Qwen2 72b BEATS LLaMA3 70b - Fully Tested (0.5b not good)
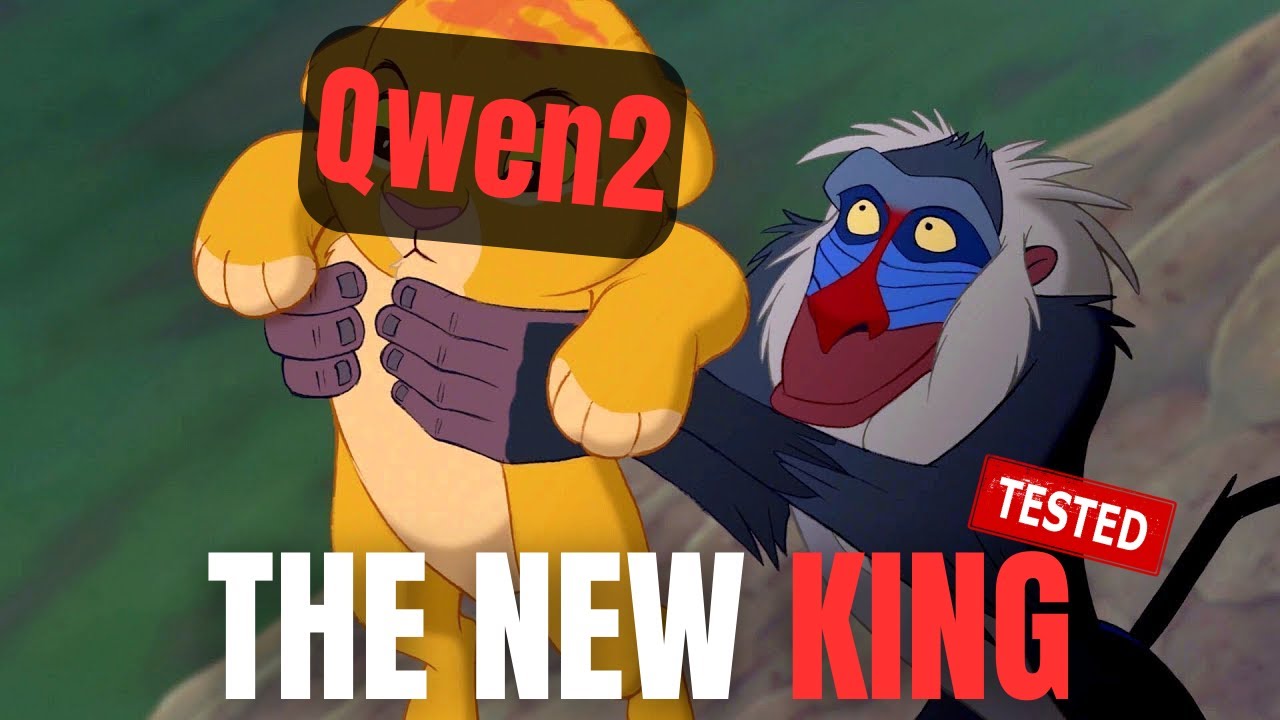
Key Takeaways at a Glance
00:00
Qwen2 72b outperforms LLaMA3 70b in various evaluations.02:12
Testing different model variations reveals performance discrepancies.03:48
Challenges arise in model responses to complex prompts.08:54
Model size impacts response accuracy and task completion.11:14
Evaluation of model performance reveals strengths and weaknesses.13:04
Understanding the concept of presence in a scenario is crucial.13:44
Logical reasoning in problem-solving is essential.
1. Qwen2 72b outperforms LLaMA3 70b in various evaluations.
🥇92
00:00
Qwen2 72b surpasses LLaMA3 70b in multiple evaluations, demonstrating superior performance across different metrics.
- Qwen2 72b excels in evaluations against LLaMA3 70b, Mixel 8 22b, and Quen 1.5 110b models.
- Performance comparison shows Qwen2 72b's superiority in code and math tasks over LLaMA3 70b.
- Extended context support up to 128k tokens enhances performance for 7B and 72b versions.
2. Testing different model variations reveals performance discrepancies.
🥈88
02:12
Evaluating 0.5b and 72b versions of Qwen2 showcases varying performance levels and capabilities.
- Testing both 0.5b and 72b versions provides insights into quality and speed trade-offs.
- Local testing using LM Studio and Hugging Face Spaces offers practical evaluation of model variations.
- Performance discrepancies between smaller and larger models highlight the impact of parameter size.
3. Challenges arise in model responses to complex prompts.
🥈85
03:48
Smaller models struggle with complex tasks like writing code, indicating limitations in handling intricate instructions.
- Small models face difficulties in tasks like writing the game Snake in Python, requiring manual intervention.
- Larger models exhibit better performance in handling complex prompts and generating accurate responses.
- Model limitations become evident in scenarios requiring nuanced understanding and detailed instructions.
4. Model size impacts response accuracy and task completion.
🥈87
08:54
Larger models demonstrate improved accuracy and task completion compared to smaller counterparts.
- Increased model size enhances the ability to handle diverse tasks and generate more accurate responses.
- Smaller models show limitations in processing complex prompts and may require manual corrections.
- Model size plays a significant role in determining the quality and reliability of AI-generated outputs.
5. Evaluation of model performance reveals strengths and weaknesses.
🥈89
11:14
Assessing model responses to diverse tasks highlights strengths and weaknesses in AI capabilities.
- Testing models on logic and reasoning questions exposes their ability to handle different types of queries.
- Performance discrepancies between models underscore the importance of selecting the right model for specific tasks.
- Understanding model limitations aids in optimizing AI usage for desired outcomes.
6. Understanding the concept of presence in a scenario is crucial.
🥇92
13:04
Even if a person is incapacitated, their physical presence in a room counts towards the total count, impacting the scenario's outcome.
- Presence of individuals, even if incapacitated, affects the total count in a scenario.
- Physical presence in a room is considered regardless of the individual's state.
- The number of individuals in a scenario includes those physically present, even if inactive.
7. Logical reasoning in problem-solving is essential.
🥈88
13:44
Applying logical reasoning step by step aids in solving complex problems effectively and arriving at accurate conclusions.
- Logical reasoning involves analyzing each step of a problem-solving process.
- Step-by-step reasoning helps in deducing correct answers in challenging situations.
- Effective problem-solving requires a logical approach to reach accurate solutions.