This Curious Robot Should Be Impossible!
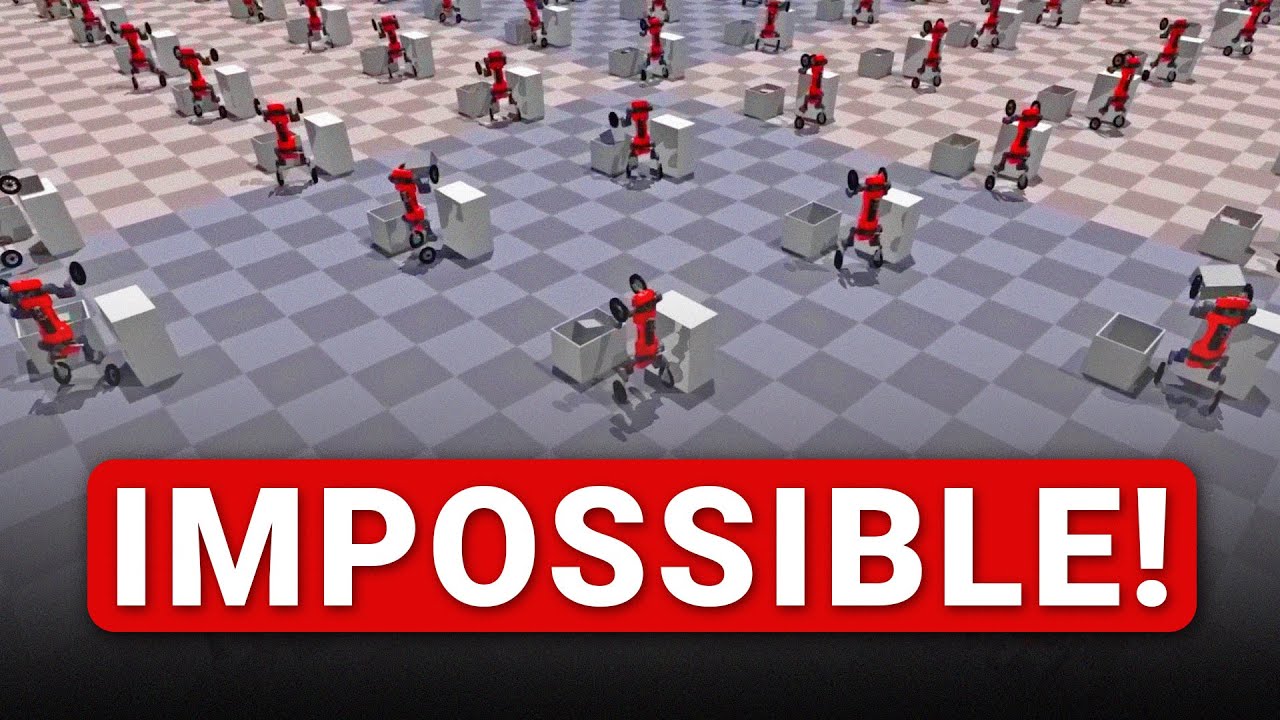
🆕 from Two Minute Papers! Discover how training robots in simulated environments can enable them to perform complex tasks in the real world. #Robotics #AI.
Key Takeaways at a Glance
00:00
Training robots in a simulation environment can enable them to perform complex tasks in the real world.02:11
Curiosity-driven learning can enhance a robot's ability to explore and understand its surroundings.03:47
Training robots in virtual environments can have real-world applications.06:09
Hand-engineering reward functions is a limitation in training AI agents.
Watch full video on YouTube. Use this post to help digest and retain key points. Want to watch the video with playable timestamps? View this post on Notable for an interactive experience: watch, bookmark, share, sort, vote, and more.
1. Training robots in a simulation environment can enable them to perform complex tasks in the real world.
🥇92
00:00
By allowing robots to learn and explore in a simulated video game environment, they can acquire the skills needed to navigate, handle objects, and perform tasks in the real world.
- Simulated training provides a safe and controlled environment for robots to learn without the risk of injury or damage.
- The rewards in the simulation environment are engineered to encourage the robot to learn and explore.
2. Curiosity-driven learning can enhance a robot's ability to explore and understand its surroundings.
🥈88
02:11
By designing reward functions that incentivize the robot to explore and understand the world, it becomes more curious and motivated to learn.
- Curiosity-driven learning can help robots adapt to new tasks and environments.
- DeepMind's agents are an example of how curiosity can drive exploration and learning in robots.
3. Training robots in virtual environments can have real-world applications.
🥈86
03:47
The knowledge gained from training robots in virtual environments can be applied to real-world tasks, such as last mile delivery and self-driving cars.
- Virtual training allows for the creation of challenging environments that can improve the performance of AI agents.
- Virtual training can also accelerate the learning process by allowing robots to train for extended periods of time in simulation.
4. Hand-engineering reward functions is a limitation in training AI agents.
🥈82
06:09
The need to manually design reward functions for different tasks limits the generality of AI agents.
- Writing reward functions for new tasks can be time-consuming and may require domain expertise.
- Finding a way to automatically generate reward functions could improve the flexibility and adaptability of AI agents.
This post is a summary of YouTube video 'This Curious Robot Should Be Impossible!' by Two Minute Papers. To create summary for YouTube videos, visit Notable AI.