Nvidias NEW "Foundation AI AGENT" Will Change The WORLD! (Jim Fan)
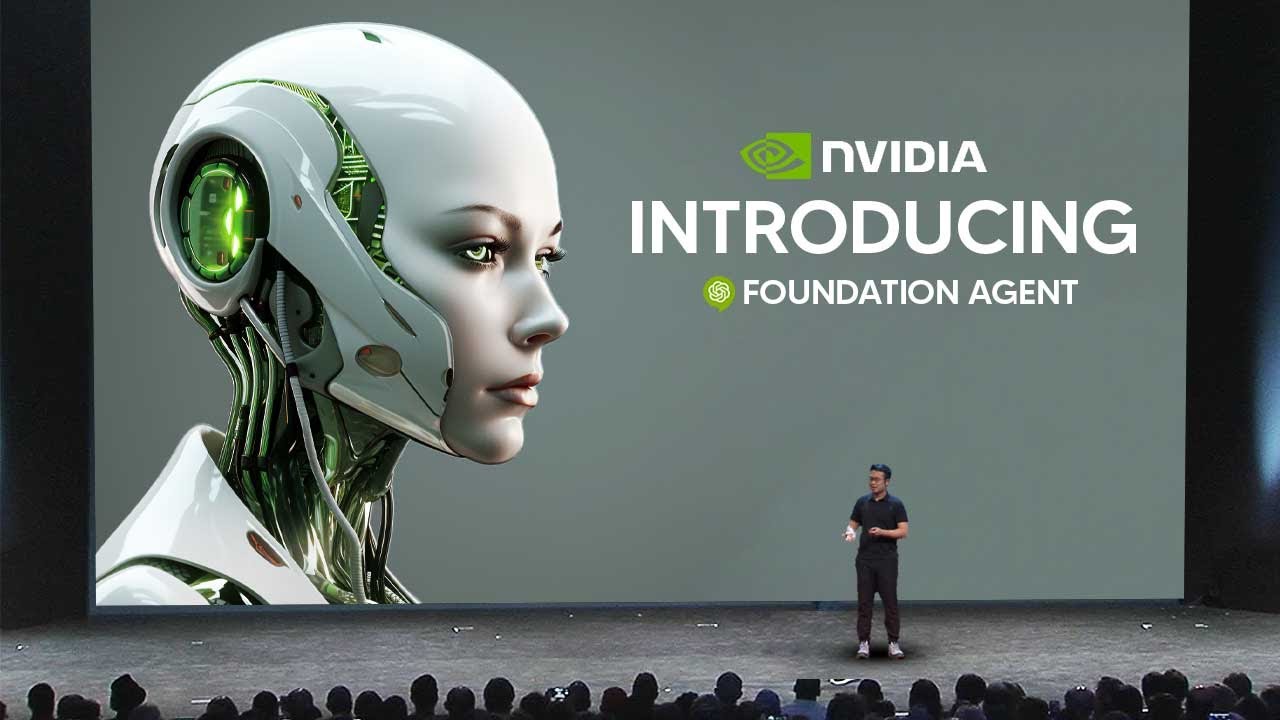
Key Takeaways at a Glance
00:00
Foundation agent's potential to revolutionize AI applications02:48
Voyager's capabilities in Minecraft showcase AI's potential07:55
Challenges and potential of multi-agent interactions09:14
Scaling AI across multiple simulated realities12:28
Utilizing YouTube data for AI skill learning15:04
Training embodied agents using video models21:48
Scalability of simulation for training complex policies23:30
Dual-loop system for training embodied agents25:42
Future scalability and real-world application of training methods27:55
AI can automate the development of robotics.30:11
Challenges in robotics research focus on data collection.
1. Foundation agent's potential to revolutionize AI applications
🥇95
00:00
The Foundation agent, a versatile AI, could seamlessly operate in virtual and physical environments, transforming various industries from video games to humanoid robots.
- It has the potential to master skills across different realities, impacting diverse domains.
