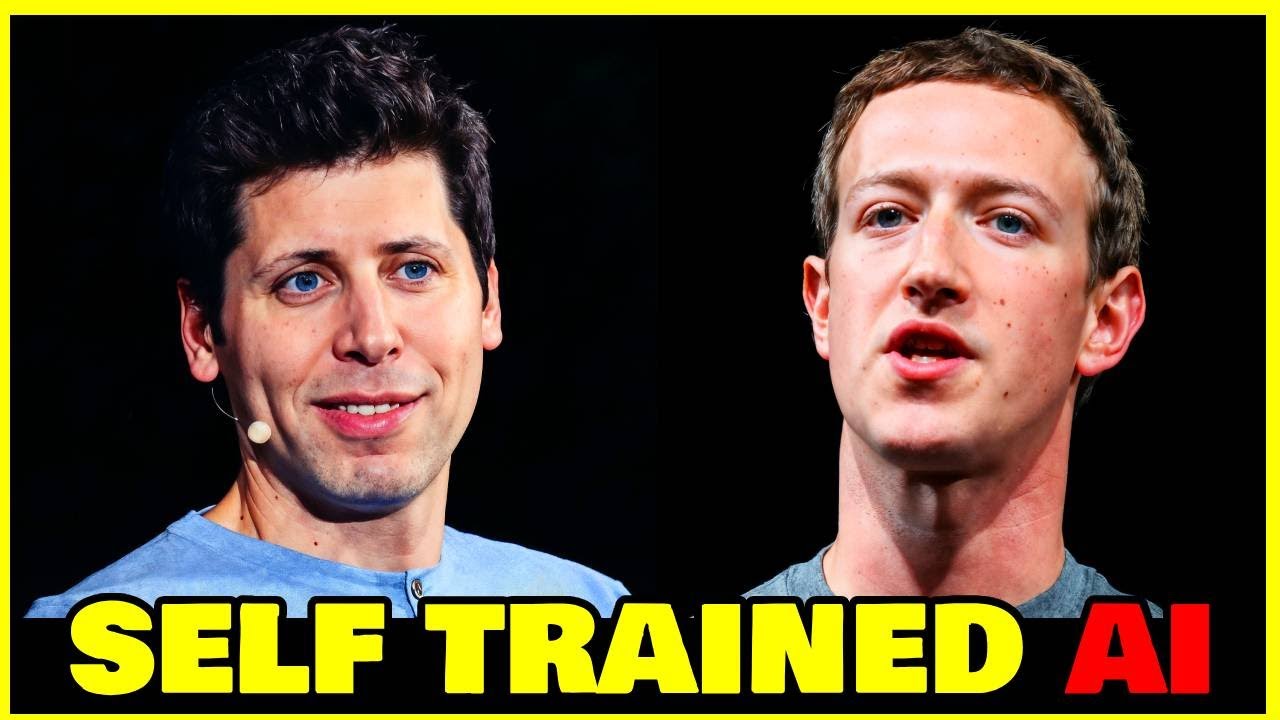
Key Takeaways at a Glance
00:00
AI's self-rewarding ability in training02:23
AI's potential to create next-generation AI03:52
AI's self-rewarding language models06:15
Continuous improvement through self-rewarding AI06:21
AI's ability to judge and improve its own responses10:56
Impact of self-rewarding training methodology on AI evolution14:02
Importance of prompt engineering in AI training.15:49
Continuous improvement of AI's self-rewarding ability.17:25
Potential of open source AI models.
1. AI's self-rewarding ability in training
🥇92
00:00
AI can be trained using self-reflection and self-generated data, similar to how humans train their brains through self-reflection and problem-solving.
- Synthetic data generation could be an important part of future AI training.
- AI's ability to self-reward and improve continuously is a significant development.
2. AI's potential to create next-generation AI
🥈88
02:23
AI version one can create AI version two, leading to potentially superintelligent AI that can unlock mysteries of the universe.
- The concept of AI creating subsequent versions has been discussed by prominent AI researchers.
- This development signifies a shift towards AI self-improvement and exponential growth.
3. AI's self-rewarding language models
🥇95
03:52
Self-rewarding language models show improved instruction following and reward modeling abilities, outperforming existing systems.
- The approach aims to create AI models that can continually improve in both instruction following and reward modeling.
- The self-rewarding process leads to superior learning models compared to those trained solely on human data.
4. Continuous improvement through self-rewarding AI
🥇91
06:15
The self-rewarding process enables AI to continually improve its instruction following and reward modeling abilities.
- The iterative approach results in AI models that continually enhance their learning and performance.
- This method increases the potential for self-improvement of learning models.
5. AI's ability to judge and improve its own responses
🥈89
06:21
AI's self-rewarding process involves generating prompts, responses, and rewards, allowing it to judge and improve its own performance.
- The AI's ability to self-assess and provide feedback to itself leads to continuous improvement.
- The process involves creating and evaluating new instruction following examples to enhance its training set.
6. Impact of self-rewarding training methodology on AI evolution
🥇93
10:56
The self-rewarding training methodology results in AI models that continually outperform previous versions, demonstrating significant evolution.
- The methodology leads to consistent improvement in AI models over iterations, indicating the effectiveness of self-rewarding.
- The approach shows promise in enhancing AI's learning and performance capabilities over time.
7. Importance of prompt engineering in AI training.
🥇92
14:02
Different prompt structures can significantly impact AI model's accuracy and reward modeling ability.
- Prompt engineering can have a massive impact on how well the AI understands and responds to instructions.
- The study showed a huge difference in accuracy scores based on different prompt structures.
8. Continuous improvement of AI's self-rewarding ability.
🥇91
15:49
AI's ability to improve itself through self-rewarding mechanisms seems to have no apparent limits, potentially surpassing human judgment and data quality.
- The study suggests that AI's self-improvement capabilities are continuously evolving and may outperform human judgment and data quality.
- This has implications for the speed, cost, and quality of AI-generated data.
9. Potential of open source AI models.
🥈88
17:25
Open source AI models are expected to become much more powerful and accessible than previously anticipated.
- The accessibility and contribution to open source AI could lead to a significant increase in its power and influence.
- This trend may have far-reaching implications for various industries and the economy.