WHISTLEBLOWER Reveals Complete AGI TIMELINE, 2024 - 2027 (Q*, QSTAR)
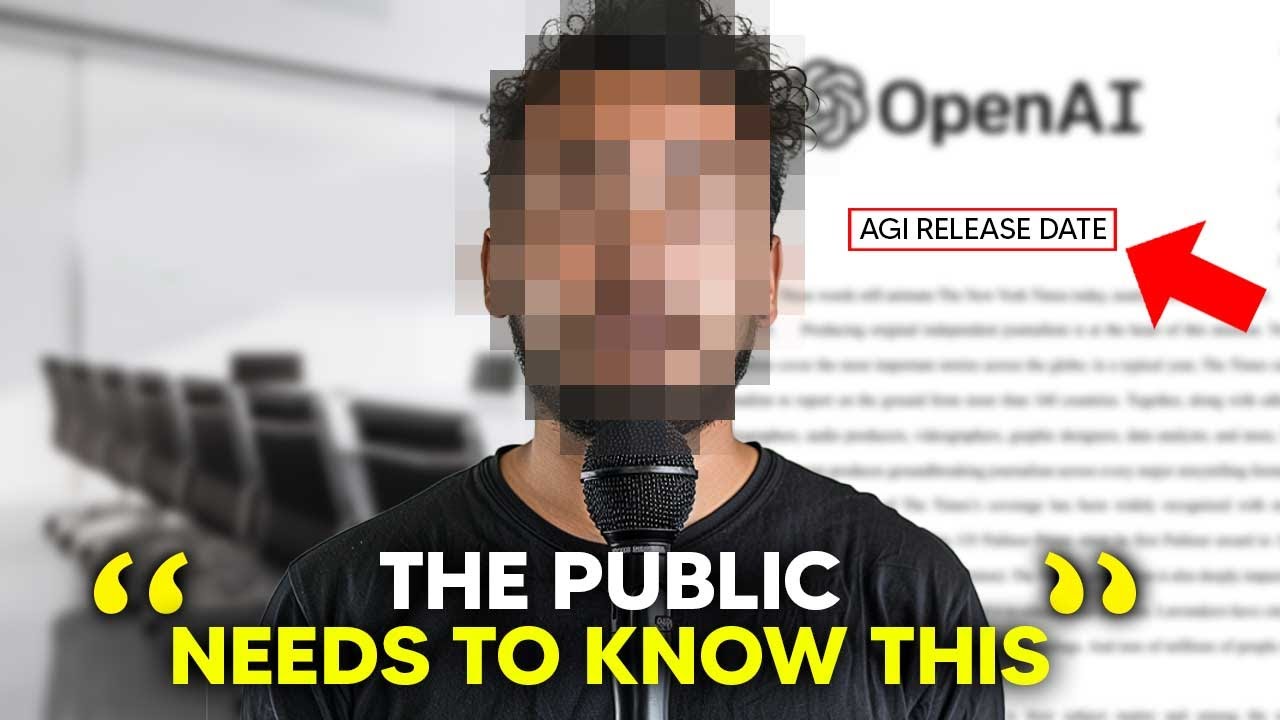
Key Takeaways at a Glance
01:13
OpenAI's plan to create AGI by 2027 involves training multimodal models.01:45
Renaming of GPT models indicates shifts in OpenAI's AI development.06:05
Levels of AGI complexity indicate varying degrees of human-like capabilities.10:00
Parameter count influences AI performance, showing a relationship to task performance.11:31
AGI performance correlates with brain size and parameter count.12:35
Early leaks and speculations hint at the development of GPT models with massive parameter counts.21:07
GPT models are evolving towards multimodal capabilities.23:01
AI training on vast data sources raises AGI possibilities.26:02
Debate surrounds AI's understanding of the physical world.27:36
AGI development timeline reveals ambitious goals.29:10
Concerns arise over rapid AI advancement.32:23
Importance of data quality for AGI development.33:11
Significance of scaling laws in AI model training.41:31
Predicting future AI capabilities through less compute-intensive systems.41:43
Iterative deployment for societal adaptation to AI advancements.42:57
AGI development requires massive computational resources.43:45
AGI progress hinges on predicting capabilities and scale.
1. OpenAI's plan to create AGI by 2027 involves training multimodal models.
🥇92
01:13
OpenAI initiated training a 125 trillion parameter multimodal model in August 2022, with the first stage being rakus or qstar, completed by December 2023.
- The launch was canceled due to high inference costs.
- The document contains verifiable information aligning with known developments.
- Speculation surrounds the delayed plans for achieving human-level AGI by 2027.
2. Renaming of GPT models indicates shifts in OpenAI's AI development.
🥈88
01:45
The original GPT-5 planned for 2025 was renamed GBT-5, with GPT-6 renamed GPT-7, which was put on hold due to a lawsuit by Elon Musk.
- The lawsuit by Elon Musk caused delays in OpenAI's AI development timeline.
- The document hints at a potential progression towards achieving AGI by 2027.
3. Levels of AGI complexity indicate varying degrees of human-like capabilities.
🥇94
06:05
AGI encompasses different levels from emerging AGI to artificial superintelligence, each reflecting increasing human-like abilities.
- AGI levels range from emerging AGI to virtuoso AGI and artificial superintelligence.