- This technology could fundamentally change our lives by permeating everything from video games and metaverse to drones and humanoid robots.
2. Voyager's capabilities in Minecraft showcase AI's potential
🥇92
02:48
Voyager, an AI agent, can play Minecraft professionally for hours without human intervention, demonstrating AI's ability to master complex tasks and skills.
- Voyager's exploration and self-improvement in Minecraft highlight AI's capacity for lifelong learning and autonomous skill development.
- The AI's ability to write code, self-reflect, and improve its skills showcases its advanced capabilities.
3. Challenges and potential of multi-agent interactions
🥈85
07:55
Exploring the potential of multi-agent interactions presents new emerging properties for AI, although current frameworks may not fully support this concept, highlighting the need for future advancements in AI frameworks.
- The idea of multiple agents interacting and developing different goals presents intriguing possibilities for AI development.
- The discussion on multi-agent interactions underscores the ongoing evolution and potential of AI systems.
4. Scaling AI across multiple simulated realities
🥇91
09:14
The future of AI involves scaling AI models across various simulated realities, enabling them to master skills, control different embodiments, and navigate diverse virtual and physical worlds.
- AI's ability to master different simulated realities, including open-ended games and robot simulations, presents a vision for versatile AI applications.
- The concept of the real world being just another simulation to AI guides the design of next-generation embodied AI systems.
5. Utilizing YouTube data for AI skill learning
🥈88
12:28
Nvidia researchers leverage YouTube videos to train AI models, using video-text alignment to reinforce learning through human feedback, demonstrating innovative data utilization for AI skill acquisition.
- The use of YouTube videos as a data source for AI skill learning showcases creative and unconventional approaches to AI training.
- This method enables AI to learn from human feedback without manual data annotation, enhancing its learning capabilities.
6. Training embodied agents using video models
🥇92
15:04
Training embodied agents involves using video models from various sources, including games and real-world tasks, to develop common sense and intuitive physics.
- Videos encode intuitive physics, which is crucial for predicting and understanding real-world scenarios.
- Embodied agents lack common sense and intuitive physics, which can be learned from extensive video training.
- Both videos and simulations are essential for grounding knowledge and skills in embodied agents.
7. Scalability of simulation for training complex policies
🥈88
21:48
Simulation, such as ISAC Sim built on Omniverse, enables scaling up data streams and training complex policies, like pen spinning, at a significantly faster rate than real-world training.
- Simulation allows for parallel computing, simulating thousands of scenarios simultaneously, which is impractical in the real world.
- The scalability of simulation accelerates the training of embodied agents for various tasks and skills.
8. Dual-loop system for training embodied agents
🥈85
23:30
The dual-loop system in training embodied agents involves a language model writing code for the reward function and reinforcement learning to train a network controlling the agent, enabling high-level reasoning and muscle memory-based control.
- The dual-loop system consists of a deliberate, slow reasoning loop and a fast, unconscious muscle memory loop for controlling the agent.
- This approach allows for training agents to perform dexterous tasks and manual manipulation.
9. Future scalability and real-world application of training methods
🥈89
25:42
The future of training methods involves scaling simulation skills and transferring neural network learning from simulation to the real world, potentially enabling a fully LM-trained robot.
- Scaling simulation skills to master various simulated realities can aid in generalizing to the complex and diverse real world.
- Efforts are being made to bridge the gap between simulated training and real-world application for embodied agents.
10. AI can automate the development of robotics.
🥇92
27:55
Using AI, such as Nvidia's Foundation AI Agent, can automate the development of robotics by instructing how to train robots and writing reward functions better than human developers.
- AI like GPT-3 can understand and write reward functions based on physics API documentation.
- This automation could potentially lead to the entire robot stack being programmed by AI iteratively.
11. Challenges in robotics research focus on data collection.
🥈88
30:11
The primary challenge in robotics research lies in data collection, which can be sourced from internet videos or scaled-up simulations.
- Data collection from simulations involves actively collected data by the agent itself.
- The architecture used is not the main pain point; the challenge lies in obtaining sufficient data.