NVIDIAs new 'Foundation Agent' SHOCKS the Entire Industry! | Dr. Jim Fan, GR00T and Isaac Robotics
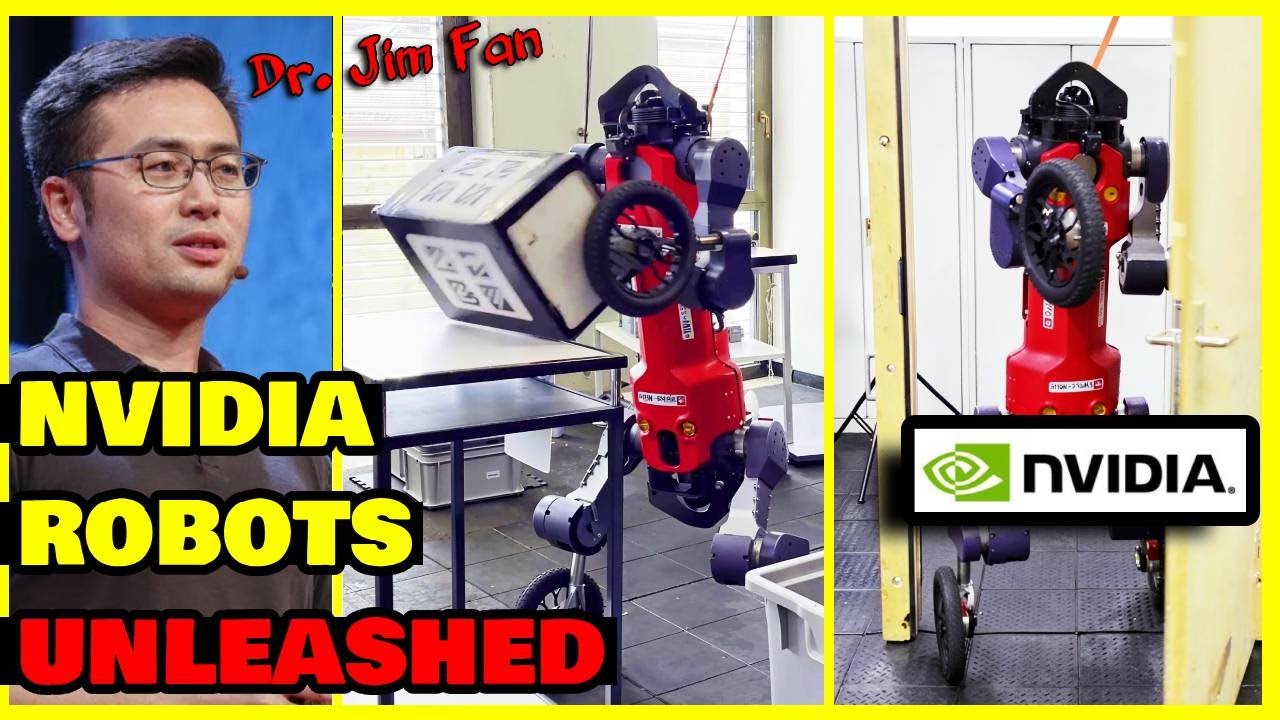
Key Takeaways at a Glance
02:12
Essential features of a versatile AI agent.03:34
Utilizing Minecraft as an open-ended environment for AI development.07:00
Training AI models with language-conditioned rewards.08:25
Advancing AI capabilities through lifelong learning and self-improvement.11:29
Enabling universal control of diverse robot embodiments with metamorph.13:46
Isaac Sim enables rapid skill acquisition through accelerated simulations.15:00
Automating reward function creation streamlines AI training.17:23
Hybrid gradient architecture enhances AI learning efficiency.18:58
Foundation Agent aims to generalize AI capabilities across realities.26:35
Foundation models like Groot are crucial for making humanoid robots useful.27:28
Collaboration with startups and research groups is welcomed for humanoid robotics advancement.29:03
Lowered entry barriers in AI enable students to engage in AI development from a young age.33:43
Sim-to-real challenges pose significant hurdles in robotics advancement.
1. Essential features of a versatile AI agent.
🥇96
02:12
AI agents need to survive, navigate, explore in open-ended worlds, possess vast pre-training knowledge, and perform a multitude of tasks.
- Survival, navigation, and exploration are crucial for open-ended environments.
- Extensive pre-training data is essential for effective agent functioning.
- Multitasking capability is vital for AI agents to handle diverse tasks.
2. Utilizing Minecraft as an open-ended environment for AI development.
🥇93
03:34
Minecraft's lack of specific objectives makes it ideal for training AI agents in a truly open-ended setting, fostering creativity and skill development.