- Synapse count correlates with intelligence levels in biological and AI systems.
4. Parameter count influences AI performance, showing a relationship to task performance.
🥈89
10:00
Increasing parameter count in neural network models enhances performance on language-related tasks, with diminishing returns at higher counts.
- Performance on tasks like translation and question-answering improves with higher parameter counts.
- The relationship between parameter count and task performance follows a pattern of diminishing returns.
5. AGI performance correlates with brain size and parameter count.
🥇92
11:31
AGI performance aligns with human brain size and parameter count, with 100 trillion parameters being a crucial threshold for optimal performance.
- Illustrated extrapolations suggest AGI performance matching human levels when brain size aligns with parameter count.
- Engineering techniques can bridge suboptimal performance gaps in AI models with slightly fewer parameters.
6. Early leaks and speculations hint at the development of GPT models with massive parameter counts.
🥈88
12:35
Leaked information suggests the development of GPT models with 100 trillion parameters, indicating significant advancements in AI technology.
- Speculations from various sources point towards the creation of GPT models with unprecedented parameter counts.
- Rumors and leaks indicate plans for models with massive parameter sizes, potentially revolutionizing AI capabilities.
7. GPT models are evolving towards multimodal capabilities.
🥈87
21:07
GPT models are progressing towards multimodal functionalities, including processing videos and audio data, expanding their capabilities beyond text and images.
- The evolution of GPT models includes the ability to process diverse data types like videos and audio for enhanced understanding.
- Multimodal models offer new possibilities, such as understanding natural language and providing cross-language responses.
8. AI training on vast data sources raises AGI possibilities.
🥈85
23:01
Training AI models on extensive internet data could lead to remarkable advancements in robotics.
- Models like GPT-4 and potential future versions could achieve astonishing robotics performance.
- The idea of training models on trillion-parameter scales hints at significant AI progress.
- AI's ability to generate multiple angles of scenes with accurate physics showcases its potential.
9. Debate surrounds AI's understanding of the physical world.
🥈88
26:02
Discussions question whether AI systems possess common sense reasoning and a world model.
- Some argue AI systems go beyond pattern recognition to understand the physical world.
- AI's ability to generate images with accurate physics raises questions about its comprehension.
- The debate centers on whether AI truly grasps the world's interactions and reasoning.
10. AGI development timeline reveals ambitious goals.
🥇95
27:36
OpenAI planned to build a human brain-sized model by 2024, aiming for AGI with 100 trillion parameters.
- Microsoft's $1 billion investment aimed to achieve AGI within 5 years.
- The plan involved training an AI model on images, text, and other data sources.
- The goal was to run a humanized brain model with 100 trillion parameters.
11. Concerns arise over rapid AI advancement.
🥇92
29:10
AI leaders express caution as AI approaches superintelligence faster than anticipated.
- Greg Brockman warned about the dangers of AI advancing towards superintelligence.
- Jeffrey Hinton left Google to discuss the risks of AI surpassing human intelligence.
- The Future of Life Institute urged a 6-month pause in training systems more powerful than GPT-4.
12. Importance of data quality for AGI development.
🥇92
32:23
High-quality data is crucial for AGI development, with vast amounts needed to train models effectively and achieve human-level performance.
- Training on vast amounts of high-quality data is essential for AGI success.
- Data quality directly impacts the performance and capabilities of AI models.
- Achieving human-level performance requires extensive training on quality data.
13. Significance of scaling laws in AI model training.
🥈89
33:11
Understanding and applying scaling laws like the chinchilla model can significantly enhance AI model performance and capabilities.
- Chinchilla scaling laws demonstrate the impact of training models on vast amounts of data.
- Scaling laws help optimize AI model training for improved performance.
- Applying scaling laws can lead to surpassing human-level performance in AI models.
14. Predicting future AI capabilities through less compute-intensive systems.
🥈85
41:31
Training AI models on less compute-intensive systems can help predict the capabilities of future, more advanced models.
- Training on less compute-intensive systems provides insights into future AI capabilities.
- Predicting future AI performance aids in planning for advancements and developments.
- Understanding current AI capabilities guides the prediction of future model performance.
15. Iterative deployment for societal adaptation to AI advancements.
🥈87
41:43
OpenAI plans iterative releases of AI models to allow society time to adapt to evolving AI capabilities and understand the technology.
- Iterative deployment strategy aims to manage societal acceptance of AI advancements.
- Releasing AI capabilities gradually helps society reassess timelines and expectations.
- Predictable scaling aids in accurately forecasting AI advancements for societal readiness.
16. AGI development requires massive computational resources.
🥇92
42:57
Despite reduced compute costs, AGI training demands significant computational power due to compute overhang and scale requirements.
- Compute-intensive nature of AGI training persists despite cost reductions.
- Compute overhang poses a challenge due to insufficient available computational resources.
- Scale is crucial for AGI development, necessitating substantial computational capabilities.
17. AGI progress hinges on predicting capabilities and scale.
🥈89
43:45
Predicting AGI capabilities and scaling up parameter count are key factors in advancing towards AGI.
- Understanding AGI's predictive capabilities is crucial for development.
- Scaling parameter count to human brain size is a significant goal for AGI progress.
- Balancing scale with computational resources is essential for AGI advancement.