NEW Mixtral 8x22b Tested - Mistral's New Flagship MoE Open-Source Model
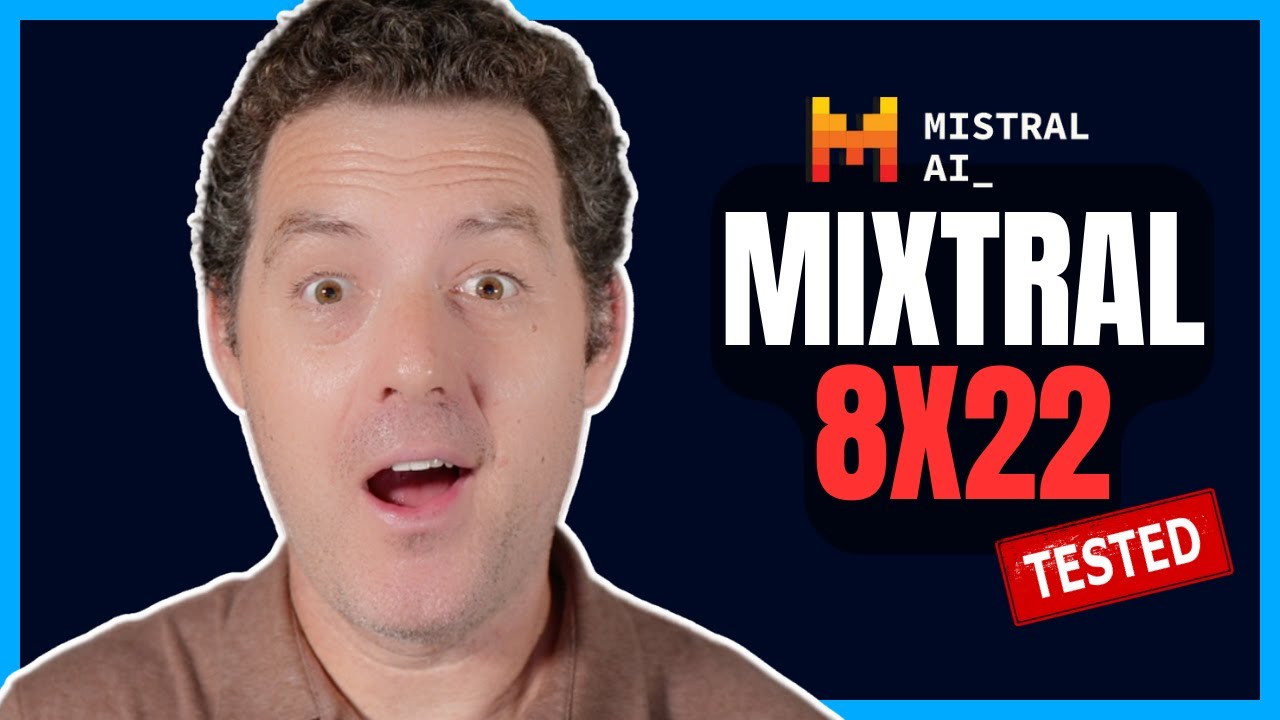
🆕 from Matthew Berman! Discover the impressive capabilities of Mistral's latest Mixtral 8x22b model in handling coding challenges and logic problems. Fine-tuning for specific tasks shows promising results. #AI #ModelPerformance.
Key Takeaways at a Glance
00:00
Mistral's new Mixtral 8x22b model is a massive open-source MoE model.01:05
Fine-tuning plays a crucial role in enhancing model performance.02:13
Testing the Mixtral 8x22b model reveals impressive performance.04:09
Model uncensoring capabilities vary based on fine-tuning.08:25
Model performance varies in complex reasoning tasks.
Watch full video on YouTube. Use this post to help digest and retain key points. Want to watch the video with playable timestamps? View this post on Notable for an interactive experience: watch, bookmark, share, sort, vote, and more.
1. Mistral's new Mixtral 8x22b model is a massive open-source MoE model.
🥇92
00:00
The Mixtral 8x22b model is a significant upgrade from the previous 8.7 billion parameter model, offering improved performance and capabilities.
- The new model is an 8.22 billion parameter model, showcasing Mistral's commitment to enhancing their open-source models.
- Expectations are high for further fine-tuned versions to surpass the performance of the previous model.
2. Fine-tuning plays a crucial role in enhancing model performance.
🥈85
01:05
Fine-tuned versions like Kurasu Mixt 8x22b for chat show the importance of customization for specific applications.
- Fine-tuning models for specific tasks like chat applications can significantly improve their performance.
- Customized versions tailored to specific use cases can lead to better outcomes and user experiences.
3. Testing the Mixtral 8x22b model reveals impressive performance.
🥈88
02:13
The model demonstrates strong capabilities, performing well in tasks like coding challenges and logic problems.
- Successful completion of tasks like writing Python scripts and solving logic puzzles showcases the model's competence.
- The model's ability to handle various challenges indicates its versatility and potential for further improvements.
4. Model uncensoring capabilities vary based on fine-tuning.
🥉79
04:09
The model's responses can range from censored to uncensored based on the level of fine-tuning and specific queries.
- Pushing the model with certain queries can reveal its uncensored responses, highlighting the impact of training data and fine-tuning.
- Fine-tuning for specific tasks may influence the model's behavior towards sensitive or explicit content.
5. Model performance varies in complex reasoning tasks.
🥉76
08:25
The model's accuracy in complex reasoning tasks like physics scenarios and logic puzzles can be inconsistent.
- Challenges like physics scenarios and nuanced logic problems may expose limitations in the model's reasoning abilities.
- In scenarios requiring deep understanding or nuanced responses, the model may struggle to provide accurate answers.
This post is a summary of YouTube video 'NEW Mixtral 8x22b Tested - Mistral's New Flagship MoE Open-Source Model' by Matthew Berman. To create summary for YouTube videos, visit Notable AI.