- Minecraft's open-ended nature allows for diverse and creative agent behaviors.
- Leveraging Minecraft's vast player-generated data for AI training is highly beneficial.
- Mind Dojo framework enables the development of general-purpose agents using Minecraft.
3. Training AI models with language-conditioned rewards.
🥈89
07:00
Using language-conditioned rewards like the Mind Clip model enhances AI understanding and performance, enabling agents to align behaviors with textual prompts.
- Mind Clip model associates video actions with corresponding text descriptions.
- Language-conditioned rewards improve agent behavior alignment with desired tasks.
- Reinforcement learning with language feedback enhances AI capabilities.
4. Advancing AI capabilities through lifelong learning and self-improvement.
🥇92
08:25
Implementing lifelong learning strategies allows AI agents like Voyager to continuously explore, learn new skills, and adapt to novel challenges without pre-programmed limitations.
- Voyager's self-reflection mechanism aids in improving skills and decision-making.
- Curriculum-based challenges help agents discover new skills progressively.
- Lifelong learning fosters curiosity and adaptability in AI agents.
5. Enabling universal control of diverse robot embodiments with metamorph.
🥈88
11:29
Metamorph's transformer-based approach allows for universal control of various robot morphologies, demonstrating the potential for cross-embodiment generalization and multibody control.
- Metamorph's transformer model enables control of robots with varied kinematic properties.
- Training a single policy for diverse robot embodiments streamlines control mechanisms.
- Generalization to unseen morphologies showcases the versatility of the approach.
6. Isaac Sim enables rapid skill acquisition through accelerated simulations.
🥇92
13:46
Isaac Sim's accelerated physics simulations allow for quick skill acquisition, like mastering martial arts in days through virtual training equivalent to years.
- Simulations run a thousand times faster than real time.
- Complex worlds with high realism aid in training AI models for various tasks.
- Procedurally generated infinite worlds enhance AI training possibilities.
7. Automating reward function creation streamlines AI training.
🥈89
15:00
Automated reward function generation in ISAC Sim reduces training time from days to minutes, enhancing AI learning efficiency.
- Reinforcement learning optimizes reward functions through trial and error.
- Automated feedback reports guide AI improvement.
- Urea bridges high-level reasoning and low-level motor control in AI training.
8. Hybrid gradient architecture enhances AI learning efficiency.
🥈88
17:23
The hybrid gradient architecture in Urea combines high-level reasoning with low-level motor control, optimizing AI training effectiveness.
- Systematic separation of slow and fast reasoning mimics human cognitive processes.
- Inference at different frequencies aids in efficient AI control.
- Urea automates reward function refinement for improved task performance.
9. Foundation Agent aims to generalize AI capabilities across realities.
🥇94
18:58
The Foundation Agent project seeks to scale AI capabilities massively across various realities, aiming for a single model that can generalize across different tasks.
- Foundation Agent takes embodiment and instruction prompts to output actions.
- Scaling up training on diverse skills and embodiments is a key focus.
- Long-term vision includes autonomous movement for all entities.
10. Foundation models like Groot are crucial for making humanoid robots useful.
🥇92
26:35
Foundation models like Groot play a fundamental role in making humanoid robots more than just a curiosity, enabling them to be truly useful in various tasks.
- Humanoid robots currently lack practical utility and are more of a novelty.
- Deploying foundation models massively can enhance the functionality of humanoid robots.
- Foundation models are essential for shipping compute infrastructure and enabling customization for robots.
11. Collaboration with startups and research groups is welcomed for humanoid robotics advancement.
🥈88
27:28
NVIDIA welcomes collaboration with startups, researchers, and students to enhance humanoid robotics technology and drive innovation.
- Many humanoid companies are startups, making collaboration with them essential.
- Encouraging researchers and students to join in the mission-driven research for humanoid robotics advancement.
12. Lowered entry barriers in AI enable students to engage in AI development from a young age.
🥈85
29:03
AI's accessibility allows students, even in middle school, to engage in AI development by leveraging APIs and open-source resources.
- Students can register for AI accounts and start building agents without significant funding.
- Middle school students can reproduce Voyager using accessible AI tools like Nvidia LM API and open APIs.
13. Sim-to-real challenges pose significant hurdles in robotics advancement.
🥇94
33:43
Transferring research from simulations to real-world applications faces challenges like simulation fidelity, hardware reliability, and software bugs.
- Domain randomization in simulations aids in generalization to real-world scenarios.
- Data collection for robotics, including internet, simulation, and real robot data, presents complex challenges.
- Scaling up AI models for robotics requires addressing action extraction issues for embodied agents.